Synaptic and circuit mechanisms prevent detrimentally precise correlation in the developing mammalian visual system
Figures
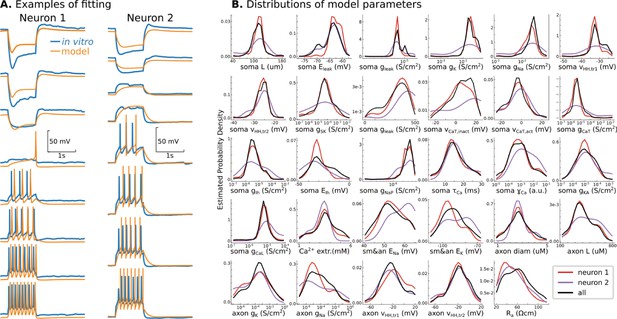
Conductance-based model of P7 TC neurons.
(A) Randomly selected examples for two recorded neurons in the database. (B) Probability density distributions of all 29 model parameters, obtained as Gaussian estimator over all models in the database. Note that EMOs use a logarithmic scale for some parameters, and therefore, probability density distributions are also estimated in the logarithmic scale. Black lines are PDFs for all models in the database, and color lines indicate PDFs for models fitted to recorded neurons shown in A.
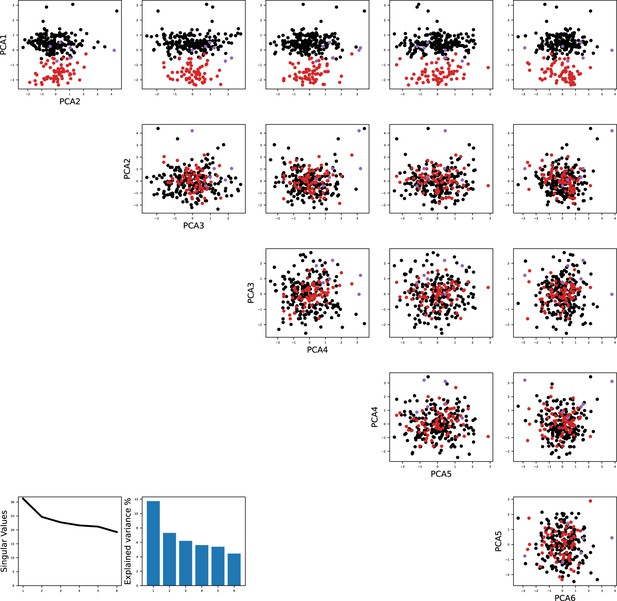
Principal component analysis shows the separation of models fitted to the same recorded neurons as in Figure 1.
The color code is the same as in Figure 1B in the main text.
-
Figure 1—figure supplement 1—source data 1
Data base with 286 models of TC neurons at P7 (available on Zenodo).
- https://cdn.elifesciences.org/articles/84333/elife-84333-fig1-figsupp1-data1-v1.zip
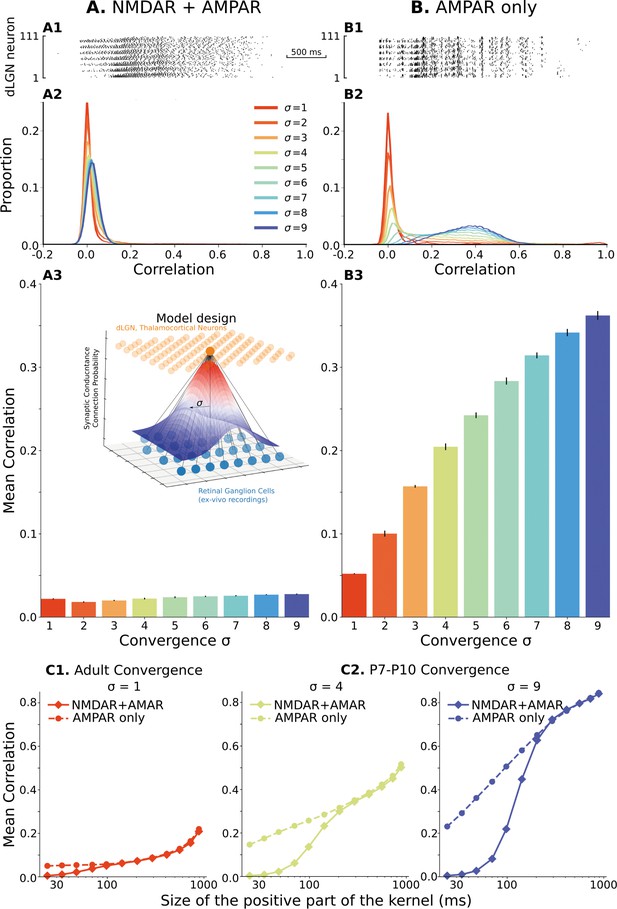
The model of the dLGN network at P7-P10 activated by spikes of rGC recorded ex vivo.
(A) Retinogeniculate synapses have both NMDA and AMPA currents, with NMDA dominance. (B) The same models with NMDA currents disabled. A1 and B1 are examples of two models () responding to the same retinal wave. A2 and B2 are mean distributions of pairwise spike correlation for different convergence factors (). A3 and B3 mean and standard deviation of network-wise average pairwise spike correlation for ten network models for each type of the synaptic drive and convergence factor (). (C) A mean network correlation depends on the timescales of the correlation. Dependence on the timescale is shown for adult convergence (, C1) and two estimates of P7-P10 convergence (C2): 10 inputs per TC neuron (, left) and 20 inputs per TC neuron (, right). The horizontal axis is the maximal between two spikes, which results in a positive correlation for a given kernel size. The insert schematically shows the model design. For all C plots, mean is computed over ten independently generated and initialized network models.
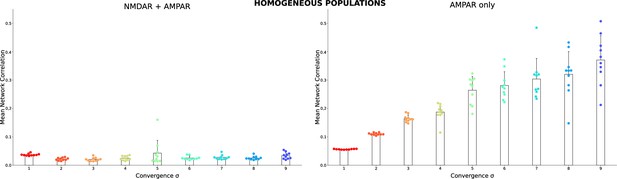
The same as in Figure 2A3 and B3 but for homogeneous populations.
Each dot shows the result for a single homogeneous network model with the same connectivity as in Figure 2 insert, but all TC neurons are the same. The TC neuron model was randomly selected from the database for each network.
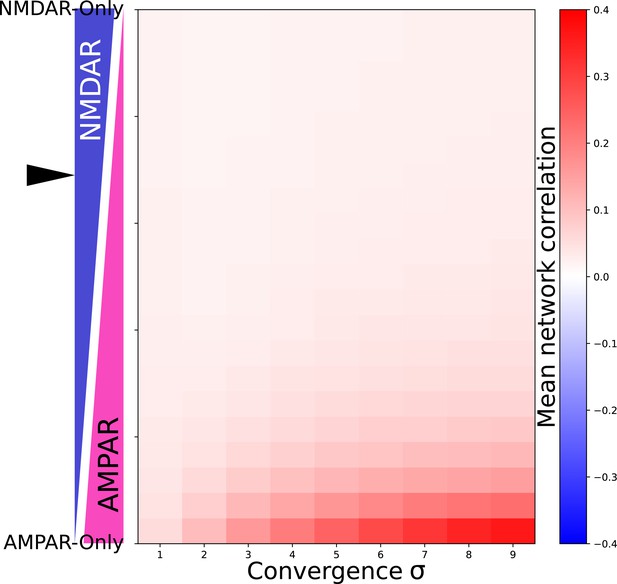
Network correlation dependes on proportion of NMDAR and AMPAR currents and convergance.
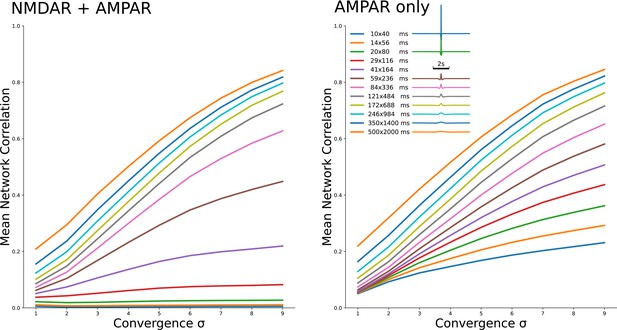
Two families of curves which show the same dependeces as Figure 2A3 and B3 for 12 different kirnels shown in legend.
The family on the left is for NMDAR and AMPAR currents, with NMDAR dominance, while the family on the right is for AMPAR currents only.
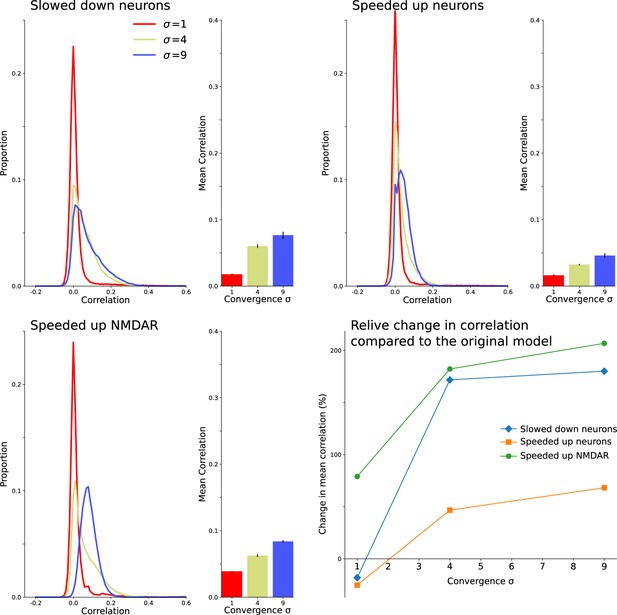
Decorrelation results from interactions between intrinsic neuron dynamics and slow NMDAR current.
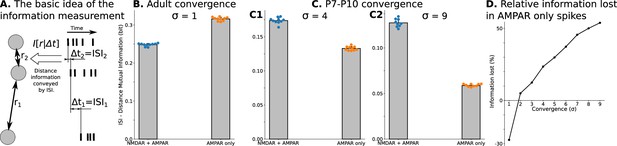
Spatial information encoded in interspike/interburst intervals (ISI) of TC neurons in the dLGN model.
For B and all C plots, mean and standard deviation is computed over ten independently generated and initialized network models. (A) Mutual information as a quantitative measure for predictability of distances from observed ISI . (B) Mutual information in a model with adult-like convergence (). (C) The same as in B but for two estimates of P7-P10 convergence: 10 inputs per TC neuron (, C1) and 20 inputs per TC neuron (, C2). (D) Dependence of information lost/gained in spikes of models with AMPA receptors only compared to models with NMDA + AMPA mixture of receptors on the convergence of rGC inputs to a single TC neuron (). For B, and all C plots, mean and standard deviation is computed over ten independently generated and initialized network models.
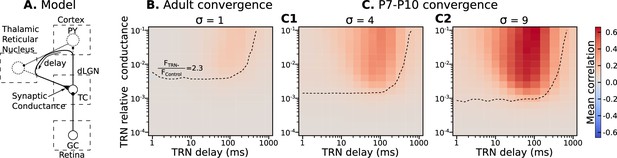
Parameters of TRN inhibitory feedback, that match in vivo observations, are outside the range when they can induce precise correlation in TC neurons.
(A) Schematic of the model design. (B) Effect of different levels of TRN relative inhibitory conductance (ordinate) and delay (abscissa) on mean spike correlations in a model with adult-like convergence of rGC inputs to a single TC neuron (). The estimated effect of TRN silencing on firing rate is shown as a dashed line. (C) The same as in B but for two estimates of P7-P10 convergence: 10 inputs per TC neuron (, C1) and 20 inputs per TC neuron (, C2).
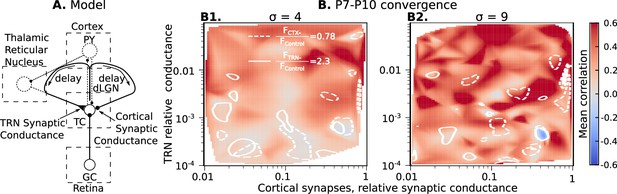
Cortical excitation and TRN inhibition parameters that match in vivo observations are outside values where they can synchronize TC neurons.
(A) Schematic of the extended model. (B) Dependence of mean network correlation upon TRN and cortical relative synaptic conductance. Two heatmaps with the convergence of 10 inputs per TC neuron (, B1) and 20 inputs per TC neuron (, B2) are shown. White solid lines and white dash lines indicate mean changes in firing rate, which match the observed TRN silencing () and cortex silencing () in vivo experiments, correspondingly. These show the regions of possible conductance based on in vivo values.
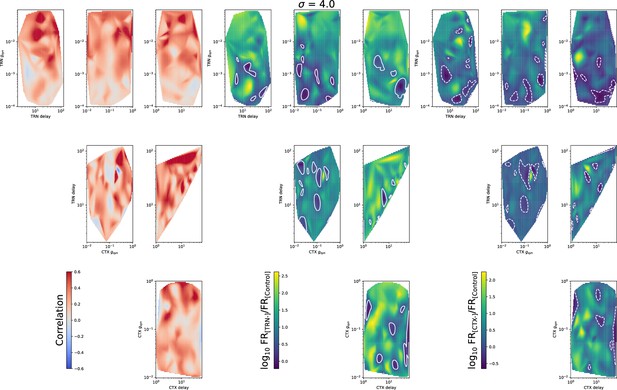
Heatmaps for mean spike correlation (left set), (middle set), and (right set) for all four model parameters: TRN synaptic conductance , TRN delay, CTX synaptic conductance , and CTX delay.
Convergence
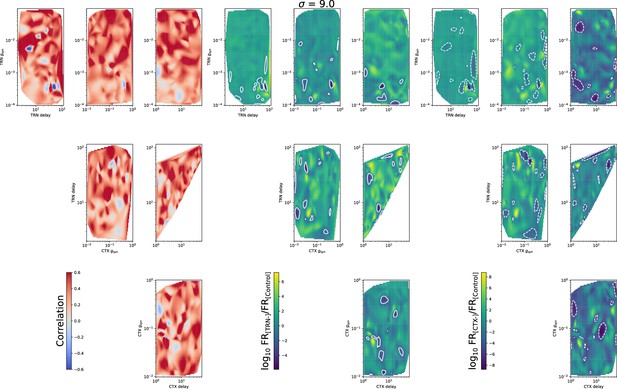
The same as in Figure 5—figure supplement 1, but for .
Tables
Open Model Parameters.
Parameter(s) | Scale | minimal boundary | maximal boundary |
---|---|---|---|
soma.L | linear | 20. | 200. |
soma(0.5).pas.e | linear | –55. | –90. |
soma(0.5).pas.g | logarithmic | 1e-7 | 1e-1 |
soma(0.5).TC_HH.gk_max | logarithmic | 1e-7 | 1e-1 |
soma(0.5).TC_HH.gna_max | logarithmic | 1e-7 | 1e-1 |
soma(0.5).TC_HH.vtraub | linear | –70. | 20. |
soma(0.5).TC_HH.vtraub2 | linear | –70. | 20. |
soma(0.5).SK_E2.gSK_E2bar | logarithmic | 1e-7 | 1e-1 |
soma(0.5).SK_E2.zTau | linear | 1. | 500. |
soma(0.5).TC_iT_Des98.shift | linear | –25. | 25. |
soma(0.5).TC_iT_Des98.actshift | linear | –25. | 25. |
soma(0.5).TC_iT_Des98.pcabar | logarithmic | 1e-7 | 5e-1 |
soma(0.5).TC_ih_Bud97.gh_max | logarithmic | 1e-7 | 5e-1 |
soma(0.5).TC_ih_Bud97.e_h | linear | –50. | 0. |
soma(0.5).TC_Nap_Et2.gNap_Et2bar | logarithmic | 1e-7 | 5e-1 |
soma(0.5).TC_cad.taur | linear | 2. | 30. |
soma(0.5).TC_cad.gamma | logarithmic | 1e-5 | 1e-1 |
soma(0.5).TC_iA.gk_max | logarithmic | 1e-7 | 1e-1 |
soma(0.5).TC_iL.pcabar | logarithmic | 1e-7 | 1e-1 |
soma.cao | linear | 1. | 6. |
soma.ena, axon.ena | linear | 40. | 65. |
soma.ek, axon.ek | linear | –65. | –110. |
axon.diam | linear | from mrth import *.5 | 5. |
axon.L | linear | 100. | 1000. |
axon(0.5).TC_HH.gk_max | logarithmic | 1e-7 | 1. |
axon(0.5).TC_HH.gna_max | logarithmic | 1e-7 | 1. |
axon(0.5).TC_HH.vtraub | linear | –70. | 20. |
axon(0.5).TC_HH.vtraub2 | linear | –70. | 20. |
axon.Ra, soma.Ra | linear | 20. | 120 |