Navigation: Where is that smell coming from?
Dogs, rodents and many other animals with a strong sense of smell often track a scent by keeping their nose to the ground, occasionally pausing to raise their heads and sniff something mysterious in the air (Jinn et al., 2020; Gire et al., 2016). However, exactly how alternating between these two behaviors helps animals navigate to the source of an odor remains unclear.
Airborne odors are transported by the wind, making them subject to the twisting and stretching of turbulent air motions. This results in animals downwind from an odor source being more likely to smell the odor intermittently, as air pockets containing the scent are interspersed with periods of clean air (Celani et al., 2014; Connor et al., 2018). Studies in insects suggest that animals surge upwind when they detect a smell in order to keep in contact with these turbulent odor plumes; when no odor is detected, they cast crosswind instead (Álvarez-Salvado et al., 2018; Demir et al., 2020; Kennedy, 1983; Flügge, 1934). Now, in eLife, Nicola Rigolli, Gautam Reddy, Agnese Seminara and Massimo Vergassola report how pausing to sniff the air when casting crosswind helps animals navigate towards the source of an odor (Rigolli et al., 2022).
To investigate how alternating behaviors impacts odor navigation, the team (who are based at institutes in France, Italy and the United States) designed a virtual search environment by simulating an odor dispersing downwind over a large area. The set-up created a challenging search scenario, including a low probability of encountering an odor pocket far from the source. Using machine learning, computer programs trained ‘artificial navigating agents’ to find the origin of the smell as quickly as possible (Sutton and Barto, 2019). During their search, these artificial agents were allowed to alternate between ‘walking’ while sniffing close to the ground and stopping to smell the air. Information gathered from these behaviors allowed agents to decide where to go next. After each attempt, the agents could use feedback about their previous search times to modify their strategy in the next trial. Although the researchers did not impose any explicit strategy or solution, agents reliably learnt that stopping to sniff the air sped up their search, even though it required them to pause.
Notably, trained agents mostly stopped to smell the air during the initial phase of their search before they had detected any odor. This suggests that alternating between ground and air sniffing helps agents to explore areas with dispersed levels of odor more efficiently (Figure 1A). Once agents successfully encounter an airborne cue, this signals that they have entered an odor rich zone and their rate of alternation drastically decreases.
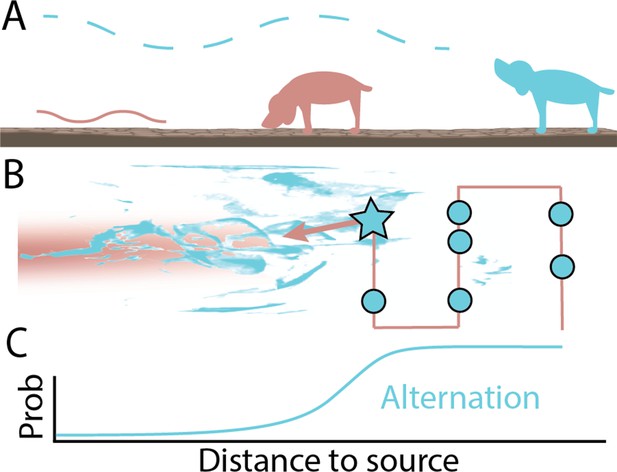
The optimal strategy for finding the source of a smell.
(A) When tracking the source of an odor, animals alternate between walking while sniffing the ground (brown) and pausing to sniff the air (blue). Animals sniff the air more frequently when they are further away from the source and airborne cues are more dispersed (blue dashed line). As they get nearer and the density of the airborne cues increases (brown line), animals alternate less frequently and track the scent by sniffing close to the ground. (B) Rigolli et al. used a machine learning algorithm to identify the optimal strategy for tracking odors in the wind. They simulated an odor dispersing in the air (blue plume) and close to the ground (brown plume) and then trained artificial agents to find the source of the smell: the brown line indicates the trajectory agents took whilst sniffing the floor, and the blue circles represent where they paused to sniff the air. The algorithm revealed that the best way for agents to find the source of the odor was for them to alternate to sniffing the air when moving crosswind, and intersperse this with occasional surges forward until an odor was detected (blue star). (C) The simulation showed that agents displayed this alternating behavior less frequently as they moved closer to the odor source.
Image credit: Samuel Brudner; odor plumes in panel B are based on images by Nirag Kadakia and Mahmut Demir.
But how exactly does alternation speed up getting that first hint of a scent? Failing to detect an odor in a region indicates that the source of the smell is unlikely to be upwind of this area, eliminating the need to search there in the future. Odors disperse slower near the ground, and as a result do not reach as far as odors travelling in the air. Sniffing above their heads therefore allows agents to rule out larger upwind areas (if no smell is present), while also increasing the likelihood of detecting faint signals that are absent at ground level.
The simulation also revealed that during the early search phase, trained agents combined alternation with specific patterns of locomotion (Figure 1B). Agents moving crosswind sniffed the air more frequently than when they surged upwind. Rigolli et al. observed that this behavior helps the agents to rule out cross-sections of the simulated arena before moving upwind to gather evidence about a new region.
This cast-sniff-surge strategy involves many tradeoffs: casting over a wider distance takes longer but also eliminates a wider cross-section; sniffing in the air requires stopping and therefore losing time. Using a mathematical framework, Rigolli et al. show that optimally balancing these tradeoffs requires exploring the arena back and forth in an expanding crosswind zigzag, gradually casting across larger areas as the search progresses. Remarkably, these characteristics also appeared in trained agents which were not constrained to use a cast-sniff-surge approach.
Overall, Rigolli et al. demonstrate how, in theory, alternating between sniffing the ground and the air allows animals to efficiently search large areas for an odor source. Future studies should now test these predictions, for example examining if real animals do tend to alternate behaviors mostly in odor-poor regions. This work could also be applied to robotics, in particular to improve the exploratory behavior of drones used in difficult search-and-rescue operations.
References
-
Odor landscapes in turbulent environmentsPhysical Review X 4:041015.https://doi.org/10.1103/PhysRevX.4.041015
-
Quantification of airborne odor plumes using planar laser-induced fluorescenceExperiments in Fluids 137:2591-3.https://doi.org/10.1007/s00348-018-2591-3
-
Geruchliche raumorientierung von Drosophila melanogasterZeitschrift Für Vergleichende Physiologie 20:463–500.https://doi.org/10.1007/BF00339650
-
Zigzagging and casting as a programmed response to wind-borne odour: a reviewPhysiological Entomology 8:109–120.https://doi.org/10.1111/j.1365-3032.1983.tb00340.x
Article and author information
Author details
Publication history
Copyright
© 2022, Brudner and Emonet
This article is distributed under the terms of the Creative Commons Attribution License, which permits unrestricted use and redistribution provided that the original author and source are credited.
Metrics
-
- 712
- views
-
- 67
- downloads
-
- 1
- citations
Views, downloads and citations are aggregated across all versions of this paper published by eLife.
Download links
Downloads (link to download the article as PDF)
Open citations (links to open the citations from this article in various online reference manager services)
Cite this article (links to download the citations from this article in formats compatible with various reference manager tools)
Further reading
-
- Physics of Living Systems
In social behavior research, the focus often remains on animal dyads, limiting the understanding of complex interactions. Recent trends favor naturalistic setups, offering unique insights into intricate social behaviors. Social behavior stems from chance, individual preferences, and group dynamics, necessitating high-resolution quantitative measurements and statistical modeling. This study leverages the Eco-HAB system, an automated experimental setup that employs radiofrequency identification tracking to observe naturally formed mouse cohorts in a controlled yet naturalistic setting, and uses statistical inference models to decipher rules governing the collective dynamics of groups of 10–15 individuals. Applying maximum entropy models on the coarse-grained co-localization patterns of mice unveils social rules in mouse hordes, quantifying sociability through pairwise interactions within groups, the impact of individual versus social preferences, and the effects of considering interaction structures among three animals instead of two. Reproducing co-localization patterns of individual mice reveals stability over time, with the statistics of the inferred interaction strength capturing social structure. By separating interactions from individual preferences, the study demonstrates that altering neuronal plasticity in the prelimbic cortex – the brain structure crucial for sociability – does not eliminate signatures of social interactions, but makes the transmission of social information between mice more challenging. The study demonstrates how the joint probability distribution of the mice positions can be used to quantify sociability.
-
- Physics of Living Systems
Chromosome structure is complex, and many aspects of chromosome organization are still not understood. Measuring the stiffness of chromosomes offers valuable insight into their structural properties. In this study, we analyzed the stiffness of chromosomes from metaphase I (MI) and metaphase II (MII) oocytes. Our results revealed a tenfold increase in stiffness (Young’s modulus) of MI chromosomes compared to somatic chromosomes. Furthermore, the stiffness of MII chromosomes was found to be lower than that of MI chromosomes. We examined the role of meiosis-specific cohesin complexes in regulating chromosome stiffness. Surprisingly, the stiffness of chromosomes from three meiosis-specific cohesin mutants did not significantly differ from that of wild-type chromosomes, indicating that these cohesins may not be primary determinants of chromosome stiffness. Additionally, our findings revealed an age-related increase of chromosome stiffness for MI oocytes. Since aging is associated with elevated levels of DNA damage, we investigated the impact of etoposide-induced DNA damage on chromosome stiffness and found that it led to a reduction in stiffness in MI oocytes. Overall, our study underscores the dynamic and cyclical nature of chromosome stiffness, modulated by both the cell cycle and age-related factors.