Lead-OR: A multimodal platform for deep brain stimulation surgery
Figures
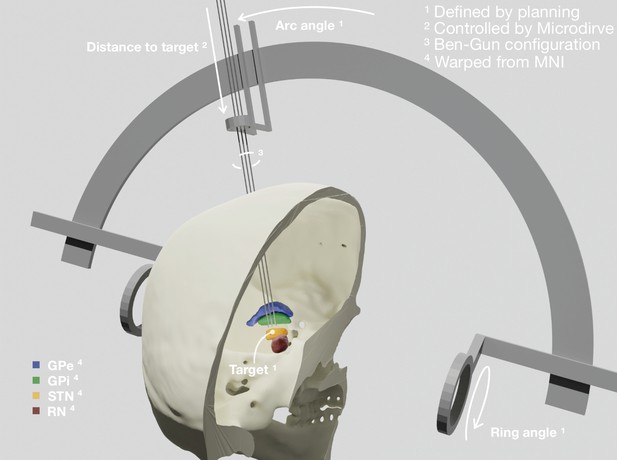
Patient-specific visualization generated by aggregating different sources of data.
The stereotactic planning procedure defines the surgical target coordinate, as well as ring and arc angles, which together describe the central trajectory. The Ben-Gun configuration presented in the figure shows additional posterolateral and anteromedial trajectories, 2 mm apart from the central one. Up to five trajectories are currently supported by the software. In our current setup, the distance to the target is controlled by the NeuroOmega system, accessed with its Software Development Kit (SDK) – but can alternatively be set manually within the tool itself. Relevant subcortical nuclei have been warped to patient space via a manually refined normalization. GPe: external segment of the globus pallidus; GPi: internal segment of the globus pallidus; STN: subthalamic nucleus; RN: red nucleus.
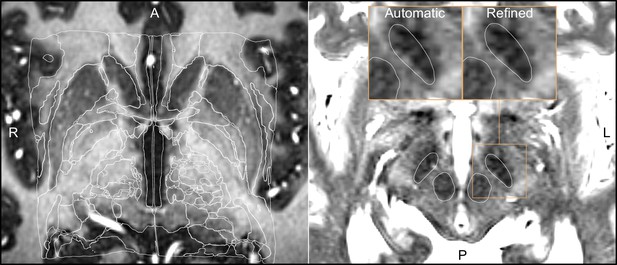
Output of a Lead-DBS/Advanced Normalization Tools (ANTs)-based automated normalization with and without subsequent manual refinement.
Two MRI modalities are shown anterior commissure-posterior commissure (AC-PC) aligned: T1-MPRAGE (left) and T2-TSE (right). Both MRI modalities (together with FGATIR, not shown here) were used as an input to the normalization step implemented in Lead-DBS, which allows multispectral registration using ANTs. The white outline shows atlases: MNI PD 25 histology (Xiao et al., 2017) (left) and DISTAL (Ewert et al., 2018) (right), both included within Lead-DBS.
General overview of the visualizations and tools made available through the WarpDrive module implementation in Slicer.
The video shows an image normalized to MNI space and how the Smudge and Drawing tools can help refine the displacement field.
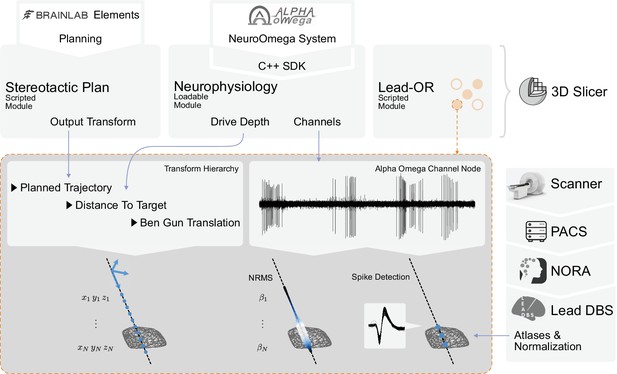
Overview of the SlicerNetstim extension.
The current setup shows interfaces with specific commercial products. Similar interfaces to competing tools are planned and will be included in the future. A PDF plan exported from Brainlab Elements is used as an input to the Stereotactic Plan module to store the planned trajectory as a Slicer Transform. The NeuroOmega system is connected via its Software Development Kit (SDK) through the Neurophysiology module, providing continuous updates about the drive depth and electrophysiological channel input. Finally, in the Lead-OR module, the Ben-Gun configuration is defined by selecting the used trajectories and assigning them to input channels from the NeuroOmega device. Using a transform hierarchy, the spatial position of the microelectrode is defined: the Ben-Gun translation is transformed by the distance to the target, this one itself being transformed by the planned trajectory. By doing so, the features extracted from the respective microelectrode recordings (MERs) can be mapped to their spatial location. At our center, an automatic pipeline for preprocessing data retrieved from a picture archiving and communication system (PACS) system is setup using the NORA medical imaging platform (https://www.nora-imaging.com/) to automatically run the core Lead-DBS pipeline once images arrive in the hospital’s PACS system. This part (right-hand side) is not discussed in detail since it is largely specific to our center.
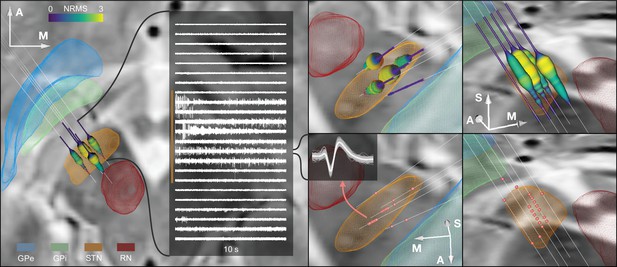
Example case showing trajectories, microelectrode recording (MER) features, and DISTAL atlas volumes mapped to patient space.
10 s recording snippets from one trajectory are displayed. Normalized root mean square (NRMS) activity is represented by a tube with varying diameter and color matching the value. Spike clusters are represented by red point fiducials. GPe: external segment of the globus pallidus; GPi: internal segment of the globus pallidus; STN: subthalamic nucleus; RN: red nucleus.
-
Figure 4—source data 1
Slicer scene containing the spatial data shown in the Figure 4.
- https://cdn.elifesciences.org/articles/72929/elife-72929-fig4-data1-v2.zip
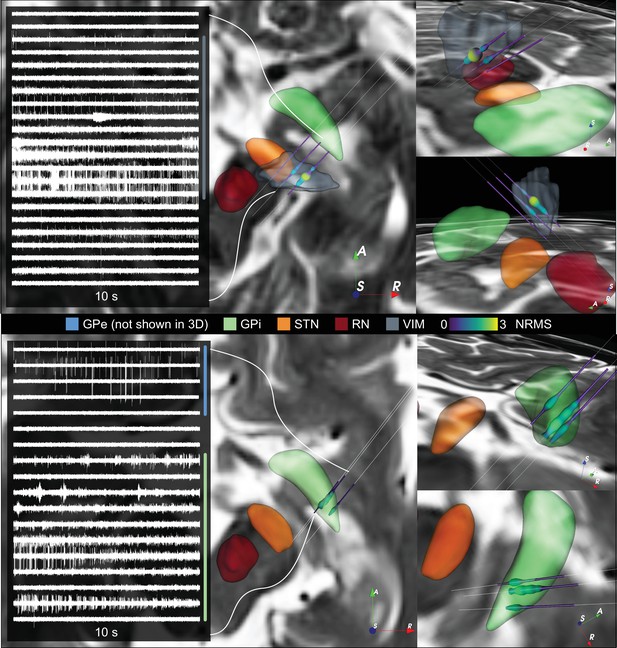
Additional ventral intermediate nucleus (VIM) and internal segment of the globus pallidus (GPi) cases.
Additional VIM and GPi cases are shown in top and bottom panels, respectively. The figure shows 10 s electrophysiology extracts together to their normalized root mean squared value mapped to space using Lead-OR. DISTAL atlas nuclei were warped to patient space. GPe: external segment of the globus pallidus; STN: subthalamic nucleus; RN: red nucleus.
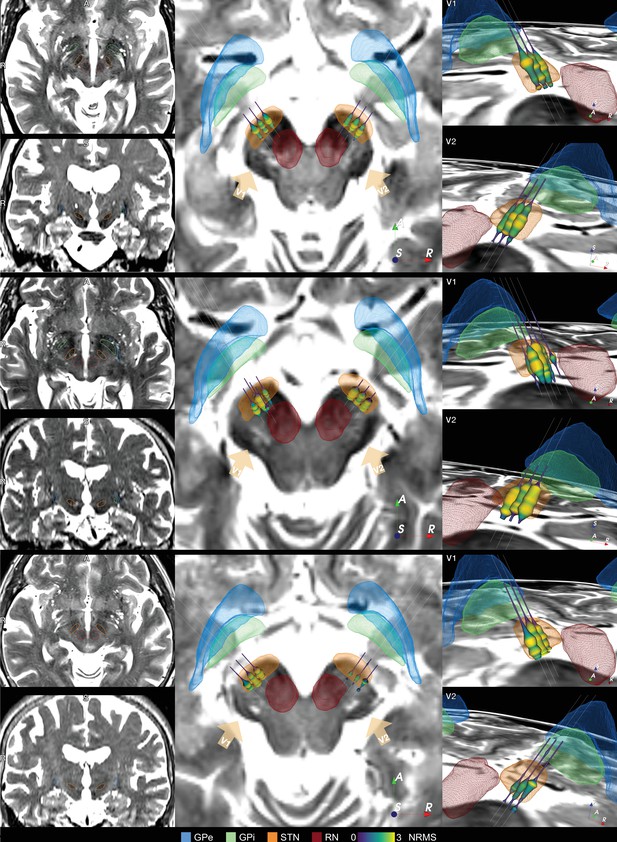
Additional example cases of subthalamic nucleus-deep brain stimulation (STN-DBS) Lead-OR visualizations.
Example cases of STN-DBS showing preoperative MRIs in synopsis with resulting Lead-OR visualizations. The three cases feature unusual anatomical configurations with comparably narrow skull (top), asymmetric hemispheres and STN (middle), and comparably broad skull (bottom). The atlas-based segmentation using Lead-DBS following WarpDrive refinement was able to capture individual patient anatomy in all cases. GPe: external segment of the globus pallidus; GPi: internal segment of the globus pallidus; RN: red nucleus.
General overview of the Lead-OR real-time application.
The video shows patient-specific imaging together with atlases, the BigBrain template (Amunts et al., 2013), planned microelectrode trajectories, microelectrode recordings (MERs) and feature extraction, and a test stimulation example.
Video showing the program user interface and its use.
Includes exemplary connection to the hardware device, channel selection for recording, configuration of trajectories, input planning, stimulation setup, and visualization and processing of live data streaming into the software.
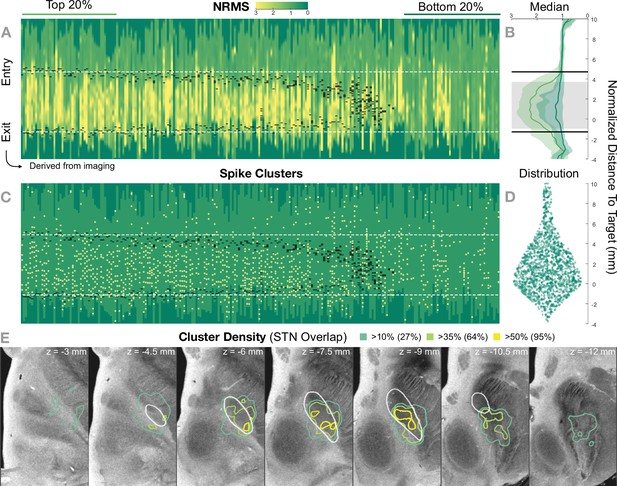
Retrospective group analysis investigating agreement between imaging- and electrophysiology-defined subthalamic nucleus (STN).
In (A) and (C), each trajectory is presented as a column, showing normalized root mean square activity (NRMS) and spike clusters, respectively, with the normalized distance to target denoted on vertical axes. Trajectories are sorted from left to right according to their distance to the STN as defined in the DISTAL atlas (Ewert et al., 2018). Dark green values (indicating NRMS of zero) represent no recordings at these sites. Black dashes represent STN entry and exit, and the dashed white line the median entry and exit for the top 20%. (B) shows comparisons between bottom and top trajectories, with the gray area representing a significant band (nonparametric Wilcoxon’s signed-rank test p<0.01 with false discovery rate [FDR] correction), which resides within the STN. The plots show median, 0.25 and 0.75 quantiles. (D) shows the overall distribution of spike clusters. (E) shows isosurfaces of a volume where each voxel contains the number of clusters detected divided by the number of recordings carried out within 1 mm distance to the location (cluster density). The legend shows the percentage of the volume overlap with the STN at different thresholds. The 7 T MRI ex vivo human brain template (Edlow et al., 2019) is shown as the background image with DISTAL STN outline. Decreasing values on the z-axis traverse from superior to inferior.
-
Figure 5—source code 1
Uses Figure 5—source data 1 to generate Figure 5—source data 2 and panels A–D.
- https://cdn.elifesciences.org/articles/72929/elife-72929-fig5-code1-v2.zip
-
Figure 5—source code 2
Uses Figure 5—source data 1 to generate Figure 5—figure supplement 1.
- https://cdn.elifesciences.org/articles/72929/elife-72929-fig5-code2-v2.zip
-
Figure 5—source data 1
Trajectories data including normalized root mean square (NRMS) traces, subthalamic nucleus (STN) entry-exit positions, spike clusters, brain shift, and distance to STN values.
- https://cdn.elifesciences.org/articles/72929/elife-72929-fig5-data1-v2.zip
-
Figure 5—source data 2
Cluster density volume shown in Figure 5E.
- https://cdn.elifesciences.org/articles/72929/elife-72929-fig5-data2-v2.zip
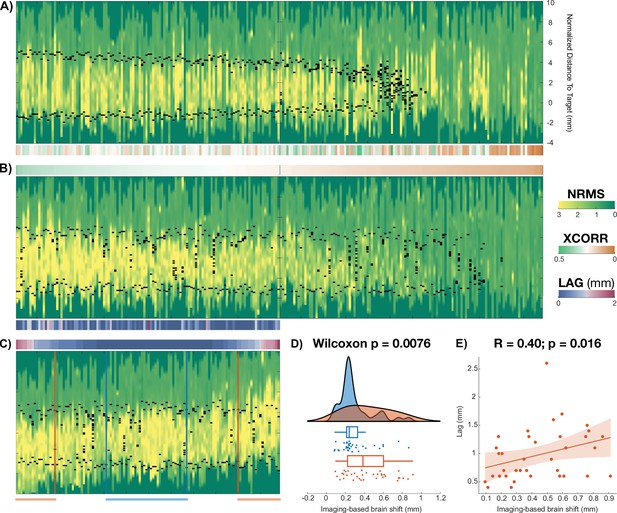
Brain shift study.
Staring from Figure 5A, this figure shows the analysis done to derive the relationship between imaging-based and electrophysiology-based brain shift. Brain shift analysis. (A) shows the same trajectory traces as in Figure 5A. For each of the trajectories, the cross-correlation sequence was computed between the normalized root mean square (NRMS) and distance to subthalamic nucleus (STN) values along the trajectory (Figure 5—source code 2). (B) displays the trajectories sorted according to the peak value of the cross-correlation sequence (color bars between A and B visualize this sorting). After discarding the bottom half trajectories (trajectories distant to the STN with low NRMS values), the remaining ones were sorted once more, this time according to their lag (or displacement) at which the maximum occurred (C). This measure quantifies the shift between signals and served as an electrophysiology-derived estimate of brain shift. (D) shows agreements between electrophysiology- and imaging-derived measures of brain shift. Contrasting imaging-derived brain shift estimates between low- versus high-lag (value above 1 SD) groups showed significant difference in their distributions (Wilcoxon’s signed-rank test ). (E) shows the correlation between electrophysiology- and imaging-derived estimates of brain shift (, ).
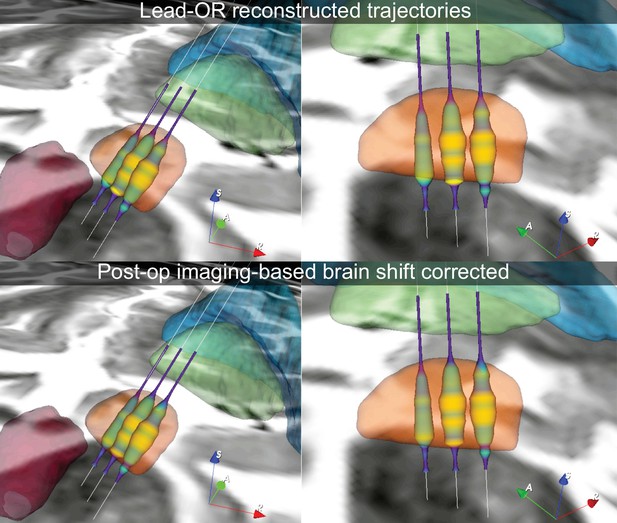
Example case in which the imaging-derived brain shift transform was applied to the Lead-OR scene, post-hoc.
The top panels feature the actual scene as observed during surgery, in which a slight displacement between imaging (too dorsal) and electrophysiology (too ventral) is apparent. By correcting for brain shift based on postoperative CT, this discrepancy could be accounted for.
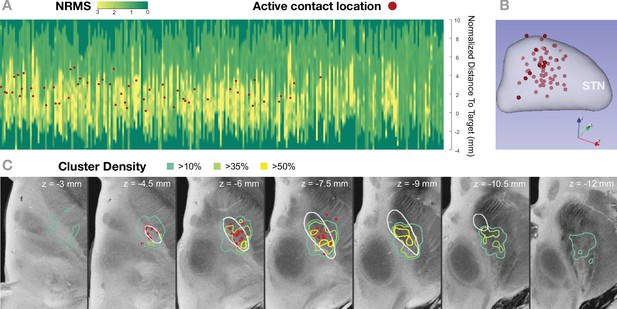
Active contact coordinates overlayed with Figure 5A and E.
(A) and (C) show normalized root mean square (NRMS) and cluster density results as in Figure 5A and E, respectively. This figure additionally includes clinically active contact locations overlayed. (A) shows NRMS traces for the 236 trajectories analyzed in this study and clinical active coordinates projected to the nearest point in the closest trajectory. (B) shows the locations of the active contacts in MNI space (left hemisphere) together with the subthalamic nucleus (STN) from DISTAL atlas. Right hemispheric electrode contacts were flipped to the left. (C) shows isosurfaces of the cluster density volume (i.e., number of spike clusters divided by number of trajectories within 1 mm radius for each voxel) and the active coordinate location. Slices from the 7 T MRI ex vivo human brain template (Edlow et al., 2019) are shown as the background image with DISTAL STN outline. A general correspondence can be seen between active contact location and features extracted from the microelectrode recordings.
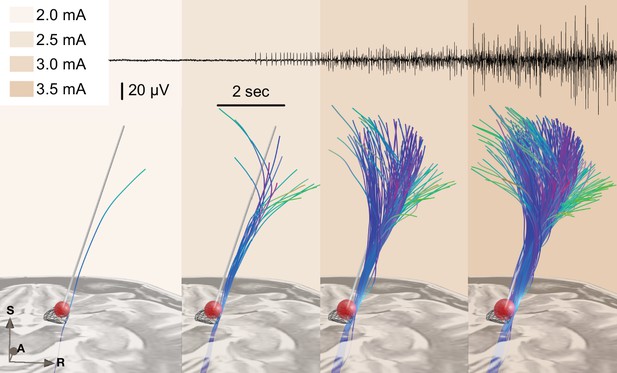
Example of test stimulation setup (also see Figure 4—video 1 for the demonstration of a real-time application).
A simplified stimulation volume is modeled based on the applied test stimulation parameters following the approach of Dembek et al., 2017. From a set of predefined fiber tracts representing the internal capsule (without hyperdirect components; Petersen et al., 2019) that were registered to patient space, fibers passing through the volume were visualized in real time. Alternatively, tractograms obtained based on diffusion MRI of the individual patient data or normative connectomes could be used. The top panel shows needle electromyography (EMG) activity that was recorded within clinical routine from the brachioradialis muscle during stimulation in the same patient. Colors represent stimulation amplitude. After a preliminary exploratory analysis of the k2 parameter from Dembek’s formula, a value of 0.8 was used for the shown example.
-
Figure 6—source data 1
Slicer scene containing the spatial data shown in the Figure 6.
- https://cdn.elifesciences.org/articles/72929/elife-72929-fig6-data1-v2.zip
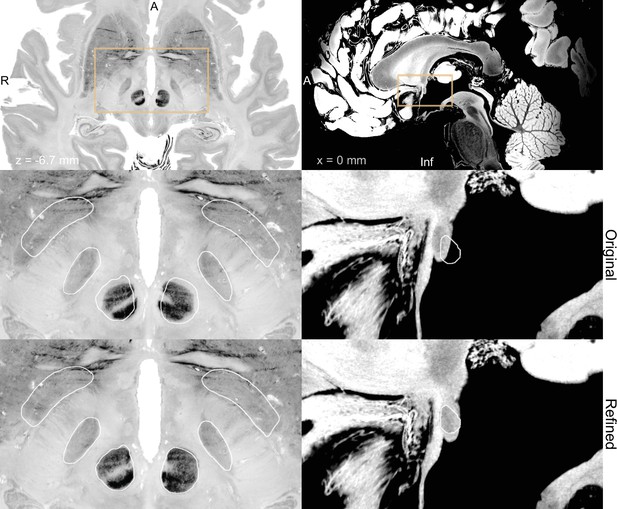
BigBrain (Amunts et al., 2013; Xiao et al., 2019) (left) and 7 T MRI ex vivo human brain template (Edlow et al., 2019) (right) are two high-resolution (100 µm isotropic) imaging resources that can be used from Lead-DBS and Lead-OR.
The middle panel shows a closeup in a plane using the original transformation to MNI space (Xiao et al. in the case of BigBrain) with white outlines of DISTAL atlas (Ewert et al., 2018) (left) and anterior commissure from Neudorfer et al., 2020 (right). The bottom panel shows the same slice, but using a refined transformation using Lead-DBS and WarpDrive. The refined transformation files can be found in the supplementary data repository (Oxenford, 2022a).
Additional files
-
Transparent reporting form
- https://cdn.elifesciences.org/articles/72929/elife-72929-transrepform1-v2.pdf
-
Supplementary file 1
Inclusion flow chart.
- https://cdn.elifesciences.org/articles/72929/elife-72929-supp1-v2.pdf
-
Reporting standard 1
STROBE checklist.
- https://cdn.elifesciences.org/articles/72929/elife-72929-repstand1-v2.pdf