Assessing the balance between excitation and inhibition in chronic pain through the aperiodic component of EEG
Figures
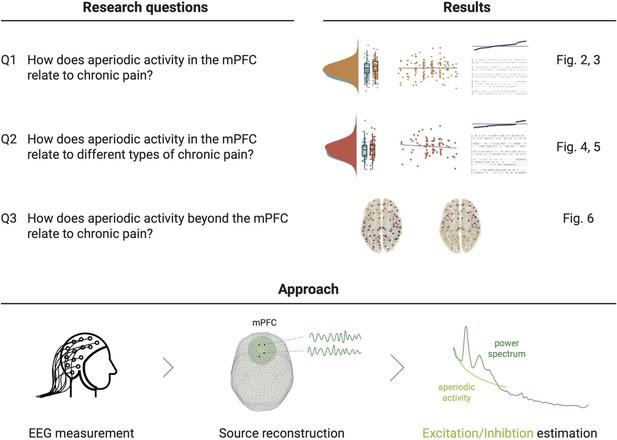
Study outline.
The upper panel states the research questions and sketches their accompanying results from the two pre-registered analyses and their complementing multiverse analyses. The lower panel illustrates the pre-registered approach to estimate E/I non-invasively with EEG. We reconstructed the time series at one hundred spatial points in source space and summarized the medial prefrontal cortex (mPFC) activity (Q1 and Q2) by averaging power spectra in this region. We then estimated the aperiodic activity of the average power spectrum. The aperiodic exponent (the slope of the aperiodic activity in log-log space) is the proposed proxy for E/I, with high aperiodic exponents indicating low E/I ratio. Figure 1—figure supplement 1 shows the grand averaged power spectra in the mPFC.
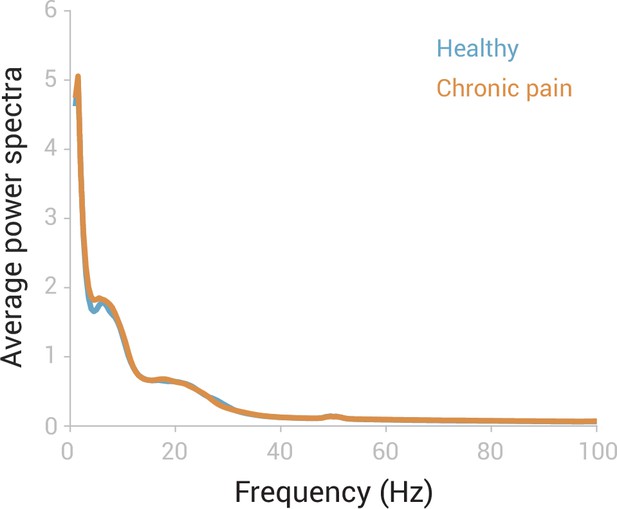
Grand averaged power spectra in the mPFC.
Power spectra derived from source-reconstructed signals were averaged for each group of participants at the four source locations of the atlas comprising the medial prefrontal cortex.
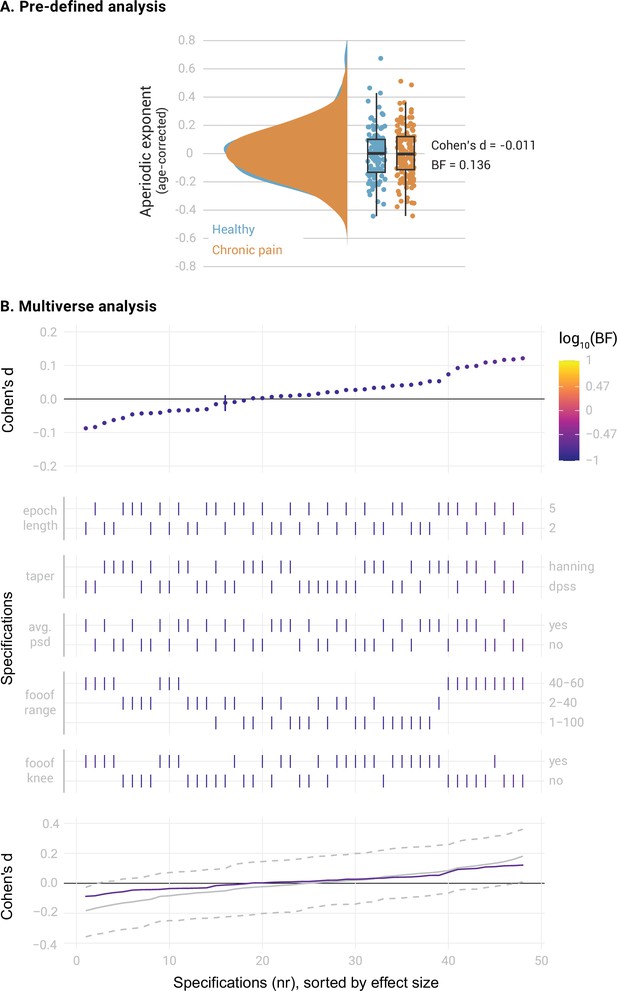
Comparison of the aperiodic exponent in the medial prefrontal cortex (mPFC) between people with chronic pain (N=149) and healthy participants (N=115).
(A) Pre-defined analysis. Raincloud plots include individual data points, boxplots and probability density functions. The box of the boxplots ranges from the first to the third quartile, with a thicker line representing the sample median. Whiskers extend the first and third quartile to 1.5 times the interquartile range. Age was regressed out from the aperiodic exponents of each group. Bayes Factor (BF) was derived from a two-sided independent samples Bayesian t-test comparing the two groups. (B) Multiverse analysis. The upper panel visualizes the specification curve, which indicates the effect size (y axis) and evidence (color coded) for each specification (x axis). Specifications are ordered by effect size. A vertical bar indicates the specification of the pre-defined analysis. The color scale’s upper and lower limits indicate strong evidence for and against a difference between groups. The middle panel further describes the parameters of each specification, with vertical bars indicating the parameters selected in each methodological decision (Table 1). The lower panel visualizes inference on the specification curve. In purple, the original specification curve is depicted. In grey, the null distribution of specification curves derived from 500 randomized curves is represented by the median curve (solid grey line) and 2.5th and 97.5th percentile curves (dashed lines). Intuitively, if the original curve lies between the 2.5th and 97.5th percentile curves, it is not significantly different from the null distribution of specification curves.
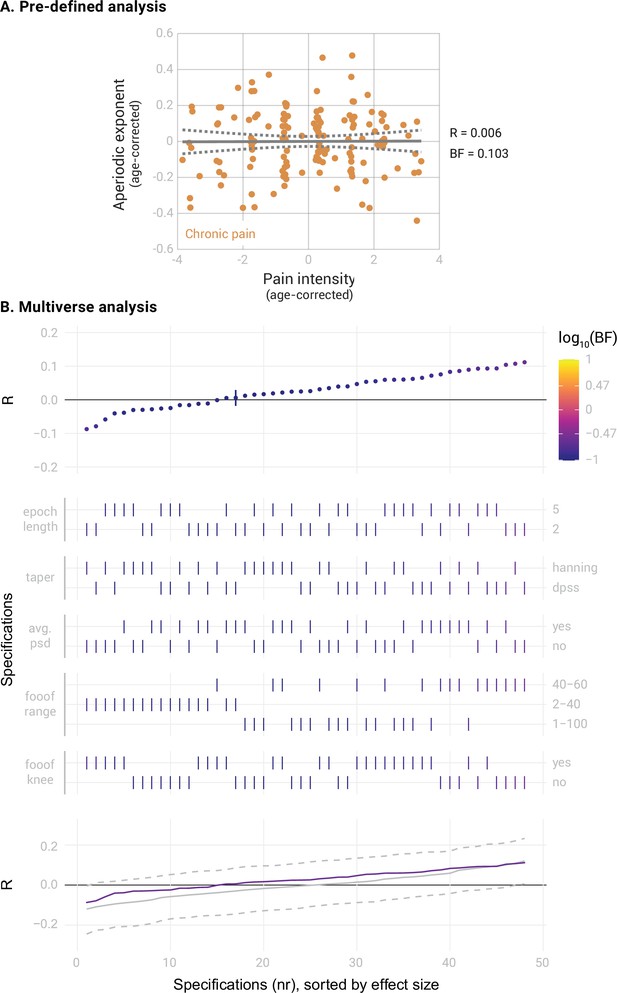
Correlation between the aperiodic exponent in the medial prefrontal cortex (mPFC) and average pain intensity in people with chronic pain (N=149).
(A) Pre-defined analysis. The scatter plot represents the aperiodic exponent and average pain intensity ratings. Age was regressed out from both variables to perform a partial correlation. Grey lines indicate the linear regression slope (solid line) and the 95% confidence interval (dashed lines). Bayes Factor (BF) was obtained from a Bayesian linear correlation performed on the residuals. (B) Multiverse analysis including the specification curve panel with Pearson’s correlation coefficients (R) as effect size, the specifications panel, and the inference panel as in Figure 2.
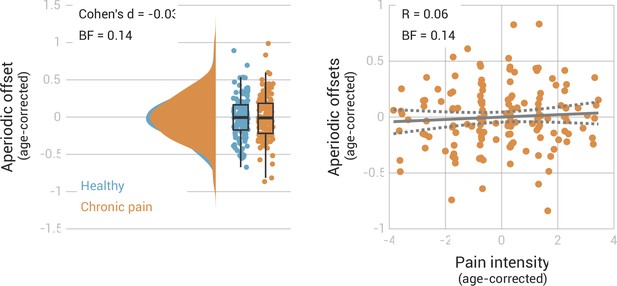
Relationship between the aperiodic offset in the mPFC and chronic pain.
Apart from the aperiodic exponent, the aperiodic offset is another parameter estimated when computing the aperiodic component. The aperiodic offset has a less clear physiological interpretation than the aperiodic exponent. Nevertheless, we also investigated whether it was altered in the mPFC in people with chronic pain or was related to pain intensity. Left panel: raincloud plots represent the aperiodic offsets of individual participants corrected for age. Bayes Factor (BF) was derived from a two-sided independent samples Bayesian t-test comparing the offsets of the two groups. Right panel: the scatter plot represents the aperiodic offsets and pain intensity ratings of people with chronic pain corrected for age. Grey lines indicate the linear regression slope (solid line) and the confidence interval (dashed lines). Bayes Factor (BF) was obtained from a Bayesian linear correlation between aperiodic offsets and pain intensity in people with chronic pain. We found moderate evidence against a difference in aperiodic offsets between people with chronic pain and healthy participants (Cohen’s d=–0.03, BF10=0.14). Furthermore, we found moderate evidence against a correlation between aperiodic offsets and average pain ratings (R=0.06, BF10=0.14).
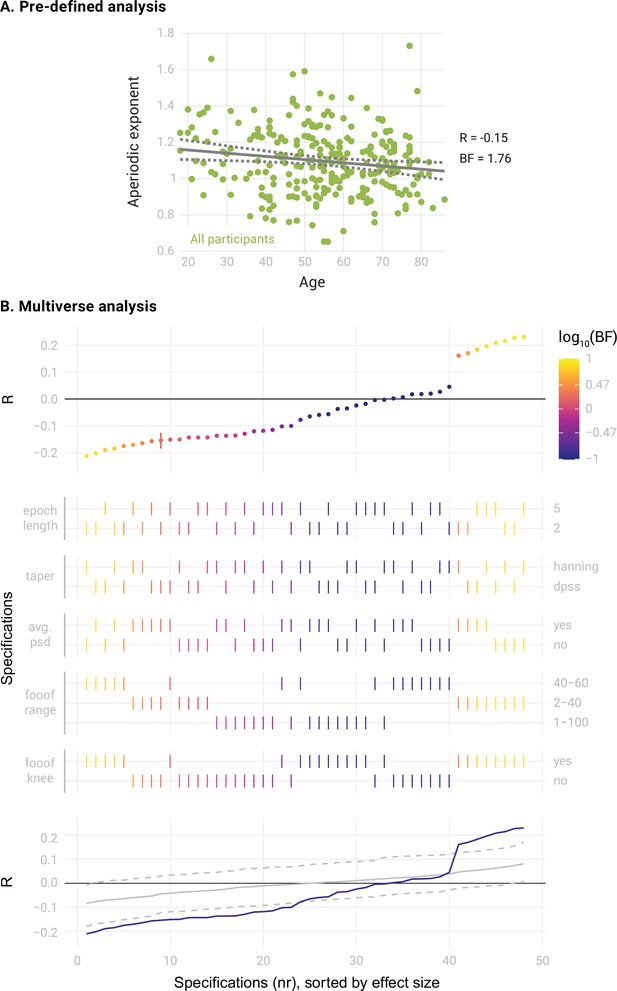
Relationship between the aperiodic exponent in the mPFC and age for all participants in the study (N=264).
We controlled for age effects by regressing out age from the aperiodic exponent, and pain ratings in all analyses. Previous literature has observed a negative relationship between aperiodic activity and age during maturation, mainly in the occipital area and in young participants (Hill et al., 2022; Tröndle et al., 2022; Turner et al., 2023). Thus, exploratively, we investigated the effects of age on aperiodic exponents in the mPFC in our dataset. We performed a Bayesian correlation between age and aperiodic exponents in the mPFC for all participants with the same parameters used in the pre-defined analyses. (A) Analysis according to the pre-defined settings for estimating aperiodic activity. The scatter plot represents the aperiodic exponent (y axis) and age (x axis). Grey lines indicate the linear regression slope (solid line) and the 95% confidence interval (dashed lines). Bayes Factor (BF) was obtained from a Bayesian linear correlation. The results show inconclusive evidence for a negative correlation between age and aperiodic exponents (R=–0.15, BF10=1.76). (B) Multiverse analysis. The upper panel visualizes the specification curve, which indicates the effect size (y axis) and evidence (color coded) for each specification (x axis). Specifications are ordered by effect size. A vertical bar indicates the specification of the pre-defined settings. The color scale’s upper and lower limits indicate strong evidence for and against a difference between groups. The middle panel further describes the parameters of each specification, with vertical bars indicating the parameters selected in each methodological decision (Table 1). The lower panel visualizes inference on the specification curve. In purple, the original specification curve is depicted. In grey, the null distribution of specification curves derived from 500 randomized curves is represented by the median curve (solid grey line) and 2.5th and 97.5th percentile curves (dashed lines). Intuitively, if the original curve lies between the 2.5th and 97.5th percentile curves, it is not significantly different from the null distribution of specification curves. Surprisingly, the Specification Curve Analysis revealed very strong evidence for a negative correlation in specifications 1–6 and a positive correlation in specifications 41–48. Statistical inference on the specification curve indicated that the curve was significantly different from a null distribution of specification curves (pmedian = 0.06, pshare = 0.006, paggregate = 0.002). Both ranges were associated with specific parameters of the ‘spectparam’ algorithm. This indicates that the set of parameters used to estimate the aperiodic exponent greatly impacts the existence and direction of a correlation between aperiodic exponents and age. Thus, further brain maturation studies interested in aperiodic activity should consider this. In our particular case, this analysis confirmed the necessity of controlling for age when studying aperiodic activity in chronic pain.
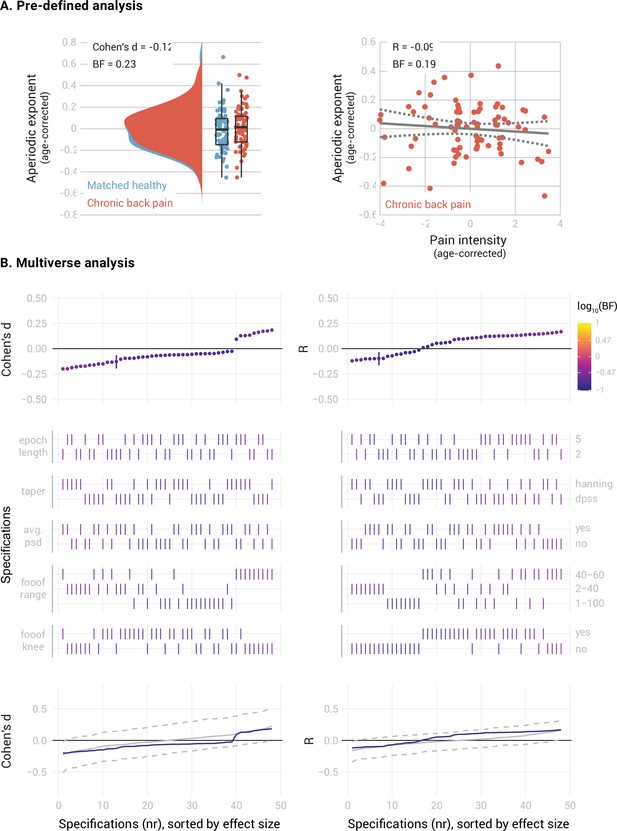
elationship between the aperiodic exponent in the mPFC and average pain intensity in people with Chronic Back Pain.
(A) Pre-defined analysis. (B) Multiverse analysis. The left column shows differences between people with chronic back pain (N=80) and a healthy subsample, matched in sample size, age, gender, and dataset belonging to the CBP sample (N=80). The right column shows the correlation between aperiodic exponents and pain intensity in people with CBP (N=80). Aperiodic exponents were corrected for age by regressing out age from the participants subsample (left panel) or the people with CBP sample (right panel). Pain intensity ratings were corrected for age by regressing out age from the people with CBP subsample.
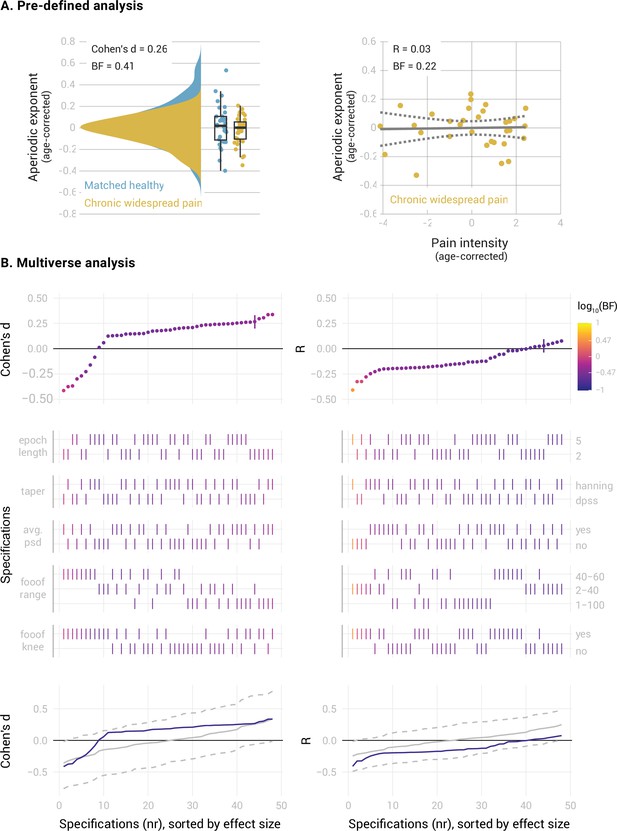
Relationship between the aperiodic exponent in the mPFC and average pain intensity in people with Chronic Widespread Pain.
(A) Pre-defined analysis. (B) Multiverse analysis. The left column shows differences between people with Chronic Widespread Pain (N=33) and a healthy subsample matched in sample size, age, gender, and dataset belonging to the CWP sample (N=33). The right column shows the correlation between aperiodic exponents and pain intensity in people with CWP (N=33). Aperiodic exponents were corrected for age by regressing out age from the participants subsample (left panel) or the people with CWP sample (right panel). Pain intensity ratings were corrected for age by regressing out age from the people with CWP subsample.
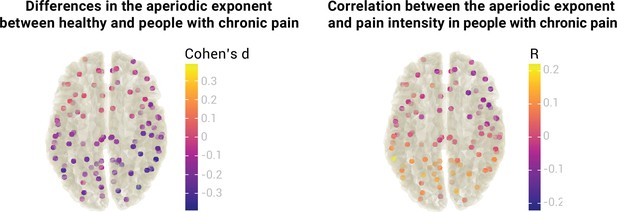
Relationship between the aperiodic exponent and chronic pain at a whole-brain level.
The left panel visualizes differences in the aperiodic exponent (Cohen’s d) between healthy participants (N=115) and people with chronic pain (N=149) at 100 different brain locations. The right panel visualizes the correlation between the aperiodic exponent and average pain intensity (Pearson’s correlation coefficient, R) in people with chronic pain at 100 different brain locations. No statistical differences or correlations were found in any location after correcting for multiple comparisons. Aperiodic exponents and pain ratings were corrected for age.
Tables
Analytical decisions and parameters investigated in the multiverse analysis.
Parameters in italics are the ones used in the pre-registered analysis. In total 48 different combinations of parameters (specifications) were investigated.
Analytical decision | Parameter | Description |
---|---|---|
Epoch length | 2 s | Length of the epochs in seconds. Epoch length affects the frequency resolution of the power spectrum. ‘2 s’ implies a frequency resolution of 0.5 Hz, ‘5 s’ of 0.2 Hz. |
5 s | ||
Taper | dpss | Method to estimate the power spectrum. ‘Dpss’ performs frequency analysis with multiple dpss tapers and 1 Hz spectral smoothing. ‘Hanning’ performs frequency analysis with a single hanning taper. |
hanning | ||
Avg. psd | yes | Method to summarize aperiodic activity on the mPFC. ‘Yes’ averages the power spectra of all the source locations encompassing the mPFC and derives the aperiodic exponent from this average. ‘No’ models the power spectrum for each location and averages aperiodic parameters obtained from each power spectra. |
no | ||
Fooof range | 2–40 Hz | Frequency range in which to model the power spectrum. ‘2–40 Hz’ is the pre-set value of the ‘spectparam algorithm’ (Donoghue et al., 2020). ’40–60’ is the original setting of Gao et al., 2017. ‘1–100’ includes the whole power spectrum. |
40–60 Hz | ||
1–100 Hz | ||
Fooof knee | no | Parameter that controls the bend of the model of aperiodic activity. ‘No’ omits the estimation of the knee. ‘Yes’ models the knee. |
yes |