Researchers use a variety of techniques to predict the scientific threats and opportunities that might emerge in the future. Bonnie Wintle of the University of Melbourne, a co-author on two 'horizon scanning' studies published in eLife, discusses the challenges involved in predicting future trends in science.
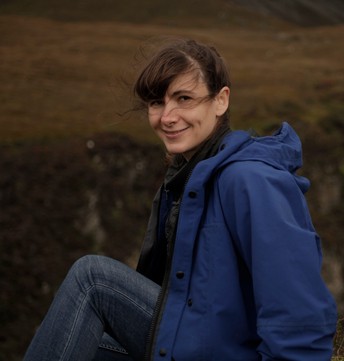
Bonnie Wintle. Image credit: Ben Saunders
What are the main methods used to make predictions about the future in science?
Many forms of 'foresight' – including horizon scanning – involve bringing together groups of people with experience and expertise in technology and innovation to monitor current trends and think about the future. These experts sometimes organise their thinking around a STEEP Framework – that is, what are the social, technological, economic, environmental and political factors that might promote or inhibit different innovations?
There are also computational approaches that analyse massive volumes of papers, patents and conference proceedings in order to identify emerging trends. Various types of network analysis can also be used to explore collaborations between scientists and/or to look for the emergence of new trends. However, I think progress in some of these more computational methods is slow, and people are still pretty indispensable when it comes to anticipating the future.
Who carries out such exercises?
Some exercises take a deep dive into a big, broad trend that cuts across different parts of society, such as the rise of big data or the Internet of Things. These big reports tend to be produced by governments, and are designed to support strategic planning across different government departments: for example, they might direct attention towards particular risks that need to be managed, or towards areas which the departments should be investing in.
Other exercises are used to explore trends in narrower fields. For example, in biosecurity it can be used to look for the emergence of new viruses; in the healthcare sector, it is primarily used to look out for innovative medicines and interventions to guide healthcare planning, and can also help connect biomedical scientists with potential collaborators and funders. Defence and Intelligence agencies are similarly interested in horizon scanning to help give them a strategic advantage on anything from medical technology to new materials for equipment.
What does a typical horizon scanning exercise involve?
The exercises described in our eLife papers both looked at emerging issues in biological engineering. We used a Delphi style approach that brings together a large group of experts (broadly defined) to identify a large pool of potentially emerging and important issues, to discuss and debate them, and then narrow in on a final list of top issues.
Specifically, we ask around 30 contributors to research and identify a selection of issues individually (either identified from their own work or experience, or by attending conferences, consulting other people in their networks, searching online for news articles, using social media, etc). The long list of around 100 candidate issues, including short summaries, are then compiled by the facilitator, circulated to the whole group, and privately scored by each group member in terms of 'horizon scan suitability', that is, based on a combination of novelty, future impact, and likelihood of emerging. From this, we get a prioritised short list, usually of around 30 issues. Often some of the borderline issues are rescued, particularly if there was a lot of variation in the scores.
At this point, we assign a handful of the issues to different group members to research further (in their own time), and then come together in a workshop to discuss each issue in turn. What makes it a suitable or unsuitable horizon scanning issue? What are the possible barriers to this issue emerging in the future? Does anyone have any insight into this issue that we might not have considered? Then we ask everyone to rescore these issues in light of this discussion to produce a final list, which usually contains 15–20 issues. For each of these issues we then discuss the implications, including the potential threats it may pose, opportunities for acting on the issue, and open questions – and write up the results in a paper.
When performing horizon scanning exercises that rely on the opinions and expert knowledge of people, what are ways to reduce subjectivity?
This whole process is done in a structured way that aims to minimise individual and group biases during the exercise. For example, we use anonymity and set ground rules for discussion, and ask people to give their scores individually rather than forcing them to agree as a group. This helps us avoid biases like 'halo effects', where group members might just defer to the most senior, dominant or charismatic person in the room. Also, we try to capture a diversity of views, so different perspectives are balanced out.
Our eLife exercises were based on the same approach taken by Bill Sutherland and colleagues at Cambridge University, who run annual horizon scans to identify emerging issues with the potential to impact global conservation.
Have you revisited these two exercises? If yes, how successful were they? What did they get right, and what did they miss or get wrong?
As part of our "Bioengineering horizon scan 2020", which was led by Luke Kemp, we did revisit some of the issues identified in our 2017 paper on emerging issues in biological engineering, and there had been some notable developments. We saw a significant advance in human germline genome editing in 2019 with the birth of two girls with CRISPR/Cas9-edited genomes. Also, a breakthrough in ‘enhanced photosynthesis' technology did indeed lead to increased agricultural productivity as predicted, a technology that is now being used in crops. Another topic we identified in 2017, the use of 'platform technologies to address emerging disease pandemics' became particularly significant during the COVID-19 pandemic, and many of the vaccine candidates for COVID-19 were developed from platforms for non-coronavirus candidates, such as influenza, SARS and Ebola.
But I haven't come across many 'looking back' reviews of horizon scan issues. There was a review of the first global conservation scan that I mentioned earlier, 10 years on, which revealed that most of the 15 topics identified in the 2010 scan, including microplastic pollution, synthetic meat and environmental applications of mobile-sensing technology (collecting environmental data using mobile phones), had emerged, that is, they had become more prominent and impactful issues; three had not emerged. So we have seen some effort to explore how previously identified horizon-scan topics have unfolded, but I haven't seen much reflection on issues that may have been missed, that is, issues that were not identified, but later emerged as important. That would be really useful, I think.
What are some examples of the impact of horizon scanning on policy or decision-making?
My background and experience are mainly in environmental science, so these are the examples I'm most familiar with. A good example, I think, is the Antarctic and Southern Oceans Science Scan and Roadmap Challenges Exercise, which was a hybrid horizon scanning and road map activity. The scan itself aimed to identify important scientific questions relevant to the southern Polar Regions that should be prioritised in future research. This is a bit different to identifying novel and emerging issues that are on the horizon, like technologies, threats or opportunities, but it is still a form of horizon scanning. The first exercise was followed up by the Antarctic Roadmap Challenges Project, which translated the highest priority research questions into actionable requirements, e.g. the access, logistics, infrastructure and international cooperation needed to address the questions. These reports have been cited by, among others, the National Science Foundation in the US. The global conservation scans have also been used by the Natural Environment Research Council in the UK. However, assessing the impact of horizon scans on policy can be difficult because many decisions are influenced by a range of inputs, and some decisions are not clearly documented.
Before the start of the 2020 pandemic, what were the views regarding the likelihood of a global pandemic?
I used to work at the Centre for the Study of Existential Risk, where we had some bleak conversations about catastrophic risks to humanity. A global pandemic was always high on that list, and was considered by many to be the most probable. Pandemics are not uncommon, and experts in disease and viruses have been warning us about them for years. The Spanish flu wasn't so long ago, and obviously the pathways for exposure, establishment and spread are far greater these days.
In your opinion, how good are we at predicting trends within science?
Unfortunately, my answer to this is not very satisfying: it depends. How far into the future are we predicting? How are we measuring the success (or otherwise) of our predictions? How established is the issue already? How specific is the prediction? I won't be winning any prizes for predicting that AI is going to be big (or bigger) in the future, but if I identify something relatively unknown at the time, or a particular application, and it turns out to be transformative, that's a more useful prediction. How often does that happen? Unfortunately, I really don't know, but it would be really interesting if someone did a large-scale systematic study on that question.
Do you think that humans or computers are better at predicting the future?
My feeling is that people are currently better at predicting trends and synthesising different sources of information than computers are, as we've seen with the recent successes of human groups of superforecasters. One such group outperformed all others in a large forecasting tournament funded by the US government, designed to seek the most promising methods to improve forecasting in the intelligence community.
To develop superforecasters, elite teams of people, who already have a good track record for forecasting, are trained in areas like probability and scenarios, and given strategies to help overcome well-known human biases and frailties in judgement, like overconfidence. Similar to the Delphi-style approach to horizon scanning I outlined earlier, these superforecasters work together in small groups to exchange rationales, news articles and other information, and debate differences of opinion.
Using humans to predict the future is time consuming and expensive compared to using computers, but problems faced by some computational methods are proving difficult to overcome: for example, sentiment analysis offers a potentially useful tool for computers to identify new innovations that people are excited about. But analysing sentiment is tricky, because language is not entirely formulaic. Even within the same language, people use irony, sarcasm, idioms and tone in different ways. Generally speaking, characterising what the longer-term future might look like is hard – although I think we're getting better at it!
What are the limitations of horizon scanning approaches?
A challenge in how we approach horizon scanning is that the experts selecting the issues are prioritising them based on a mix of novelty, future impact, and likelihood of emerging, which are subjective qualities, and difficult to measure or estimate. The results will also depend on what people value, of course. It's difficult to get around this, which really highlights how important it is to capture different perspectives and values in the participants.
Additionally, there are trade-offs in what makes a good horizon scanning issue: we want to avoid identifying issues that are already well known, but that still have a reasonable chance of emerging. Also, we want to see issues framed at a similar level of granularity; for example, it's hard to compare something really specific, like using AI to create logos for businesses in Japan, with something really big and broad that is almost certain to continue to emerge in some form and have huge consequences, like machine learning. In our horizon scans, we do give contributors some guidance on the level of granularity that we seek (with examples), but of course we're still comparing apples with oranges to some extent.
Of course, there is always a risk with any research seeking to impact policy or decisions that useful information is gathered but not followed up. Often this is because decisions are driven by many factors, including non-scientific ones, like budgets, politics or the culture of the organisation at the time. Another limitation is that many horizon scanning outputs aren't clearly 'actionable': they can sometimes be a bit fuzzy and it's not always clear what a single organization or government can do about them, or who is responsible for integrating outputs into policy-making. To stand a better chance of being adopted, horizon scans can be embedded into a broader strategic foresight framework or program. That is, horizon scanning can be used as a first step to identifying emerging and novel threats and opportunities, and then be followed up with other tools like backcasting to work out an actual, concrete pathway to some desired future point. Backcasting basically gives you a roadmap of the steps you need to take to be in a desired place down the track (or, as is often the case, if you seek to avoid the 'undesirable' future that you'd likely face if you continued on your current trajectory). For example, if a company wishes to cut carbon emissions by 50% in ten years, what are the policies and changes that would need to be adopted in say, 2 years, 5 years and 8 years for this to happen? And who is responsible for each step, what are the likely obstacles you'll encounter, and how might you get around them?
Do you think that exercises such as horizon scans have the potential to affect their own results?
I think the answer really depends on the size of the scan and the nature of the issue. Yes, perhaps if a really high-profile scan identifies a previously unknown scientific innovation, maybe more people will start researching it or a government agency or funder might take a look at it. But of course, if we're talking about identifying threats, you hope that the scan has the potential to affect future outcomes. We hope that a new or emerging threat like microplastics in honey will be addressed. It's a tough question!
In the next few years, do you think that advances in computational methods will have an impact on horizon scanning and related approaches?
Good question. I'm no expert in computational methods, but advances in big data analytics and natural language processing will almost certainly improve our ability to spot weak signals. Automated parsing and processing of large volumes of information will undoubtedly speed up data gathering, but may be limited to information that is already available online, or at least, machine-readable. There are also barriers to accessing information that is not publicly available, e.g. exploratory work that is not yet published, and this can be the most cutting-edge and useful material for horizon scanning. I think there will always be value in accessing what's in a person's head… new ideas and seeds for innovation that haven't yet been written down and put out into the world.
Bonnie Wintle was interviewed by Helena Pérez Valle, Associate Features Editor, eLife.