Meta-Research: Gender variations in citation distributions in medicine are very small and due to self-citation and journal prestige
Abstract
A number of studies suggest that scientific papers with women in leading-author positions attract fewer citations than those with men in leading-author positions. We report the results of a matched case-control study of 1,269,542 papers in selected areas of medicine published between 2008 and 2014. We find that papers with female authors are, on average, cited between 6.5 and 12.6% less than papers with male authors. However, the standardized mean differences are very small, and the percentage overlaps between the distributions for male and female authors are extensive. Adjusting for self-citations, number of authors, international collaboration and journal prestige, we find near-identical per-paper citation impact for women and men in first and last author positions, with self-citations and journal prestige accounting for most of the small average differences. Our study demonstrates the importance of focusing greater attention on within-group variability and between-group overlap of distributions when interpreting and reporting results of gender-based comparisons of citation impact.
https://doi.org/10.7554/eLife.45374.001Introduction
Over the past four decades, the share of female graduates in medicine has increased from less than 10% to more than 50% in OECD countries, and recent statistics suggest near-parity in the representation of women and men as authors in medical research in Australia, Brazil, Chile, Europe and North America (Huggett, 2017; OECD, 2019). However, gender inequalities persist in the upper echelons of academic medicine. As of 2013, women constituted just 21% of full professors in the United States and just 23% in Europe, with the proportion of female department chairs and deans also remaining low (European Commission, 2016; Lautenberger et al., 2014).
These gender imbalances likely reflect myriad obstacles to women's career progress, including chilly and sometimes hostile work climates (Carr et al., 2003; Jenner et al., 2019; Pololi et al., 2013), bias in recruitment and selection practices (Van den Brink, 2011), societal cultures that still expect a strongly gendered division of domestic labor (Jolly et al., 2014), an underrepresentation of women in last-author positions (González-Álvarez and Cervera-Crespo, 2019; Jagsi et al., 2006; Lerchenmueller and Sorenson, 2018), and disparities in research funding (Jagsi et al., 2009; Sege et al., 2015). Given that citation indicators are increasingly being used to inform tenure, hiring and funding decisions in many areas of the medical sciences, possible gender differences in citation impact have the potential to contribute to the perpetuation of these inequalities.
A survey of the literature revealed 22 papers on gender and citations in the medical sciences published between 2006 and 2016 (see Supplementary file 1). The study designs, impact measures and statistics used in these papers are too heterogeneous for meta-analytical comparisons, and this literature is also characterized by notable variations in results depending on specialty, country, study design and type of citation indicator (h-index, citations per paper, cumulative citations, m-quotient and journal impact factor). Some studies report an average citation advantage in favor of men (see e.g. Larivière et al., 2011; Nielsen, 2016); others do not observe any notable gender difference (see e.g. Mirnezami et al., 2016; Pagel and Hudetz, 2011). Existing articles are in most cases based on convenience samples and limit their focus to single specialties or sub-specialties (16 out of 22), and the literature is characterized by a North American bias, with only five studies focusing on countries outside the US and Canada. Furthermore, only six of the papers report direct comparisons of the average number of citations per paper for male and female authors (Housri et al., 2008; Larivière et al., 2011; Mirnezami et al., 2016; Nielsen, 2016; Pagel and Hudetz, 2015; Pagel and Hudetz, 2011).
Researchers have also studied gender and citation distributions in fields other than medicine, and again these studies are characterized by ambiguous results that vary by geographical focus, time period and discipline. Some report average differences in favor of male authors (Aksnes et al., 2011; Caplar et al., 2017; Eagly and Miller, 2016; Maliniak et al., 2013), some report smaller average differences in favor of female authors (Borrego et al., 2010; Long, 1992; van Arensbergen et al., 2012), and some report no discernable gender difference (Nielsen, 2017; Slyder et al., 2011; Symonds et al., 2006).
A recurring limitation in the literature is the lack of attention paid to within-group variability and between-group overlap in citation distributions. Many studies rely on simple, mean-based comparisons to derive generic conclusions about gender differences (or similarities) in per-paper citation impact. This practice can be misleading for at least two reasons. First, the reported gender differences are generally small, and will, given the expected distribution for citation data, imply a great deal of overlap for women and men (we return to this below). Second, the lack of attention to co-varying factors influencing a paper’s likelihood of being cited (e.g. institutional affiliation, country-affiliation, self-citations and number of authors) will inevitably limit what can be learned from bivariate comparisons of this sort.
Here we report the results of a comprehensive, global analysis of possible gender variations in the per-paper citation impact of medical researchers. We analyzed 1,269,542 papers on disease-specific medical research published between 2008 and 2014. To reduce confounding and ensure balanced case-control groups, three matching covariates (institutional prestige, geographic location and medical specialty) were used to generate three datasets: sample 1 had female first authors as the case and male first authors as the control (n=1,018,665); sample 2 had female last authors as the case and male last authors as the control (n=653,233); and in sample 3, pairs of female first and last authors constituted the case group and all other author combinations were included in the control group (n=368,374). The outcome variable was field-normalized citations per paper, and regression analyzes were used to explore the influence of additional co-varying factors (self-citations, number of authors, international collaboration and journal prestige) on differences in per-paper citation impact (see Methods). Given the large sample size, global scope, and matched design, our study is less vulnerable to biases resulting from sample-specific variance, confounders and selection than previous studies in the medical field.
Results
Table 1 specifies the gender composition of the unmatched sample (n=1,269,542) by main specialty, institutional prestige and geographic location. Male researchers dominate all five main specialty groupings. Female last authors are underrepresented, in comparison to their representation in the global population, in all five groupings, but most notably in Surgical/Procedural specialties. The proportion of female first and last authors is highest in Latin America and lowest in South East Asia. Note here that numerous countries located in Eastern Asia have been excluded from the analysis due to unreliable gender disambiguation based on first-name and country information (see Methods for more details). In Western Europe and North America the proportions of female first and last authors lie close to the Global averages.
Women’s share of authorships overall, across five main specialties, institutional prestige, and geocultural area.
f_w is the weighted proportion of women per paper, f_first the proportion of female first authorships, f_last the proportion of female last authorships, f_both the proportion of papers where women are both first and last authors.
Overall | f_w | f_first | f_last | f_both |
---|---|---|---|---|
0.35 | 0.40 | 0.26 | 0.15 | |
Main specialty | f_w | f_first | f_last | f_both |
Basic science | 0.39 | 0.46 | 0.30 | 0.18 |
Hospital based | 0.37 | 0.43 | 0.28 | 0.16 |
Medical | 0.33 | 0.38 | 0.24 | 0.13 |
Pediatric | 0.46 | 0.52 | 0.37 | 0.24 |
Surgical/procedural | 0.29 | 0.32 | 0.21 | 0.11 |
Institutional prestige | f_w | f_first | f_last | f_both |
Top-100 University | 0.36 | 0.42 | 0.27 | 0.16 |
Other university | 0.35 | 0.39 | 0.25 | 0.14 |
Geographic location | f_w | f_first | f_last | f_both |
Arab countries | 0.33 | 0.34 | 0.27 | 0.16 |
Commonwealth of Independent States | 0.40 | 0.45 | 0.30 | 0.17 |
East Asia | 0.19 | 0.19 | 0.09 | 0.04 |
Latin America | 0.46 | 0.52 | 0.39 | 0.25 |
North America | 0.36 | 0.40 | 0.27 | 0.15 |
Oceania | 0.40 | 0.48 | 0.31 | 0.20 |
South and Central Europe | 0.40 | 0.44 | 0.31 | 0.18 |
Sub-Saharan Africa | 0.36 | 0.39 | 0.31 | 0.20 |
South-West Asia | 0.29 | 0.31 | 0.24 | 0.10 |
Western Europe | 0.35 | 0.42 | 0.24 | 0.14 |
Citation impact per paper is measured by field-normalized citation scores with a four-year fixed citation window (NCS). Using NCS as the outcome variable strengthens our matched design by adjusting for sub-specialty variations in citation practices. NCS is a continuous outcome variable. It is calculated by dividing the citations accrued by a paper within the first four years after publication with the expected citation score of other papers published in the same year and field (Waltman et al., 2012). Fields are delineated using the same article-level classification system as the Leiden Ranking. This classification system consists of 4,047 micro-level clusters of publications and offers one of the best, current approaches to item-oriented field normalization (Waltman and van Eck, 2012). This item-oriented field normalization procedure allows for comparison of papers published in different sub-fields, with different publication dates.
Figure 1 displays the density distributions of log-transformed citation scores for the matched sets of papers with female first authors and male first authors (Sample 1), female last authors and male last authors (Sample 2), and female first and last authors and other gender combinations of first and last authors (Sample 3). For all distributions, the absolute uncertainty of the mean is between 0.001 and 0.005. On average, papers with female first authors are cited 8.7% less than papers with male first authors (Sample 1. Female first authors: n = 509,330; x̄ = 1.16; σ = 1.83; x̃ =0.73. Male first authors: n = 509.335; x̄ = 1.27; σ = 2.00; x̃ =0.76); however, the overlap between the two distributions is extensive (Cohen's d = −.06; Weitzman's Δ = 95.4%; Weitzman, 1970). Papers with female last authors are cited 6.5% less than papers with male last authors (Sample 2. Female last authors: n = 326,611; x̄ = 1.16; σ = 1.93; x̃ =0.72. Male first authors: n = 326,622; x̄ = 1.24; σ = 1.93; x̃ =0.76); again, the overlap between the two distributions is extensive (Cohen's d = −.04; Weitzman's Δ = 95.6%). Finally, papers in which both the first and last authors are female are cited 12.6% less than papers with other gender combinations (Sample 3. Female first and last authors: n = 184,183; x̄ = 1.11; σ = 1.75; x̃ =0.71. Other combinations: n = 184,191; x̄ = 1.27; σ= 2.28; x̃ =0.76); again, the overlap between the two distributions is extensive (Cohen's d = −.08; Weitzman's Δ = 93.1%).
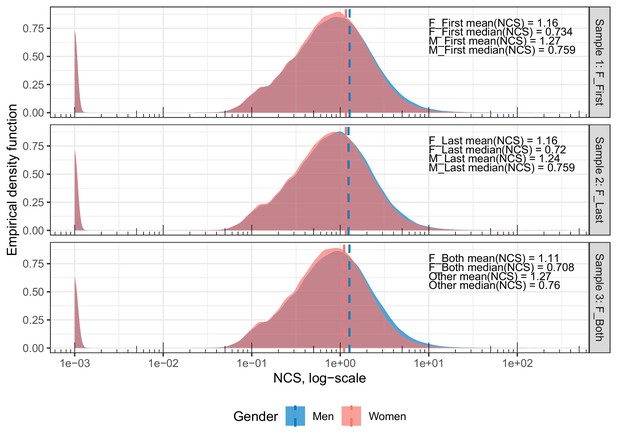
Density distributions of the log-transformed, per-paper NCS for the matched set of male and female first authors (Sample 1), female and male last authors (Sample 2), and female first and last authors vs. other author combinations (Sample 3).
Dashed lines indicate the mean NCS for each sample. The y-axis indicates the proportion of papers found in that area of the NCS, equivalent to a smoothed histogram. The x-axis gives the per-paper NCS on a log-transformed scale. For all distributions, between-group overlap is extensive (93.1% to 95.6%). The difference between men and women is most clearly seen in the exceptionally highly cited studies, of which there are relatively few. Please note that. 001 (=1e-03) has been added to NCS in order to include uncited papers. The left-most peak in each sample represents uncited papers. The proportion of uncited papers per sample is 5.7%, 6.1%, and 5.9% for the case papers and 5.9%, 5.9%, and 5.8% for the control papers.
To obtain a closer approximation of the observed gender variation on the right side of the distribution curves (Figure 1), we calculated the percentage share of case papers among the top 5% and top 10% most cited papers in each sample. Note here that the case and control-papers, given our matching approach, each comprise 50% of all papers in Samples 1, 2, and 3. Our calculations showed that papers with female first authors comprise 43.6% of the top 5% most cited and 45.5% of the top 10% most cited in Sample 1. Papers with female last authors comprise 45.8% of the top 5% most cited and 46.8% of the top 10% most cited in Sample 2. Finally, papers in which both the first and last authors are female comprise 41.1% of the top 5% most cited and 43.5% of the top 10% most cited in Sample 3.
While these results indicate large within-group variation and very small between-group differences, they are consistent with previous reports of a slight average citation advantage for papers by male lead authors. We decided to use regression analyses to explore the underlying variations that may drive this average difference.
We ran Tweedie regressions to estimate the residual effect of author gender after adjusting for self-citations, numbers of authors per paper, field-normalized journal impact (MNCS journal) and international collaboration. MNCS journal is calculated as the mean-normalized citation score (NCS) of all papers published in a journal in a given year; in this case the same year as the observed paper. MNCS journal is essentially a measure of journal prestige that takes into account that most journals publish papers in more than one field, and that different fields have different citation characteristics. (See Methods for a discussion of the relationship between NCS and MNCS).
Exponentiated beta coefficients and 95% confidence intervals for the three models with NCS as outcome are displayed in Figure 2. The exponentiated beta coefficients should be interpreted as, ceteris paribus, the predicted relative change in the outcome resulting from a one unit increase in the predictor (Coates et al., 2018). For instance, an exponentiated coefficient of 0.95 for the case variable in Sample 1 (male first author = 0, female first author = 1) would indicate that female first authors, on average, receive 0.95 times the citations accrued by comparable papers with male first authors, hence on average 5% fewer citations.
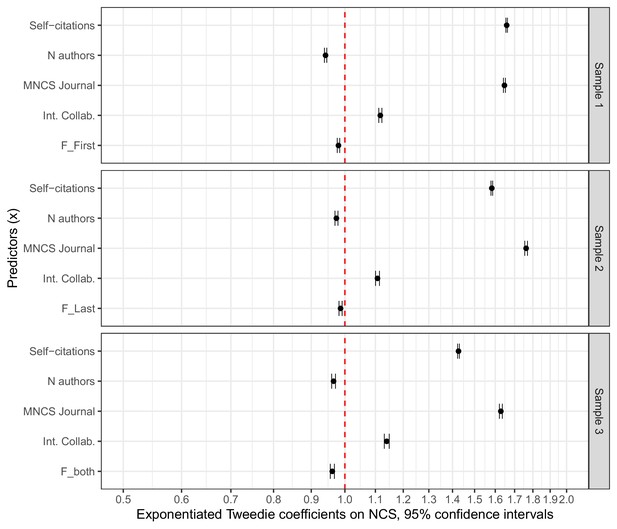
Standardized, exponentiated coefficients for the predictors included in the Tweedie regressions.
Error bars represent 95% confidence intervals (see Figure 2—source data 1 for estimate specifications and dispersion parameters). All regressions are based on matched samples. Sample 1 compares papers with female first authors to those with male first authors. Sample 2 compares papers with female last authors to those with male last authors. Sample 3 compares papers with female first and last authors to those with other author combinations. Values are on a logarithmic scale. The figure indicates very small residual effects of gender on NCS (case variables: F_First, F_Last and F_Both).
-
Figure 2—source data 1
Tweedie regression results.
- https://doi.org/10.7554/eLife.45374.005
-
Figure 2—source data 2
Regression results for Tweedie regressions on the full, unmatched sample, using NCS as outcome.
- https://doi.org/10.7554/eLife.45374.006
-
Figure 2—source data 3
Regression results for the three negative binomial regressions with times cited (CS) as outcome.
- https://doi.org/10.7554/eLife.45374.007
-
Figure 2—source data 4
Tweedie regression of standardized parameters, using MNCS Journal quantiles rather than measurements.
- https://doi.org/10.7554/eLife.45374.008
The numeric input variables have been rescaled by dividing by two standard deviations (Gelman, 2008). We did this to allow the numeric inputs (i.e. MNCS journal, N authors, self-citations) to be interpreted on the same scale as the binary case variables (i.e. F_first, F_last, F_both). The standardized coefficients should be interpreted as two-standard deviation changes on a logit scale, from a low value to a high value (Gelman, 2008). Figure 2—source data 1 summarizes the exponentiated values for both the direct and standardized coefficients.
The models indicate very small residual effects of author gender on citation impact per paper. The exponentiated coefficient for the case variable, F_first in Sample 1 (F_first: female first author=1, male first authors=0) is 0.98 (CI=0.98–0.98). The exponentiated coefficient for the case variable in Sample 2 (F_last: female last author=1, male last author=0) is 0.99 (CI=0.98–0.99), and the exponentiated coefficient for the case variable in Sample 3 (F_last: female first and last authors=1, all other author constellations=0) is 0.96 (CI=0.96–0.97). MNCS journal and self-citations are the strongest predictors in the models although their effect sizes can be considered small. The two remaining covariates (N authors and International collaboration) both have exponentiated coefficients close to one, indicating small effects.
Figure 3 displays the estimated marginal means (EM-means) and 95% CIs for the case variables in Samples 1, 2 and 3. The EM-means are used to report the predicted, average citation score per paper for each group, adjusting for self-citations, number of authors, MNCS journal and international collaboration. In accordance with Figure 1, we observe only very small differences between the EM-means for the case and control groups in the three samples (Figure 3). Robustness checks were carried out to examine the sensitivity of the regression results to alternative model and sample specifications (for specifications see Methods). All of these analyses yielded qualitatively similar results. All of the models indicated very small residual effects of author gender on citation impact per paper (see Figure 2—source datas 2–4).
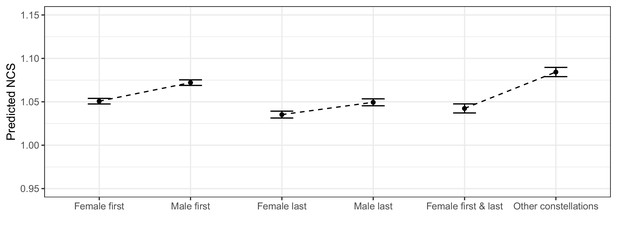
Plot of estimated marginal means for the case and control groups in Samples 1, 2 and 3.
The error bars display 95% confidence intervals. The figure visualizes the predicted, average, differences in per-paper citation scores for the case and control groups after adjusting for self-citations, number of authors, MNCS journal, and international collaboration. Sample 1 compares papers with female first authors to those with male first authors. Sample 2 compares papers with female last authors to those with male last authors. Sample 3 compares papers with female first and last authors to those with other author combinations. Note that the y-axis has a restricted span from. 95 to 1.15. The comparisons indicate trivial, average gender differences.
To examine which of the covariates that vary the most by lead-author gender, we ran three logistic regressions with F_first as outcome in Sample 1, F_last as outcome in Sample 2, and F_both as outcome in Sample 3. The results are presented in Figure 4. Again, the regression inputs have been rescaled by dividing by two standard deviations to allow for meaningful comparisons of the binary and numeric variables. Figure 4—source data 1 summarizes both the direct and the standardized coefficients. Self-citations have the largest standardized coefficients in all three samples, followed by N authors in Samples 1 and 2, and MNCS journal in Sample 3. We observe no discernable effect of international collaboration on the outcome variable in any of the samples. The results indicate that papers with high self-citation rates and high MNCS journal scores are less likely to be led by female authors than male authors in all samples. Notice that the effect sizes are small. Further, papers with a high value of N authors are slightly more likely to have a female first author than a male first author, and slightly less likely to have a female last author than a male last author. As indicated in Figure 2, N authors has exponentiated coefficients extremely close to 1.0 in the Tweedie regressions with NCS as outcome. Hence, we restrict our focus to self-citations and MNCS journal in the remaining part of the analysis.
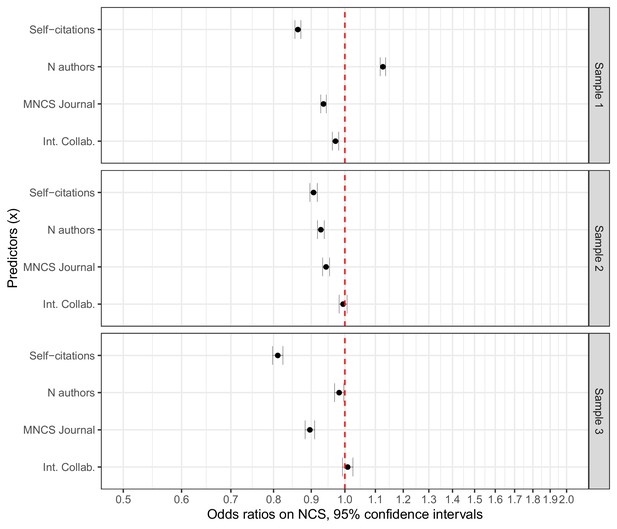
Odds ratios for the standardized predictors included in the logistic regressions.
Error bars represent 95% confidence intervals (see Figure 4—source data 1 for information on estimates and dispersion parameters). All regressions are based on matched samples. Sample 1 compares papers with female first authors to those with male first authors. Sample 2 compares papers with female last authors to those with male last authors. Sample 3 compares papers with female first and last authors to those with other author combinations. The figure indicates that self-citations is the variable that varies the most along gender lines in all three samples, albeit the effects can be considered small.
-
Figure 4—source data 1
Logistic regression results.
- https://doi.org/10.7554/eLife.45374.011
Descriptive analysis indicates larger average gender differences in self-citation rates compared to MNCS journal scores in Samples 1, 2 and 3 (Table 2), but the standardized mean differences for both variables are very small, and the percentage overlaps are extensive.
Means, standard deviations, medians, Cohen’s d, and Weitzman’s ∆ for case-control comparisons of self-citations and MNCS journal in Samples 1, 2 and 3.
Cohen’s d and Weitzman’s ∆ are calculated with two and one decimal respectively. Weitzman’s ∆ is not calculated for self-citations, as it is a discrete count variables. For sample 1, female first authors is the case and male first authors is the control. For Sample 2, female last authors is the case and male last authors is the control. For Sample 3, female first and last authors is the case and other combinations are the control.
case (σ) | control (σ) | case | control | d | ∆ | ||
---|---|---|---|---|---|---|---|
Sample 1 | Self-citations | 1.91 (3.18) | 2.16 (3.93) | 1 | 1 | -0.07 | |
MNCS journal | 1.16 (.90) | 1.21 (1.04) | .99 | 1.00 | -0.05 | 96.4% | |
Sample 2 | Self-citations | 1.84 (3.22) | 2.08 (3.77) | 1 | 1 | -0.07 | |
MNCS journal | 1.14 (.98) | 1.20 (.99) | .98 | 1.00 | -0.06 | 95.6% | |
Sample 3 | Self-citations | 1.74 (2.84) | 2.13 (3.91) | 1 | 1 | -0.11 | |
MNCS journal | 1.12 (.97) | 1.20 (1.02) | .97 | 1.0 | -0.08 | 93.4% |
To obtain a closer approximation of the extent to which self-citations may contribute to explain the observed gender variation on the right side of the distribution curves in Figure 1, we plotted the average proportion of per-paper self-citations (number of per-paper self-citations/raw per-paper citation scores) in 5% intervals from the quantile of least cited papers to the quantile of top cited papers in Samples 1, 2 and 3. As displayed in the upper panel of Figure 5, the average proportion of self-citations for papers in the top 5% bin is ~15% in all three samples. This implies that at least part of the gender variation observed on the right side of the curves in Figure 1 may be attributable to average gender differences in self-citation rates per paper. It should also be noted that our citation indicators are calculated with a four-year window, which may contribute to explain the relatively large proportion of self-citations in the samples.
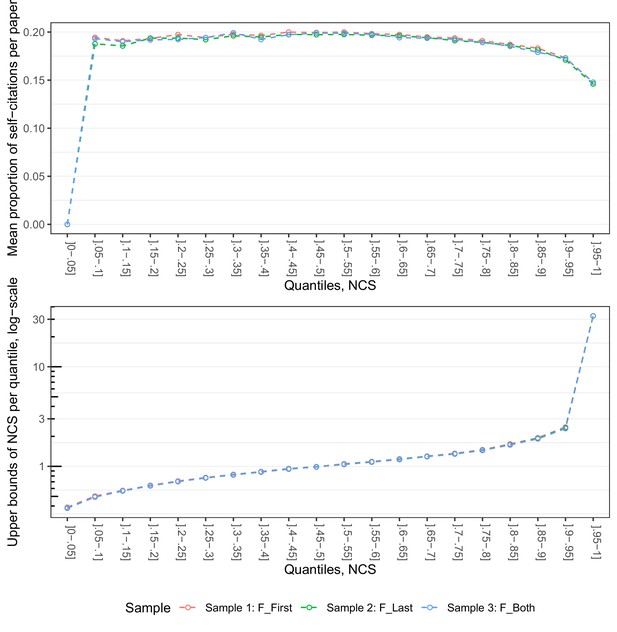
The upper panel shows the distribution of self-citations by five-percentile bins of NCS for each sample.
The average proportions of self-citations are given on the y-axis, the five-percentile bins of NCS on the x-axis. The lower panel displays the distribution of the upper bounds of NCS across the five-percentile bins of NCS. The upper bounds of NCS are given on the y-axis, and the five-percentile bins on the y-axis.
Finally, to examine the observed gender variation in MNCS journal scores in closer detail, we plotted the average proportion of case papers (i.e. papers with female first authors in Sample 1, papers with female last authors in Sample 2, and papers with female first and last authors in Sample 3) in 5% intervals – from the papers with the lowest to the highest MNCS journal scores. Note again that the case papers and the control papers each comprise 50% of all papers in Samples 1, 2, and 3. As displayed in the upper panel in Figure 6, the relative proportion of case papers is slightly overrepresented on the left side and in the middle part of the x-axis and slightly underrepresented on the right side. The relative proportion of case papers drops below 50% at the 80th percentile. The most notable drop occurs at the 90th–95th percentile. This indicates that most of the observed, average gender differences in MNCS journal scores are due to an underrepresentation of female-led papers in the most high impact publication outlets. Of the papers published in the top 5% outlets with the highest MNCS journal scores, papers with female first authors and female last authors comprise 45% and 44%, respectively, while papers with female first and last authors comprise 41%.
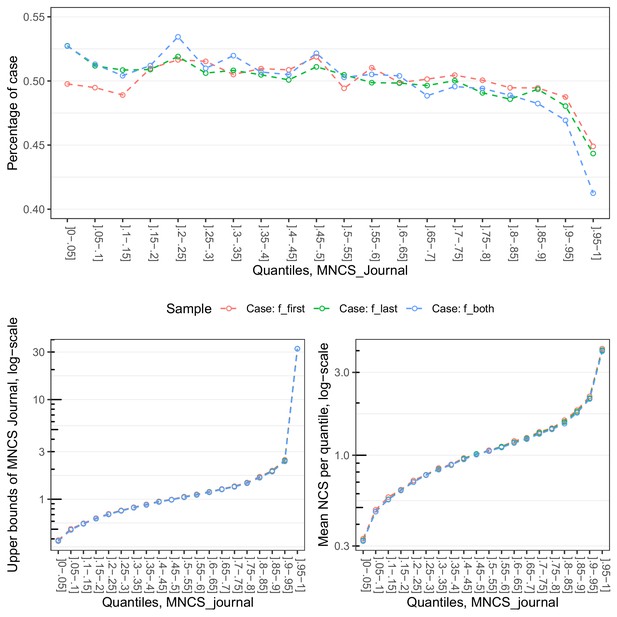
The upper panel shows the proportions of papers with female first authors in Sample 1, female last authors in Sample 2, combinations of female first and last authors in Sample 3, by five-percentile bins of MNCS.
The proportions of case papers are given on the y-axis, and the five-percentile bins of MNCS journal on the y-axis. The lower-left panel displays the upper bounds of MNCS journal by five-percentile bins of MNCS journal for each sample, while the lower-right panel shows the mean NCS by five-percentile bins of MNCS journal for each sample. The upper bounds of MNCS journal (left) and Mean NCS (right) are given on the y-axes, and the five-percentile bins of MNCS journal on the x-axes.
Discussion
Decision-makers in academic medicine increasingly use citation-based metrics to evaluate scholarly merits and allocate individual opportunities and rewards. Examining data to discern systematic gender differences in medical researchers’ citation impact is therefore more important than ever. However, existing evidence from the medical sciences falls short of providing tangible guidance for policy on this issue. In this study, we carried out a controlled, large-scale, global gender comparison of lead authors’ average citation impact per paper in disease-specific medical research.
Descriptive analysis indicated average gender differences of 6.5 to 12.6% in the three matched samples. However, the standardized mean differences were very small, and the percentage overlaps between male and female distributions were extensive.
In regressions adjusted for international collaboration, self-citations, number of authors, and field-normalized journal impact, the average citation impact per paper was close to identical for women and men, irrespective of first and last author combinations. Additional analyses indicated that self-citations and field-normalized journal impact accounted for most of the average differences observed in bivariate gender comparisons.
Compared to previous studies, these findings align with what is known about “decline effects" (Ioannidis, 2005). As sample sizes become larger and study designs more advanced, effect sizes tend to decline towards minuscule effects.
Here, it is relevant to highlight that the gender effect attributable to self-citations likely reflects a generation effect, with more senior male authors having more publications to self-cite. For instance a recent analysis of 1.5 million life-science papers demonstrates that observed gender differences in self-citation rates are leveled out when adjusting for each author’s prior publication output (Mishra et al., 2018; see also King et al., 2017).
Given this latent generation effect, one could argue that it is somewhat surprising that we end up observing very small residual effects of author gender on per-paper citation impact. If the male authors in our sample, on average, are older and more established researchers, one would expect them to have a slight citation advantage in a cross-sectional analysis like ours.
Note also that women may still receive less credit for their citations due to gender-based double standards in evaluative judgments (Botelho and Abraham, 2017; Moss-Racusin et al., 2012). For instance, a sophisticated analysis of scientists receiving early-career grants from the National Institutes of Health in the US (1985–2009) shows that women compared to men gain fewer returns on citations in terms of transition time from a postdoc grant to an R01 grant. Adjusting for a large number of factors, women on average spent one year longer transitioning to an R01 grant than men with the same number of citations (Lerchenmueller and Sorenson, 2018).
The observed differences in the relative proportion of female- and male-led papers published in the journals with the highest field-normalized impact echo the findings of previous research in the medical sciences (Jagsi et al., 2006; Lerchenmueller and Sorenson, 2018). Part of this difference may be due to the generation effect described above. Other possible explanations may be that female lead authors are less likely to submit their research to journals with high impact factors (Berg, 2017; Nature Neuroscience, 2018), or that they have slightly lower success rates in peer review, e.g. due to gender bias or topic bias in journals with high impact factors. In the future, we hope to see closer examinations of the mechanisms driving this gender gap. This issue is critical given the strong emphasis on publishing in journals with high impact factors in evaluations of the performance of individual researchers (McKiernan et al., 2019).
Building diverse and inclusive research organizations requires careful attention to the mechanisms perpetuating gender inequalities in the higher academic ranks. The results reported here demonstrate that gender differences in per-paper citation impact are a negligible factor in this stratification process. Instead, universities and research leaders should develop strategies for effectively counteracting the broader societal and institutional barriers to the scientific advancement of women, including chilly and hostile work climates (Carr et al., 2003; Pololi et al., 2013), gender bias in recruitment and selection practices (Van den Brink, 2011) and work-family conflicts in the early stages of the academic career (Jolly et al., 2014).
Counteracting such barriers may be critical to improving the rigor and precision of medical research. For instance, an association between the representation of women as last authors (in combination with male first authors) and adequate statistical power in clinical trials was reported recently (Otte et al., 2018). Another recent study suggests a robust positive correlation between the attention to gender- and sex-related variations in medical research and the involvement of women as first and last authors (Nielsen et al., 2017).
Our study has some limitations. First, the analysis excluded articles with first or last authors from 18 countries, due to unreliable gender determination (see Methods). Despite a relatively small reduction in sample size (7.2% of the total population), this exclusion implies that countries located in East Asia and Sub-Saharan Africa, are underrepresented in the analysis, and the results should be interpreted accordingly.
Second, this study limited its focus to average gender differences in citation impact per paper. This focus precludes us from drawing any conclusions on potential long-term differences in male and female researchers’ cumulative citation impact. If men, for instance, have higher average publication rates (e.g. due to shorter career breaks, more collaborative research articles, more funding and more people in their labs), this would imply that their average cumulative citation impact would be higher as well. Hence, while differences in per-paper citations appear to be a negligible factor in the perpetuation of gender inequalities in academic medicine, disparities in publication rates may still play a role – especially at the early-career stages and especially in evaluation systems where publication rates have a strong influence on decisions about tenure, hiring and funding. Future research could use author-disambiguation algorithms to compare the cumulative citation impact and publication rates of large samples of individual researchers over time, adjusting for institutional affiliation, country affiliation, research area, scientific age and other relevant factors.
Third, per-paper citation impact represents a very specific proxy of scholarly impact. In practice, research evaluators at funding organizations and universities may use citation indicators in other ways, e.g. by restricting their focus to the five most influential publications of an applicant. Indeed, having a few highly cited papers may in some evaluative contexts do more for a researcher’s career progression than having a higher than average per-paper citation impact.
Fourth, the relatively small gender differences observed in the descriptive analysis should be interpreted with caution. Such results are sensitive to generic noise in the data (Schneider, 2013), and inherent uncertainties associated with statistical inferences based on non-random samples of “found data” (Freedman et al., 2003).
In conclusion, our results demonstrate that adjusting for co-varying factors, men and women in first and last author positions are cited at similar rates. The analysis presented here raises concerns that at least parts of the gender differences reported in prior research may be distorted by methodological limitations and imprecision in how the results are interpreted. We acknowledge the critical importance of recognizing even the small drawbacks that can add up over time and become cumulative disadvantages for women in science (Caplan, 1993; Cole and Singer, 1991). However, our study demonstrates the importance of focusing greater attention on within-group variability and between-group overlap of distributions when interpreting and reporting results.
Methods
Figure 7 displays the data-selection process. Peer-reviewed articles published between 2008 and 2014 were collected in PubMed Medline. To target core medical research and enable exact matching based on primary medical specialty, we needed information on the disease-specific Medical Subject Headings assigned to each paper. Hence, the initial sample was limited to records indexed with the broad MeSH descriptor “Diseases Category" (n=2,336,805). Eligible PubMed records were matched to article metadata in Web of Science (WoS) (citation data, author first names and affiliations), using Publication identifiers (PMID, DOI) and a fuzzy matching of reference data (source, volume, pagination, etc.). The matching percentage by journal is given in Figure 7—figure supplement 1. All papers lacking full first-name information for one or more authors were excluded from the sample (n=362,453; 15.5% of the population sample). The name-to-gender assignment algorithm, Gender API (Gender API, 2016), was used to determine the gender of all authors per paper for the remaining sample. This algorithm estimates a given author's likelihood of being a man or a woman based on first name and country affiliation. The accuracy of the algorithm has previously been validated in a random subsample (N=500 authors) drawn from the same dataset (Nielsen et al., 2017), and was recently evaluated as the best-performing service, in a bench-mark of five name-to-gender assignment algorithms (Santamaría and Mihaljević, 2018). Gender API provided valid name-to-gender estimates for 1,434,715 papers (61.4% of the population sample) (for further specification on Gender API, See Figure 7—figure supplement 3). A sensitivity analysis indicated unreliable Gender API estimates for authors from 18 countries, located in Eastern Asia and Sub-Saharan Africa. All documents with first and last authors from these countries were excluded (see Figure 7—figure supplement 2). This reduced the sample by 7.2% (n=165,173), resulting in a final sample of 1,269,542 papers (54.3% of the population sample).
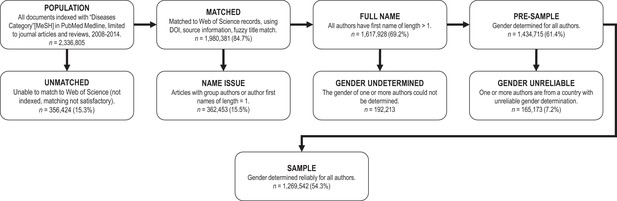
Flowchart of data collection, inclusion and exclusion.
https://doi.org/10.7554/eLife.45374.015-
Figure 7—source data 1
Excluded countries due to unreliable gender assignments from first name.
- https://doi.org/10.7554/eLife.45374.019
-
Figure 7—source data 2
List of specialty and main specialty designation, and number of papers per specialty for the full sample.
- https://doi.org/10.7554/eLife.45374.020
-
Figure 7—source data 3
Groupings of countries by geographical region.
- https://doi.org/10.7554/eLife.45374.021
In the analysis of citation impact per paper, exact matching covariates were included for institutional prestige, geographical region and medical specialty. All three factors are known to influence citation impact (Judge, 2016; Stremersch et al., 2007; van Eck et al., 2013). In addition, research shows that the participation of women in medical research varies considerably across geographical regions, top and lower-tier research institutions and medical specialties (Lautenberger et al., 2014; Nielsen et al., 2017; Weeden et al., 2017). Matching of institutional prestige was based on a binary variable specifying whether a paper includes authors affiliated with a top-100 university according to the Leiden Ranking [www.leidenranking.com]. The matching of geographical region was based on ten variables specifying the location of the first and last author. The matching of medical specialties was based on 124 specialties identified using the HeTOP MeSH specialty-classification algorithm (Darmoni et al., 2006) (for specifications on country groupings and specialty-disambiguation, see Figure 7—source datas 1–3). We used replacement sampling, resulting in case and control groups of equal sizes.
Five covariates were included in the regression models. Journal prestige is arguably the strongest single predictor of a paper's citation impact (Judge, 2016). Prior work suggest that women are less likely than men to publish in journals with high impact factors (see, for example, González-Álvarez and Cervera-Crespo, 2019; Lerchenmüller et al., 2018). To adjust for this factor, we computed the mean NCS-score per journal (MNCS journal). This indicator is advantageous compared to the journal impact factor, most notably because it corrects for subfield-specific citation characteristics.
International collaboration is another recognized predictor of citation impact (Smith et al., 2014). Again, extant research suggests that women, on average, co-author fewer papers with international colleagues compared to men (see e.g. Abramo et al., 2013; Larivière et al., 2013). A binary variable adjusts for this factor (collaboration between authors from different countries = 1).
Finally, we included two count variables that adjust for the number of authors per paper and the number of per-paper self-citations within the first four years after publication. Extant research demonstrates that per-paper citation impact is positively correlated with author-group size, and that women, on average, have fewer self-citations and fewer collaborators per paper (see e.g. Araújo et al., 2017; King et al., 2017).
A Tweedie distribution was used to estimate the relationship between author gender and NCS (Funk et al., 2010; Jørgensen, 1987). The continuous outcome variable, NCS, is highly right-skewed with a probability mass at zero. Tweedie distributions are a class of mixed compound Poisson-gamma distributions with a discrete mass at zero. This makes them useful for modeling continuous outcome variables with a mixture of zeros and positive values. Tweedie distributions belong to the exponential family of generalized linear models (GLM). The mean and variance for the Tweedie random variable are and , respectively, where is the dispersion parameter and p is the parameter controlling the variance of the distribution. Tweedie distributions take variance-power values p in the range >1 and < 2. We estimated three basic GLM-models using link power=0 corresponding to a log-link function and variance power of p=1.65, p=1.72 and p=1.6 for the three models, F_first, F_last and F_both, respectively. Variance power was derived empirically through iterative algorithms seeking an optimal fit. The dispersion parameter was used to test for goodness of fit and examine possible overdispersion. Robustness checks were carried out to examine the sensitivity of the results to alternative model and sample specifications (see Figure 2—source datas 2–4). First, we ran negative binomial regressions with raw per-paper citation scores (with a four-year citation window) (CS) as the outcome variable in Samples 1, 2 and 3. Next, we ran Tweedie regressions with NCS as outcome variable based on the full, un-matched data set. Finally, we ran Tweedie regressions with dummy variables for different levels of MNCS journal (low, medium, and high). This allowed us to examine whether adjusting for journal prestige at different thresholds influenced the case coefficients in Samples 1, 2, and 3. The dummy variables were created based on percentile ranks of MNCS journal. The percentile thresholds were ≥ 95% for the high-category variable, ≥ 50% < 95% for the medium-category variable and < 50% for the low category variable.
Logistic regression was used to estimate the relationship between the four covariates (self-citations, N authors, MNCS journal and international collaboration) and the case variable in each sample.
The predictors and covariates in all the regression models had Variance Inflation Factors below two, indicating very low levels of multicollinearity.
The statistical analyses were conducted in R version 3.4.3. For the matching procedure, we used the R “Matching" package (Sekhon, 2011), for the Tweedie regressions we used the “tweedie" and “statmod" packages (Dunn, 2017; Dunn and Smyth, 2008; Dunn and Smyth, 2005). Finally, we used the “emmeans’ package to calculate the estimated marginal means for the case variables in the Tweedie regressions (Lenth, 2019).
Information for the calculation of bibliometric indices (CS, NCS, JS, MNCS journal, self-citations and international collaboration) were obtained from the Centre for Science and Technology Studies (CWTS), Leiden University. CWTS hosts a curated, quality-added version of the Web of Science which enables the calculation of field-normalized citation indicators, which is not immediately possible in the standard version available online. Calculation methods are standard operations, as described in Waltman et al. (2012). For clarity, we briefly explain the NCS and MNCS journal indicators here. The purpose of using field-normalized citation indicators is to account for very large differences in citation activity and density across fields, stemming from differences in the referencing behavior and norms for various fields. The operation makes comparison between fields possible, as the score expresses impact relative to the field a given paper is published in, rather than an absolute impact, which may have different meanings across fields. To normalize citation scores, the raw citation count (CS) is divided by the mean citation scores of equivalent papers from the same field. These are papers published in the same year and field, and in this case, with citations counted in the same number of years. This gives us the NCS. Fields are here delimited by an algorithmic approach developed by Waltman and van Eck (2012), where papers are assigned to clusters based on their citing, citation and topical commonalities. These clusters thus define small fields with common referencing cultures, increasing internal consistency when calculating field normalizations. The MNCS journal is simply the mean NCS of all papers published in a given journal in a given year. Like the Journal Impact Factor, the MNCS journal changes from one year to another, and the MNCS journal for a paper is then calculated for the year the paper was published.
Weitzman’s measure, or Δ, is well-defined for density functions. Let f(x) and g(x) be two probability density functions, then:
However, for empirical distributions the solution is not as well-defined. We used the “overlapping” R-package (Pastore, 2018), which divides two empirical density distributions into intervals and calculates the cumulative sum (integral) of minimum values per interval. As both distributions by definition have a cumulative sum of 1, the result is in the range 0 to 1, where 1 implies identical distributions and 0 the complete absence of any overlap. Estimating the overlap empirically heavily depends on the number of bins the distribution is divided into. We tested various bin ranges for our samples and found estimates stabilized around 5,000 bins and upward, and thus used 10,000 bins for the analysis.
Data availability
All final data and analytical scripts are available on GitHub: https://github.com/ipoga/gendcit (copy archived at https://github.com/elifesciences-publications/gendcit).
References
-
Gender differences in research collaborationJournal of Informetrics 7:811–822.https://doi.org/10.1016/j.joi.2013.07.002
-
Are female researchers less cited? A large-scale study of Norwegian scientistsJournal of the American Society for Information Science and Technology 62:628–636.https://doi.org/10.1002/asi.21486
-
Pursuing quality: How search costs and uncertainty magnify gender-based double standards in a multistage evaluation processAdministrative Science Quarterly 62:698–730.https://doi.org/10.1177/0001839217694358
-
BookLifting a Ton of Feathers: A Woman’s Guide for Survivng in the Academic WorldUniversity of Toronto Press.
-
A "ton of feathers": Gender discrimination in academic medical careers and how to manage itJournal of Women's Health 12:1009–1018.https://doi.org/10.1089/154099903322643938
-
BookA theory of limited differencesIn: Zuckerman H, Cole JR, Bruer J, editors. The Outer Circle: Women in the Scientific Community. New York: Norton. pp. 277–310.
-
A MEDLINE categorization algorithmBMC Medical Informatics and Decision Making 6:7.https://doi.org/10.1186/1472-6947-6-7
-
Tweedie: Evaluation of Tweedie exponential family modelsTweedie: Evaluation of Tweedie exponential family models, https://cran.r-project.org/web/packages/tweedie.
-
Series evaluation of Tweedie exponential dispersion model densitiesStatistics and Computing 15:267–280.https://doi.org/10.1007/s11222-005-4070-y
-
Evaluation of Tweedie exponential dispersion model densities by Fourier inversionStatistics and Computing 18:73–86.https://doi.org/10.1007/s11222-007-9039-6
-
Scientific eminencePerspectives on Psychological Science 11:899–904.https://doi.org/10.1177/1745691616663918
-
BookStatistical assumptions as empirical commitmentsIn: Blomberg TG, Cohen S, editors. Law, Punishment, and Social Control: Essays in Honor of Sheldon Messinger. New York, NY: Walter de Gruyter. pp. 235–265.
-
Scaling regression inputs by dividing by two standard deviationsStatistics in Medicine 27:2865–2873.https://doi.org/10.1002/sim.3107
-
Gender API - Determines the gender of a first nameGender API - Determines the gender of a first name, https://gender-api.com.
-
Contemporary psychology and women: A gender analysis of the scientific productionInternational Journal of Psychology 54:135–143.https://doi.org/10.1002/ijop.12433
-
Scientific impact of women in academic surgeryJournal of Surgical Research 148:13–16.https://doi.org/10.1016/j.jss.2008.02.015
-
The "gender gap" in authorship of academic medical literature: A 35-year perspectiveNew England Journal of Medicine 355:281–287.https://doi.org/10.1056/NEJMsa053910
-
Sex differences in attainment of independent funding by career development awardeesAnnals of Internal Medicine 151:804–811.https://doi.org/10.7326/0003-4819-151-11-200912010-00009
-
Prevalence of sexual harassment in academic medicineJAMA Internal Medicine 179:108–111.https://doi.org/10.1001/jamainternmed.2018.4859
-
Gender differences in time spent on parenting and domestic responsibilities by high-achieving young physician-researchersAnnals of Internal Medicine 160:344-353–353.https://doi.org/10.7326/M13-0974
-
Exponential dispersion modelsJournal of the Royal Statistical Society: Series B 49:127–145.
-
Men set their own cites high: Gender and self-citation across fields and over timeSocius: Sociological Research for a Dynamic World 3:.https://doi.org/10.1177/2378023117738903
-
BookThe State of Women in Academic Medicine: The Pipeline and Pathways to Leadership 2013-2014Association of American Medical Colleges.
-
emmeans: Estimated marginal means, aka least-squares meansemmeans: Estimated marginal means, aka least-squares means, https://cran.r-project.org/web/packages/emmeans.
-
The gender gap in early career transitions in the life sciencesResearch Policy 47:1007–1017.https://doi.org/10.1016/j.respol.2018.02.009
-
Measures of sex differences in scientific productivitySocial Forces 71:159–178.https://doi.org/10.1093/sf/71.1.159
-
The gender citation gap in international relationsInternational Organization 67:889–922.https://doi.org/10.1017/S0020818313000209
-
What determines researchers’ scientific impact? A case study of Quebec researchersScience and Public Policy 43:262–274.https://doi.org/10.1093/scipol/scv038
-
Promoting diversity in neuroscienceNature Neuroscience 21:1.https://doi.org/10.1038/s41593-017-0052-6
-
Gender and citation impact in management researchJournal of Informetrics 11:1213–1228.https://doi.org/10.1016/j.joi.2017.09.005
-
Overlapping: Estimation of overlapping in empirical distributionsOverlapping: Estimation of overlapping in empirical distributions, https://cran.r-project.org/web/packages/overlapping.
-
Experiencing the culture of academic medicine: Gender matters, a national studyJournal of General Internal Medicine 28:201–207.https://doi.org/10.1007/s11606-012-2207-1
-
Comparison and benchmark of name-to-gender inference servicesPeerJ Computer Science 4:e156.https://doi.org/10.7717/peerj-cs.156
-
Caveats for using statistical significance tests in research assessmentsJournal of Informetrics 7:50–62.https://doi.org/10.1016/j.joi.2012.08.005
-
Multivariate and propensity score matching software with automated balance optimization: The matching package for RJournal of Statistical Software 42:1–52.
-
The quest for citations: Drivers of article impactJournal of Marketing 71:171–193.https://doi.org/10.1509/jmkg.71.3.171
-
Scouting for talent: Appointment practices of women professors in academic medicineSocial Science & Medicine 72:2033–2040.https://doi.org/10.1016/j.socscimed.2011.04.016
-
The Leiden ranking 2011/2012: Data collection, indicators, and interpretationJournal of the American Society for Information Science and Technology 63:2419–2432.https://doi.org/10.1002/asi.22708
-
A new methodology for constructing a publication-level classification system of scienceJournal of the American Society for Information Science and Technology 63:2378–2392.https://doi.org/10.1002/asi.22748
-
Degrees of difference: Gender segregation of US doctorates by field and program prestigeSociological Science 4:123–150.https://doi.org/10.15195/v4.a6
-
BookMeasures of Overlap of Income Distributions of White and Negro Families in the United StatesWashington, DC: US Bureau of the Census.
Decision letter
-
Peter RodgersSenior and Reviewing Editor; eLife, United Kingdom
-
Willem M OtteReviewer; University Medical Center Utrecht, Netherlands
-
Neven CaplarReviewer; Princeton University
-
Marc LerchenmuellerReviewer; Yale University
-
Rinita DamReviewer; Oxford University, United Kingdom
In the interests of transparency, eLife includes the editorial decision letter and accompanying author responses. A lightly edited version of the letter sent to the authors after peer review is shown, indicating the most substantive concerns; minor comments are not usually included.
Thank you for submitting your article "No discernible gender difference found in global analysis of leading medical authors' per-paper citation impact" for consideration by eLife. Your article has been reviewed by four peer reviewers, and the evaluation has been overseen by the eLife Features Editor (Peter Rodgers). The following individuals involved in review of your submission have agreed to reveal their identity: Willem M Otte (Reviewer #1); Neven Caplar (Reviewer #2); Marc Lerchenmueller (Reviewer #3); Rinita Dam (Reviewer #4).
The reviewers have discussed the reviews with one another and the eLife Features Editor has drafted this decision to help you prepare a revised submission. We hope you will be able to submit the revised version within two months.
SUMMARY
The reviewers were positive about many aspects of the work. For instance, one wrote: "The authors are to be commended for an encompassing piece of work - literature review, data collection and analyses - on a very important and contentious topic in connection to the gender gap in science". However, the reviewers also raised a number of technical point that you will need to address in a revised version. You will also need to better describe the steps in your analysis and to revise the text in a number of places (see below).
LIST OF POINTS THAT NEED TO BE ADDRESSED
# General point
1. A central concern is that the manuscript in its current form convolutes confounding with mechanistic explanations for a potential sex difference in citations in the cross-section. To elaborate, prior work provides evidence that, for example, women relative to men collaborate less internationally and place papers in less prestigious journals. Both phenomena are associated with lower citations, with each then constituting a partial mechanistic explanation for why women may end up receiving less citations.
This differs in meaning from confounding a sex difference in citations that, once adjusting for, should not exist. In other words, if journal prestige adequately proxies the paper-level quality of the manuscripts that get accepted, and women's manuscripts would be accepted less frequently due to genuine quality differentials (versus e.g., bias in the review process), then the authors would have identified a partial explanation for sex differences in citations rather than having rectified a confounded result in prior literature.
Likewise if international collaborations produce higher quality science, as potentially indicated by higher citations, and women collaborate less and by extension produce different quality, on average, then one would expect lower citations to women's work. Overall, considering the matching and various "controls" employed across which men and women conceivably sort differently, one might wonder what residual effect of gender one would expect in the presented regressions?
# Introduction
2. Please provide references for the sentences that start:
- "As of 2013, women constituted 21% of full professors... . " (please add at least one reference)
- "Some studies report. . . " (please one or two references for each of the three classes of findings mentioned in this sentence)
- "Only five studies report. . . " (please add five references)
3. The measure used to quantify presence or absence of gender disparities in medical research, namely the citations, is a very specific proxy. My question would be whether this proxy captures the issue sufficiently. In countries like the Netherlands, for example, tenure track positions and grant scorings are not just based on number of citations or number of publications. The focus is often on the 'five key papers' of a person and on the impact this work has had on scientific and clinical progress. Sometimes a single paper has far more influence on a persons career, visibility and rewards than ten others. I understand that these things are currently not (yet) measurable on a large scale, but please discuss the limitations of using citation counts as a measure of the performance of scientists.
4. The paragraph that starts "However, in one of the most influential multidisciplinary studies on the topic.. . " is too long and should be shortened.
Please also delete the passage "However, others may interpret [... ] and research leaders (Casteevecchi, 2018; Sewell, 2018)." as it is too speculative for a peer-reviewed paper.
5. Please consider citing and briefly commenting upon following recent papers at appropriate places in the manuscript:
Sugimoto, Ahn, Smith, Macaluso and Larivière. 2019. Factors affecting sex-related reporting in medical research: a cross-disciplinary bibliometric analysis. The Lancet. DOI: https://doi.org/10.1016/S0140-6736(18)32995-7)
Gonzalez-Alvarez and Cervera-Crespo. 2019. Psychiatry research and gender diversity: authors, editors and peer reviewers. The Lancet Psychiatry DOI: https://doi.org/10.1016/S2215-0366(19)30039-2
6. The results of your study will be summarized in the abstract, so it is not necessary to summarize them again at the end of the introduction, so please delete the paragraph that starts "We find little evidence... "
# Results
7. Table 1 gives details about the number of papers with female authors etc in the five medical specialities considered in the survey; please consider including similar tables for geographic location and institutional prestige.
8. Please move the information about NCS from the methods section to the start of the paragraph that begins: "Figure 1 displays the.. . ".
This paragraph also needs to include more information about the distributions (eg, number of cases and controls for each sample, standard deviations for each distribution), and to avoid words like trivial and immense. Therefore, please replace the last six sentences in this paragraph with the following (or something similar).
"On average, papers with female first authors are cited X.X% less than papers with male first authors (sample 1. Female first authors: n = XXXX; x-bar = 1.16; s.d. = XXX; x-tilda =0.73. Male first authors: n = XXXX; x-bar = 1.27; s.d. = XXX; x-tilda =0.76. etc): however, the overlap between the two distributions is extensive (Cohen's d = -0.060; Weitzman's Delta = 95.4%; Weitzman, 1970). Papers with female first authors are cited X.X% less than papers with male last authors (sample 2. Female last authors: n = XXXX; x-bar = 1.16; s.d. = XXX; x-tilda =0.72. Male first authors: n = XXXX; x-bar = 1.24; s.d. = XXX; x-tilda =0.76. etc): again, the overlap between the two distributions is extensive (Cohen's d = -0.042; Weitzman's Delta = 95.6%). Papers in which both the first and last authors are female are cited 14.8% less than papers with other gender combinations (sample 3. Female first and last authors: n = XXXX; x-bar = 1.11; s.d. = XXX; x-tilda =0.71. Other combinations: n = XXXX; x-bar = 1.27; s.d. = XXX; x-tilda =0.76. etc): again, the overlap between the two distributions is extensive (Cohen's d = -0.081; Weitzman's Delta = 93.1%). While these results are consistent with previous reports of a citation advantage for papers by male authors, we decided to use regression analyses to explore if there were other explanations for the difference."
Also, for both distributions in each sample, please consider quoting the uncertainty on the mean (derived either under Gaussian approximation as standard deviation/sqrt(N-1), or via bootstrapping).
9. In the caption for figure 1, please explicitly state what the x- and y-axes are (including the units for the y-axis), and what the vertical dashed lines show.
10. In the paragraph that starts "Regression analysis were carried out.. . ", please explain how the field-normalized journal impact is calculated, and how the incidence ratio is calculated, and what incidence ratios of less than one and more than one mean.
11. The discussion of figure 3 in the paragraph that starts "To identify the primary factors.. . " is difficult to read: please move all the values for x-bar, x-tilda etc to the figure caption, and please use consistent terminology throughout (ie MNCS rather than journal impact).
12. A number of the reviewers found figure 3 confusing. Figure 2 suggests that MNCS is the largest contributor to the differences seen in figure 1, but figure 3 suggests that self-citation is the largest contributor. Please clarify.
13. Following on from this, it is not clear how the authors can claim that both self-citations and journal impact independently explain most of the average gender difference. This is possibly only in the case that 1. These two variables are tightly correlated or 2. When taking into account both of these parameters, men should have been receiving less citations than women? Just to expand on this point, I am especially confused with the self-citation statement, given the papers authored by men seems to have much larger tail of the papers with very high citations numbers (Figure 1) and I find it very difficult to believe that only self-citations could explain this high-citation tail of the distribution.
The authors may wish to consult and discuss the following study which, although spanning various fields and productivity levels, suggests self-citation rates to be about 5%-10% of overall citations (which seems similar to the study by King et al, 2017 that the authors cite):
Ioannidis JP, Klavans R, Boyack KW. 2016. Multiple citation indicators and their composite across scientific disciplines. PLOS Biology 14:e1002501.
14. One aspect that, to my mind, clouds the interpretation of the results is the way the authors account for "field". The matching accounts for field via 124 specialties and I understand from the description that the matching has been "exact" (not coarsened or nearest neighbour etc.) such that to be matched, authors had to be located in the same region, institution of like prestige, and same field. Now, the regressions account for field on both the left hand side and the right hand side of the equation through the WoS citation normalization (4K fields). It seems intuitive, that having the WoS normalization on both sides of the equation leads to substantial variance in NCS (your dependent variable) being explained by MNCS (independent variable), leaving little room (besides self-citations) for other covariates.
Related to this, it would be interesting to see the relative impact of MNCS in the regressions when the matching includes the 124 fields and when it does not. Likewise, it would be informative to control for journal through crude fixed effects (i.e., dummies for a specific journal) or journal impact factor tiers (e.g., low, middle, high JIF) in comparison to having the citation information embedded in your journal control variable to see how that influences the coefficient on gender in the regressions.
# Discussion
15. What lends credence to your interpretation of the results is, that you end up observing no tangible, residual effect of gender in your models with all controls despite the fact that the analysis is still in the cross-section and you likely pool men who are, on average, more experienced researchers than women. One might therefore expect men having a citation advantage in the cross-section. The authors might want to include this reflection in their discussion to bolster their interpretation of the findings.
# Methods
16. Based on personal experience with mapping of first and last author data [https://elifesciences.org/articles/34412] I know that the patterns of 'missing data' is not random. Most complete data is available for the most recent publications. The 'lag effect' - as is mentioned in the paper as well - may result in low citations scores for (senior) females just because the entered the field later than their (old) male colleagues. The authors try to mitigate the issue of time with a window approach, but there may still be an effect due to non-random missing data in the first years of the dataset. I would help if the authors could plot the percentages of missing data over time for the four gender classes.
17. The methods section needs to say more about how the following data were obtained and/or calculated:
- Institutional prestige
- NCS
- MNCS
- Self-citations
- International collaboration.
# Data and code
18. It would be very helpful, given the robustness of the analysis and general pipeline structure, if the authors would be willing to share their R code with the meta-research community on a (github) account.
19. Likewise, the authors should make available as much of their data as possible.
# Supplementary material
20. If your manuscript is accepted for publication we will explain how the information in the supplementary material file can be integrated into the paper itself, and how some items can be published as additional files.
[Editors' note: further revisions were requested prior to acceptance, as described below.]
Thank you for submitting the revised version of your article "No tangible gender difference found in global analysis of lead authors' per-paper citation impact in medical research" to eLife. The revised version of your article has been seen by three of the reviewers who reviewed the original version (Willem M Otte; Reviewer #1; Neven Caplar; Reviewer #2; Marc Lerchenmueller; Reviewer #3) and their responses have been largely positive. If you are able to address a small number final comments (see below), we will be able to accept your manuscript for publication.
REVIEWER COMMENTS:
Reviewer #1
I want to compliment the authors with this revised version of their manuscript. The work has significantly improved in terms of explanation, references to recent work by others as well as transparency (github) and deserves sharing with the community. I have no further issues or suggestions.
Reviewer #2
I believe that the authors have significantly improved their manuscript and I believe that I am now able to fully follow and understand how the analysis is conducted. I believe that the manuscript can be accepted in this form. I elaborate below.
I am still a bit surprised the authors choose to emphasize how the difference between the citation counts for men and women is "trivial" - if I were to write a same paper with the same data I would probably point out how the difference between citation counts for men and women, everything else being the same, is highly statistically significant. I would also be tempted to put actual numbers and error estimations in the abstract instead of quite abstract language that is currently there.
Having said that, I believe that authors have considerably improved their manuscript and the description of the analysis is now much clearer. My current objections are mostly stylistic. I see no obviously mistakes or problems in the analysis, and in the reported numbers - as such I am happy to recommend the manuscript for publication.
Note from Features Editor: Addressing the editorial comments below will address the concerns of Reviewer #2.
Reviewer #3
I would like to thank the authors for a thorough revision of the manuscript. I have no remaining substantive comment on the empirical work at this point. That said, please allow me to reiterate a concern I had when reading the initial submission of this work that remains in the revised version of the manuscript.
The authors report an unconditional mean sex difference in citations of 6.5% to 12.6% percent, which is in line with previous work. The authors then construct a set of control variables that allow testing for potential explanations for this gender gap and find, that especially self-citations and the impact of the publishing outlet have explanatory power. I appreciate that the authors have revised language in several locations that originally presented these effects as confounders to e.g., "co-variates". I also commend the authors on a thoughtful discussion of the self-citations (e.g., 4-year citation window, potentially more senior men than women in the cross-section). Still, the results allow for a narrative according to which women may garner fewer citations, at least in part, due to meritocratic dynamics. For example women may publish less frequently in high impact journals, which likely impedes the accumulation of citations, and there is emerging evidence on this dynamic (see Holman et al. 2018 or Lerchenmüller et al. 2018). Of course, it is conceivable that there exists e.g., gender bias in the review process, but until there is robust evidence for that conjecture (of which I am not aware of to date), it may also be that women experience lower citation counts because of a possibly meritocratic dynamic. Likewise, as the authors point out, sex differences in self-citations may stem from men being able to draw on more past work (particularly as the cross-section likely includes more men and the authors acknowledge that).
I would, therefore, encourage the authors to critically reflect about core claims that the manuscript currently makes, including "no tangible gender difference" in the title and "challenge meritocratic explanations" in the abstract. The fact is, that the data indicate an unconditional gender difference in the cross-section, apparently driven by the right hand tail (i.e., ostensible top performers). Although the difference is detected in the cross-section, even an ostensibly "small" difference of 6+% may accumulate to tangible career disadvantages over time (see Merton's work on the Matthew effect) and more longitudinal work is likely needed for more conclusive statements. The here identified mechanisms for this sex difference do not, to my mind, equate to there being no "tangible difference" but instead illuminate where a difference may stem from. Whereas it seems adequate to say that conditional on same quality journal, team size and international collaboration (perhaps as proxies for resources) etc. women produce work that is cited at a similar rate as work by men, it is not clear from the data that the unconditional effect does not exist for meritocratic dynamics. To be clear, I highlight these points with the belief that this manuscript has to offer a valuable contribution to a contentious and important topic, and I also believe that offering the most impartial interpretation may increase the community's recognition of this contribution.
- Holman, L., Stuart-Fox, D., & Hauser, C. E. (2018). The gender gap in science: How long until women are equally represented?. PLoS biology, 16(4), e2004956.
- Merton, R. K. (1968). The Matthew effect in science: The reward and communication systems of science are considered. Science, 159(3810), 56-63.
- Lerchenmüller, C., Lerchenmueller, M. J., & Sorenson, O. (2018). Long-term analysis of sex differences in prestigious authorships in cardiovascular research supported by the National Institutes of Health. Circulation, 137(8), 880-882.
1. Note from Features Editor: Addressing the editorial comments below will address most of the concerns of this Reviewer #3. However, please consider making further revisions to discuss and cite some or all of the papers by Holman et al., Merton, and Lerchenmüller et al.
EDITORIAL COMMENTS:
2. Please consider changing the title to the following, or something similar, to address the concerns of reviewers #2 and #3.
Gender difference in per-paper citation impact is mostly due to differences in self-citation and journal prestige
3. Please consider rewording the abstract as follows to address the concerns of reviewers #2 and #3:
A number of studies have found that scientific papers with women in leading-author positions attract fewer citations than those with men in leading-author positions. Here we report the results of a matched case-control study of 1,269,542 papers in selected areas of medicine published between 2008 and 2014. We find that papers with female authors are cited between 6.5% and 12.6% less than papers with male authors. However, when we adjust for self-citations, number of authors, international collaboration and journal prestige, we found near-identical per-paper citation impact for women and men in first and last author positions, with self-citations and journal prestige accounting for most of the difference. Given the underrepresentation of women in the upper echelons of academic medicine, these results highlight the importance of working to remove to the complex structural and cultural barriers that perpetuate gender inequalities in scientific organizations.
4. Please consider rewording the introduction as follows to address the concerns of reviewers #2 and #3 and to improve the flow of this section:
Over the past four decades, the share of female graduates in medicine has increased from less than 10% to more than 50% in OECD countries, and recent statistics suggest near-parity in the representation of women and men as authors in medical research in Australia, Brazil, Chile, Europe and North America (OECD, 2019; Elsevier, 2017). However, gender inequalities persist in the upper echelons of academic medicine. Moreover, as of 2013, women constituted just 21% of full professors in the United States and just 23% in Europe, with the proportion of women department chairs and deans being lower [OK?] (European Commission, 2016; Lautenberger et al., 2014).
These gender imbalances likely reflect myriad obstacles to women's career progress, including chilly and sometimes hostile work climates (Carr et al., 2003; Jenner et al., 2018; Pololi et al., 2013), bias in recruitment and selection practices (Van den Brink, 2011), societal cultures that still expect a strongly gendered division of domestic labor (Jolly et al., 2014), an underrepresentation of women in last-author positions (González-álvarez and Cervera-Crespo, 2019; Jagsi et al., 2006; Lerchenmueller and Sorenson, 2018), and disparities in research funding (Jagsi et al., 2009; Sege et al., 2015). Given that citation indicators are increasingly being used to inform tenure, hiring and funding decisions in many areas of the medical sciences, any gender bias in citations has the potential to contribute to the perpetuation of these inequalities, so a number of researchers have explored the topic of gender and citations in recent years.
A survey of the literature revealed 22 papers on gender and citations in the medical sciences published between 2006 and 2016 (see supplementary file 1). The study designs, impact measures and statistics used in these papers are too heterogeneous for meta-analytical comparisons, and this literature is also characterized by notable variations in results depending on specialty, country, study design and type of citation indicator (h-index, citations per paper, cumulative citations, m-quotient and journal impact factor). Some studies report an average male-citation advantage (e.g., Larivière et al., 2011; Nielsen, 2016), whereas others do not observe any notable gender difference (e.g., Mirnezami et al., 2016; Pagel and Hudetz, 2011). Existing articles are in most cases based on convenience samples and limit their focus to single specialties or sub-specialties (16 out of 22), and the literature is characterized by a North American, bias with only five studies focusing on countries outside the US and Canada. Moreover, most articles (14 out of 22) base their gender comparisons on relatively small samples, and very few adjust for relevant covariates that may contribute to explain average gender differences, such as collaboration patterns, numbers of authors per paper, self-citations and institutional prestige. Furthermore, only six of the papers report direct comparisons of the average number of citations per paper for male and female authors (Housri et al., 2008; Larivière et al., 2011; Mirnezami et al., 2016; Nielsen, 2016; Pagel and Hudetz, 2015; Pagel and Hudetz; 2011).
Researchers have also studied gender and bias in fields other than medicine, and again these studies are characterized by ambiguous results that vary by geographical focus, time-period and discipline. Some report differences in favor of male authors (Aksnes et al., 2011; Larivière et al., 2013; Caplar et al., 2017; Eagly and Miller, 2016; Maliniak et al., 2013), some report smaller differences in favour of female authors (Borrego et al., 2010; Long, 1992; van Arensbergen et al., 2012), and some report no discernable gender difference (Nielsen, 2017; Slyder et al., 2011; Symonds et al., 2006). [Query: Is it correct that Nielsen, 2016 finds a male-citation advantage, where Nielsen, 2017 finds no gender difference?]
Here we report the results of a comprehensive, global analysis of possible gender variations in the per-paper citation impact of medical researchers. We analyzed 1,269,542 papers on disease-specific medical research published between 2008 and 2014 (n=1,269,542). To reduce confounding and ensure balanced case-control groups, three matching covariates (institutional prestige, geographic location and medical specialty) were used to generate three datasets: sample 1 had female first authors as the case and male first authors as the control (n=1,018,665); sample 2 had female last authors as the case and male last authors as the control (n=653,233); and in sample 3, pairs of female first and last authors constituted the case group and all other author combinations were included in the control group (n=368,374). The outcome variable was field-normalized citations per paper, and regression analyzes were used to explore the influence of additional co-varying factors (self-citations, number of authors, international collaboration and journal prestige) on differences in per-paper citation impact (see Methods). Given the large sample size, global scope, and matched design, our study is less vulnerable to biases resulting from sample-specific variance, confounders and selection than previous studies.
5. The word "trivial" appears four times in your manuscript. Please reword (by, for example, changing trivial to small or a similar word) to address the concerns of reviewers #2 and #3. The first two sentences of the conclusion could also be reworded as follows:
In conclusion, our results demonstrate that, adjusting for co-varying factors, men and women in first and last author positions are cited at similar rates.
6. In general the manuscript refers to "per-paper citation impact" or just "citation impact". However, it sometimes uses other phrases, such as "citation score" or "citation rate". If these phrases all mean different things, that is fine. However, if any two of them mean the same thing, please use just one of them.
https://doi.org/10.7554/eLife.45374.026Author response
# General point
A central concern is that the manuscript in its current form convolutes confounding with mechanistic explanations for a potential sex difference in citations in the cross-section. To elaborate, prior work provides evidence that, for example, women relative to men collaborate less internationally and place papers in less prestigious journals. Both phenomena are associated with lower citations, with each then constituting a partial mechanistic explanation for why women may end up receiving less citations.
This differs in meaning from confounding a sex difference in citations that, once adjusting for, should not exist. In other words, if journal prestige adequately proxies the paper-level quality of the manuscripts that get accepted, and women's manuscripts would be accepted less frequently due to genuine quality differentials (versus e.g., bias in the review process), then the authors would have identified a partial explanation for sex differences in citations rather than having rectified a confounded result in prior literature.
Likewise if international collaborations produce higher quality science, as potentially indicated by higher citations, and women collaborate less and by extension produce different quality, on average, then one would expect lower citations to women's work. Overall, considering the matching and various "controls" employed across which men and women conceivably sort differently, one might wonder what residual effect of gender one would expect in the presented regressions?
These are excellent points. We have revised the paper to make this distinction clear and removed the term “confounding” where it is unwarranted.
# Introduction
2. Please provide references for the sentences that start:
- "As of 2013, women constituted 21% of full professors... . " (please add at least one reference)
- "Some studies report. . . " (please one or two references for each of the three classes of findings mentioned in this sentence)
- "Only five studies report. . . " (please add five references)
The requested references have been added in the revised manuscript.
3. The measure used to quantify presence or absence of gender disparities in medical research, namely the citations, is a very specific proxy. My question would be whether this proxy captures the issue sufficiently. In countries like the Netherlands, for example, tenure track positions and grant scorings are not just based on number of citations or number of publications. The focus is often on the 'five key papers' of a person and on the impact this work has had on scientific and clinical progress. Sometimes a single paper has far more influence on a persons career, visibility and rewards than ten others. I understand that these things are currently not (yet) measurable on a large scale, but please discuss the limitations of using citation counts as a measure of the performance of scientists.
This is a certainly a relevant perspective, and we have included a reflection on this matter in the Discussion section of the revised manuscript.
4. The paragraph that starts "However, in one of the most influential multidisciplinary studies on the topic.. . " is too long and should be shortened.
Please also delete the passage "However, others may interpret [... ] and research leaders (Casteevecchi, 2018; Sewell, 2018)." as it is too speculative for a peer-reviewed paper.
The paragraph has been shortened, and we have removed the speculative passage in the introduction.
5. Please consider citing and briefly commenting upon following recent papers at appropriate places in the manuscript:
Sugimoto, Ahn, Smith, Macaluso and Larivière. 2019. Factors affecting sex-related reporting in medical research: a cross-disciplinary bibliometric analysis. The Lancet. DOI: https://doi.org/10.1016/S0140-6736(18)32995-7)
Gonzalez-Alvarez and Cervera-Crespo. 2019. Psychiatry research and gender diversity: authors, editors and peer reviewers. The Lancet Psychiatry
DOI: https://doi.org/10.1016/S2215-0366(19)30039-2
In the revised manuscript, we cite and briefly comment upon each of these references.
6. The results of your study will be summarized in the abstract, so it is not necessary to summarize them again at the end of the introduction, so please delete the paragraph that starts "We find little evidence... "
Good point. We have removed this section from the introduction.
# Results
7. Table 1 gives details about the number of papers with female authors etc in the five medical specialities considered in the survey; please consider including similar tables for geographic location and institutional prestige.
Thank you for this suggestion. Table 1 now includes information about geographic location and institutional prestige as well.
8. Please move the information about NCS from the methods section to the start of the paragraph that begins: "Figure 1 displays the.. . ".
This paragraph also needs to include more information about the distributions (eg, number of cases and controls for each sample, standard deviations for each distribution), and to avoid words like trivial and immense. Therefore, please replace the last six sentences in this paragraph with the following (or something similar).
"On average, papers with female first authors are cited X.X% less than papers with male first authors (sample 1. Female first authors: n = XXXX; x-bar = 1.16; s.d. = XXX; x-tilda =0.73. Male first authors: n = XXXX; x-bar = 1.27; s.d. = XXX; x-tilda =0.76. etc): however, the overlap between the two distributions is extensive (Cohen's d = -0.060; Weitzman's Delta = 95.4%; Weitzman, 1970). Papers with female first authors are cited X.X% less than papers with male last authors (sample 2. Female last authors: n = XXXX; x-bar = 1.16; s.d. = XXX; x-tilda =0.72. Male first authors: n = XXXX; x-bar = 1.24; s.d. = XXX; x-tilda =0.76. etc): again, the overlap between the two distributions is extensive (Cohen's d = -0.042; Weitzman's Delta = 95.6%). Papers in which both the first and last authors are female are cited 14.8% less than papers with other gender combinations (sample 3. Female first and last authors: n = XXXX; x-bar = 1.11; s.d. = XXX; x-tilda =0.71. Other combinations: n = XXXX; x-bar = 1.27; s.d. = XXX; x-tilda =0.76. etc): again, the overlap between the two distributions is extensive (Cohen's d = -0.081; Weitzman's Delta = 93.1%). While these results are consistent with previous reports of a citation advantage for papers by male authors, we decided to use regression analyses to explore if there were other explanations for the difference."
Also, for both distributions in each sample, please consider quoting the uncertainty on the mean (derived either under Gaussian approximation as standard deviation/sqrt(N-1), or via bootstrapping).
These are great points. The manuscript has been revised in accordance with each of the requests. For all distributions, the absolute uncertainty of the mean is between 0.001 and 0.005. This is reported in the first paragraph, p. 4.
9. In the caption for figure 1, please explicitly state what the x- and y-axes are (including the units for the y-axis), and what the vertical dashed lines show.
The caption for Figure 1 has been revised in accordance with these requests.
10. In the paragraph that starts "Regression analysis were carried out.. . ", please explain how the field-normalized journal impact is calculated, and how the incidence ratio is calculated, and what incidence ratios of less than one and more than one mean.
These are great suggestions. This paragraph now includes a specification on how NCS and MNCS journal are calculated. We also specify how the exponentiated coefficients in the Tweedie should be interpreted. In addition, we have computed estimated marginal means to allow for a more intuitive interpretation of the outcomes of the Tweedie regressions. Please note that that the numeric regression inputs have been rescaled by dividing by two standard deviations to allow for meaningful comparison of binary and numeric variables. We have also expanded the field-normalization explanation in the methods section.
11. The discussion of figure 3 in the paragraph that starts "To identify the primary factors.. . " is difficult to read: please move all the values for x-bar, x-tilda etc to the figure caption, and please use consistent terminology throughout (ie MNCS rather than journal impact).
This is a good point. This information is now reported in Table 2. Please note that we have revised this part of the manuscript considerably to accommodate other reviewer concerns.
12. A number of the reviewers found figure 3 confusing. Figure 2 suggests that MNCS is the largest contributor to the differences seen in figure 1, but figure 3 suggests that self-citation is the largest contributor. Please clarify.
13. Following on from this, it is not clear how the authors can claim that both self-citations and journal impact independently explain most of the average gender difference. This is possibly only in the case that 1. These two variables are tightly correlated or 2. When taking into account both of these parameters, men should have been receiving less citations than women? Just to expand on this point, I am especially confused with the self-citation statement, given the papers authored by men seems to have much larger tail of the papers with very high citations numbers (Figure 1) and I find it very difficult to believe that only self-citations could explain this high-citation tail of the distribution.
The authors may wish to consult and discuss the following study which, although spanning various fields and productivity levels, suggests self-citation rates to be about 5%-10% of overall citations (which seems similar to the study by King et al, 2017 that the authors cite):
Ioannidis JP, Klavans R, Boyack KW. 2016. Multiple citation indicators and their composite across scientific disciplines. PLOS Biology 14:e1002501.
These are very important points. We have removed Figure 3 from the manuscript. In the revised version, we use logistic regression to examine associations between the covariates and the case variables (Figure 4). In figure 5, we examine the average proportion of per-paper self-citations plotted by NCS-based quantiles (5-percentile bins) in samples 1, 2, 3. This figure indicates that self-citations comprise approximately 15% of the per-paper citations in the top 5% most cited papers. This implies that at least part of the gender variation observed on the right side of the curves in Figure 1 may be attributable to average gender differences in per-paper self-citation rates. Note here, that our citation indicators are calculated with a four-year window, which may contribute to explain the relatively large proportion of self-citations in the samples.
The revised manuscript also includes a more detailed analysis of variations in the representation of case papers and control papers plotted by MNCS journal-based 5-percentile bins – from low impact to high impact journals. Figure 6 indicates an underrepresentation of case papers (with women in leading author positions) in the top-5 percent journals with the highest MNCS-journal score.
14. One aspect that, to my mind, clouds the interpretation of the results is the way the authors account for "field". The matching accounts for field via 124 specialties and I understand from the description that the matching has been "exact" (not coarsened or nearest neighbour etc.) such that to be matched, authors had to be located in the same region, institution of like prestige, and same field. Now, the regressions account for field on both the left hand side and the right hand side of the equation through the WoS citation normalization (4K fields). It seems intuitive, that having the WoS normalization on both sides of the equation leads to substantial variance in NCS (your dependent variable) being explained by MNCS (independent variable), leaving little room (besides self-citations) for other covariates.
Related to this, it would be interesting to see the relative impact of MNCS in the regressions when the matching includes the 124 fields and when it does not. Likewise, it would be informative to control for journal through crude fixed effects (i.e., dummies for a specific journal) or journal impact factor tiers (e.g., low, middle, high JIF) in comparison to having the citation information embedded in your journal control variable to see how that influences the coefficient on gender in the regressions.
In the original manuscript, we also ran the Tweedie regressions with raw, un-normalized citations scores as the outcome variables (as a robustness check) with qualitatively similar results. This suggests that double-normalization is not a problematic issue in this case. In the revised manuscript, we have included three robustness checks to examine the sensitivity of the results to alternative model and sample specifications. First, we ran negative binomial regressions with raw per-paper citation scores (with a four-year citation window) (CS) as the outcome variable in Samples 1, 2 and 3. Next, we ran Tweedie regressions with NCS as outcome variable based on the full, un-matched data set. Finally, we ran Tweedie regressions with dummy variables for different levels of journal prestige as suggested by one of the reviewers.
We should perhaps also stress that fields in field-normalizations and medical specialties are two very different things. We have tried to stick to the precise use of these terms and have added additional explanation about how field-normalizations are performed.
# Discussion
15. What lends credence to your interpretation of the results is, that you end up observing no tangible, residual effect of gender in your models with all controls despite the fact that the analysis is still in the cross-section and you likely pool men who are, on average, more experienced researchers than women. One might therefore expect men having a citation advantage in the cross-section. The authors might want to include this reflection in their discussion to bolster their interpretation of the findings.
We agree that this is an important factor. We now reflect on this in the Discussion section.
# Methods
16. Based on personal experience with mapping of first and last author data [https://elifesciences.org/articles/34412] I know that the patterns of 'missing data' is not random. Most complete data is available for the most recent publications. The 'lag effect' - as is mentioned in the paper as well - may result in low citations scores for (senior) females just because the entered the field later than their (old) male colleagues. The authors try to mitigate the issue of time with a window approach, but there may still be an effect due to non-random missing data in the first years of the dataset. I would help if the authors could plot the percentages of missing data over time for the four gender classes.
This is a relevant concern, which we have looked into. The proportion of papers for which we can reliably determine the gender is stable throughout the period, regardless of whether we compare it to the population (pubmed) or the matched WoS sample. We have added a figure showing this in the supplementary materials. There is however a slightly smaller proportion in 2008, the earliest year, suggesting this would be worse going further back in time.
17. The methods section needs to say more about how the following data were obtained and/or calculated:
- Institutional prestige
- NCS
- MNCS
- Self-citations
- International collaboration.
We have added additional explanations of the calculation and acquisition of these variables, where they are used and more extensively in the methods section.
# Data and code
18. It would be very helpful, given the robustness of the analysis and general pipeline structure, if the authors would be willing to share their R code with the meta-research community on a (github) account.
19. Likewise, the authors should make available as much of their data as possible.
We completely agree in this practice and had planned to do something along this line. We have removed elements of our data (Web of Science identification numbers) that would prevent us from sharing the data, and have added all analytical files and cleaned, prepared datasets to a GitHub account. We are contractually prohibited from sharing the scripts that generate these datasets and the underlying raw data, as these are the property of Clarivate Analytics.
Data and code are available here: https://github.com/ipoga/gendcit
Compiled supplementary material notebook is available here: https://ipoga.github.io/gendcit/
# Supplementary material
20. If your manuscript is accepted for publication we will explain how the information in the supplementary material file can be integrated into the paper itself, and how some items can be published as additional files.
As part of the above code sharing, we have also created a GitHub page containing a generated report with all the information from the supplementary materials. We hope this approach is useful and acceptable.
[Editors' note: further revisions were requested prior to acceptance, as described below.]
We have very carefully considered all the suggestions and chosen to adopt almost all of them. In the following, we respond directly to the comments with the changes we have made. During this process we have also made minor aesthetic revisions of the figures (purely to increase readability) and language – but no data or meaning has been changed apart from what has been suggested or as direct consequence of suggested changes.
Reviewer #1
I want to compliment the authors with this revised version of their manuscript. The work has significantly improved in terms of explanation, references to recent work by others as well as transparency (github) and deserves sharing with the community. I have no further issues or suggestions.
Thank you for your kind review, and for previous work on the manuscript. We greatly appreciate it.
Reviewer #2
I believe that the authors have significantly improved their manuscript and I believe that I am now able to fully follow and understand how the analysis is conducted. I believe that the manuscript can be accepted in this form. I elaborate below.
I am still a bit surprised the authors choose to emphasize how the difference between the citation counts for men and women is ``trivial' - if I were to write a same paper with the same data I would probably point out how the difference between citation counts for men and women, everything else being the same, is highly statistically significant. I would also be tempted to put actual numbers and error estimations in the abstract instead of quite abstract language that is currently there.
Having said that, I believe that authors have considerably improved their manuscript and the description of the analysis is now much clearer. My current objections are mostly stylistic. I see no obviously mistakes or problems in the analysis, and in the reported numbers - as such I am happy to recommend the manuscript for publication.
Note from Features Editor: Addressing the editorial comments below will address the concerns of Reviewer #2.
Thank you for your comments, we are happy that the revisions have improved the manuscript. We have reconsidered the use of the word trivial in regard to effect sizes, and while it is essentially stylistic, we have changed it to “very small”. Originally, we aligned our interpretation with the rules of thumb outlined by Jacob Cohen where a standardized mean difference of (Cohen’s d) <.2 (corresponding to an odds ratio below 1.4) would be seen as trivial in size, not big enough to register as a small effect. However, as these rules of thumb are indeed contextual, and the term “trivial” may have problematic connotations in our context, we have decided to change it to “very small”.
Regarding the second suggestion (about statistical significance), in line with the American Statistical Association and others (see Wasserstein, Schirm & Lazar, 2019). We generally do not approve of the term “statistical significance” and its implications. We endorse an estimation approach. In the present study, we provide confidence intervals, although they have their limitations too. Nonetheless, with the sample sizes we work with, estimation becomes very precise and therefore the confidence limits become tiny.
Wasserstein, R. L., Schirm, A. L., & Lazar, N. A. (2019). Moving to a world beyond ‘p<0.05’. The American Statistician, 73(supol 1), 1-19
Reviewer #3
I would like to thank the authors for a thorough revision of the manuscript. I have no remaining substantive comment on the empirical work at this point. That said, please allow me to reiterate a concern I had when reading the initial submission of this work that remains in the revised version of the manuscript.
The authors report an unconditional mean sex difference in citations of 6.5% to 12.6% percent, which is in line with previous work. The authors then construct a set of control variables that allow testing for potential explanations for this gender gap and find, that especially self-citations and the impact of the publishing outlet have explanatory power. I appreciate that the authors have revised language in several locations that originally presented these effects as confounders to e.g., "co-variates". I also commend the authors on a thoughtful discussion of the self-citations (e.g., 4-year citation window, potentially more senior men than women in the cross-section). Still, the results allow for a narrative according to which women may garner fewer citations, at least in part, due to meritocratic dynamics. For example women may publish less frequently in high impact journals, which likely impedes the accumulation of citations, and there is emerging evidence on this dynamic (see Holman et al. 2018 or Lerchenmüller et al. 2018). Of course, it is conceivable that there exists e.g., gender bias in the review process, but until there is robust evidence for that conjecture (of which I am not aware of to date), it may also be that women experience lower citation counts because of a possibly meritocratic dynamic. Likewise, as the authors point out, sex differences in self-citations may stem from men being able to draw on more past work (particularly as the cross-section likely includes more men and the authors acknowledge that).
I would, therefore, encourage the authors to critically reflect about core claims that the manuscript currently makes, including "no tangible gender difference" in the title and "challenge meritocratic explanations" in the abstract. The fact is, that the data indicate an unconditional gender difference in the cross-section, apparently driven by the right hand tail (i.e., ostensible top performers). Although the difference is detected in the cross-section, even an ostensibly "small" difference of 6+% may accumulate to tangible career disadvantages over time (see Merton's work on the Matthew effect) and more longitudinal work is likely needed for more conclusive statements. The here identified mechanisms for this sex difference do not, to my mind, equate to there being no "tangible difference" but instead illuminate where a difference may stem from. Whereas it seems adequate to say that conditional on same quality journal, team size and international collaboration (perhaps as proxies for resources) etc. women produce work that is cited at a similar rate as work by men, it is not clear from the data that the unconditional effect does not exist for meritocratic dynamics. To be clear, I highlight these points with the belief that this manuscript has to offer a valuable contribution to a contentious and important topic, and I also believe that offering the most impartial interpretation may increase the community's recognition of this contribution.
- Holman, L., Stuart-Fox, D., & Hauser, C. E. (2018). The gender gap in science: How long until women are equally represented?. PLoS biology, 16(4), e2004956.
- Merton, R. K. (1968). The Matthew effect in science: The reward and communication systems of science are considered. Science, 159(3810), 56-63.
- Lerchenmüller, C., Lerchenmueller, M. J., & Sorenson, O. (2018). Long-term analysis of sex differences in prestigious authorships in cardiovascular research supported by the National Institutes of Health. Circulation, 137(8), 880-882.
Thank you for your insightful and very important comment. We want to emphasize that we acknowledge the unconditional gender differences in mean citation impact, and the potential harm this can cause for the individual researcher. However, noise in the underlying data (e.g. from unmatched citations) also means that there is little practical significance of the unconditional differences, when they are not larger than observed, although the sample size deceptively leads to statistical significance.
We also think it is valuable to point out that the observed, unconditional differences are not due to women deliberately being cited less (regardless of interpretation), but rather that it is explained by other factors, such as research area and outlet. Our emphasis is therefore also on the distributions and their overlap rather than the differences in means – meaning there are many more men and women who are the same than who are not.
We especially appreciated your final comment, which has led us to reconsider a number of phrasings, among which is a rephrasing of “trivial” to “very small”, for effects sizes.
1. Note from Features Editor: Addressing the editorial comments below will address most of the concerns of this Reviewer #3. However, please consider making further revisions to discuss and cite some or all of the papers by Holman et al., Merton, and Lerchenmüller et al.
We have included Lerchenmüller et al. We have not included Merton, as we believe our reference to Cole & Singer is more on point. We were not able to find a good location to include Holman et al. Other revisions are commented below.
EDITORIAL COMMENTS:
2. Please consider changing the title to the following, or something similar, to address the concerns of reviewers #2 and #3.
Gender difference in per-paper citation impact is mostly due to differences in self-citation and journal prestige
We have revised the title in line with, but not identical to, the suggested title.
3. Please consider rewording the abstract as follows to address the concerns of reviewers #2 and #3:
A number of studies have found that scientific papers with women in leading-author positions attract fewer citations than those with men in leading-author positions. Here we report the results of a matched case-control study of 1,269,542 papers in selected areas of medicine published between 2008 and 2014. We find that papers with female authors are cited between 6.5% and 12.6% less than papers with male authors. However, when we adjust for self-citations, number of authors, international collaboration and journal prestige, we found near-identical per-paper citation impact for women and men in first and last author positions, with self-citations and journal prestige accounting for most of the difference. Given the underrepresentation of women in the upper echelons of academic medicine, these results highlight the importance of working to remove to the complex structural and cultural barriers that perpetuate gender inequalities in scientific organizations.
We have revised the abstract to this version, albeit with minor modifications.
4. Please consider rewording the introduction as follows to address the concerns of reviewers #2 and #3 and to improve the flow of this section:
Over the past four decades, the share of female graduates in medicine has increased from less than 10% to more than 50% in OECD countries, and recent statistics suggest near-parity in the representation of women and men as authors in medical research in Australia, Brazil, Chile, Europe and North America (OECD, 2019; Elsevier, 2017). However, gender inequalities persist in the upper echelons of academic medicine. Moreover, as of 2013, women constituted just 21% of full professors in the United States and just 23% in Europe, with the proportion of women department chairs and deans being lower [OK?] (European Commission, 2016; Lautenberger et al., 2014).
These gender imbalances likely reflect myriad obstacles to women's career progress, including chilly and sometimes hostile work climates (Carr et al., 2003; Jenner et al., 2018; Pololi et al., 2013), bias in recruitment and selection practices (Van den Brink, 2011), societal cultures that still expect a strongly gendered division of domestic labor (Jolly et al., 2014), an underrepresentation of women in last-author positions (González-álvarez and Cervera-Crespo, 2019; Jagsi et al., 2006; Lerchenmueller and Sorenson, 2018), and disparities in research funding (Jagsi et al., 2009; Sege et al., 2015). Given that citation indicators are increasingly being used to inform tenure, hiring and funding decisions in many areas of the medical sciences, any gender bias in citations has the potential to contribute to the perpetuation of these inequalities, so a number of researchers have explored the topic of gender and citations in recent years.
A survey of the literature revealed 22 papers on gender and citations in the medical sciences published between 2006 and 2016 (see supplementary file 1). The study designs, impact measures and statistics used in these papers are too heterogeneous for meta-analytical comparisons, and this literature is also characterized by notable variations in results depending on specialty, country, study design and type of citation indicator (h-index, citations per paper, cumulative citations, m-quotient and journal impact factor). Some studies report an average male-citation advantage (e.g., Larivière et al., 2011; Nielsen, 2016), whereas others do not observe any notable gender difference (e.g., Mirnezami et al., 2016; Pagel and Hudetz, 2011). Existing articles are in most cases based on convenience samples and limit their focus to single specialties or sub-specialties (16 out of 22), and the literature is characterized by a North American, bias with only five studies focusing on countries outside the US and Canada. Moreover, most articles (14 out of 22) base their gender comparisons on relatively small samples, and very few adjust for relevant covariates that may contribute to explain average gender differences, such as collaboration patterns, numbers of authors per paper, self-citations and institutional prestige. Furthermore, only six of the papers report direct comparisons of the average number of citations per paper for male and female authors (Housri et al., 2008; Larivière et al., 2011; Mirnezami et al., 2016; Nielsen, 2016; Pagel and Hudetz, 2015; Pagel and Hudetz; 2011).
Researchers have also studied gender and bias in fields other than medicine, and again these studies are characterized by ambiguous results that vary by geographical focus, time-period and discipline. Some report differences in favor of male authors (Aksnes et al., 2011; Larivière et al., 2013; Caplar et al., 2017; Eagly and Miller, 2016; Maliniak et al., 2013), some report smaller differences in favour of female authors (Borrego et al., 2010; Long, 1992; van Arensbergen et al., 2012), and some report no discernable gender difference (Nielsen, 2017; Slyder et al., 2011; Symonds et al., 2006). [Query: Is it correct that Nielsen, 2016 finds a male-citation advantage, where Nielsen, 2017 finds no gender difference?]
Here we report the results of a comprehensive, global analysis of possible gender variations in the per-paper citation impact of medical researchers. We analyzed 1,269,542 papers on disease-specific medical research published between 2008 and 2014 (n=1,269,542). To reduce confounding and ensure balanced case-control groups, three matching covariates (institutional prestige, geographic location and medical specialty) were used to generate three datasets: sample 1 had female first authors as the case and male first authors as the control (n=1,018,665); sample 2 had female last authors as the case and male last authors as the control (n=653,233); and in sample 3, pairs of female first and last authors constituted the case group and all other author combinations were included in the control group (n=368,374). The outcome variable was field-normalized citations per paper, and regression analyzes were used to explore the influence of additional co-varying factors (self-citations, number of authors, international collaboration and journal prestige) on differences in per-paper citation impact (see Methods). Given the large sample size, global scope, and matched design, our study is less vulnerable to biases resulting from sample-specific variance, confounders and selection than previous studies.
We are very grateful for the thorough help provided here. We have included the majority of the suggested changes and agree that the flow has improved as a result. The proposed revisions involved deleting a paragraph, which could be considered a more confrontational paragraph than the rest. Rather than deleting this paragraph we have rewritten it to be less confrontational. See page 2 line 35 with simple markup or mid-page 3 with full markup.
5. The word "trivial" appears four times in your manuscript. Please reword (by, for example, changing trivial to small or a similar word) to address the concerns of reviewers #2 and #3. The first two sentences of the conclusion could also be reworded as follows:
In conclusion, our results demonstrate that, adjusting for co-varying factors, men and women in first and last author positions are cited at similar rates.
We have changed “trivial” and some occurrences of “marginal” to the probably more fitting “very small”. We have also reworded the line in question.
6. In general the manuscript refers to "per-paper citation impact" or just "citation impact". However, it sometimes uses other phrases, such as "citation score" or "citation rate". If these phrases all mean different things, that is fine. However, if any two of them mean the same thing, please use just one of them.
There are distinct differences in the interpretation of these terms. Impact is the general term, while score is used for specific indicator calculations. There were cases where “rates” was used in a less optimal fashion – these have now been corrected, and the remaining occurrences are correct.
https://doi.org/10.7554/eLife.45374.027Article and author information
Author details
Funding
Uddannelses- og Forskningsministeriet (6183-00001B)
- Jesper Wiborg Schneider
Aarhus University Research Foundation (AUFF-2018-7-5)
- Mathias Wullum Nielsen
The funders had no role in study design, data collection and interpretation, or the decision to submit the work for publication.
Publication history
- Received:
- Accepted:
- Accepted Manuscript published:
- Version of Record published:
Copyright
© 2019, Andersen et al.
This article is distributed under the terms of the Creative Commons Attribution License, which permits unrestricted use and redistribution provided that the original author and source are credited.
Metrics
-
- 5,107
- views
-
- 343
- downloads
-
- 64
- citations
Views, downloads and citations are aggregated across all versions of this paper published by eLife.
Download links
Downloads (link to download the article as PDF)
Open citations (links to open the citations from this article in various online reference manager services)
Cite this article (links to download the citations from this article in formats compatible with various reference manager tools)
Further reading
-
The study of science itself is a growing field of research.