Affected cell types for hundreds of Mendelian diseases revealed by analysis of human and mouse single-cell data
Figures
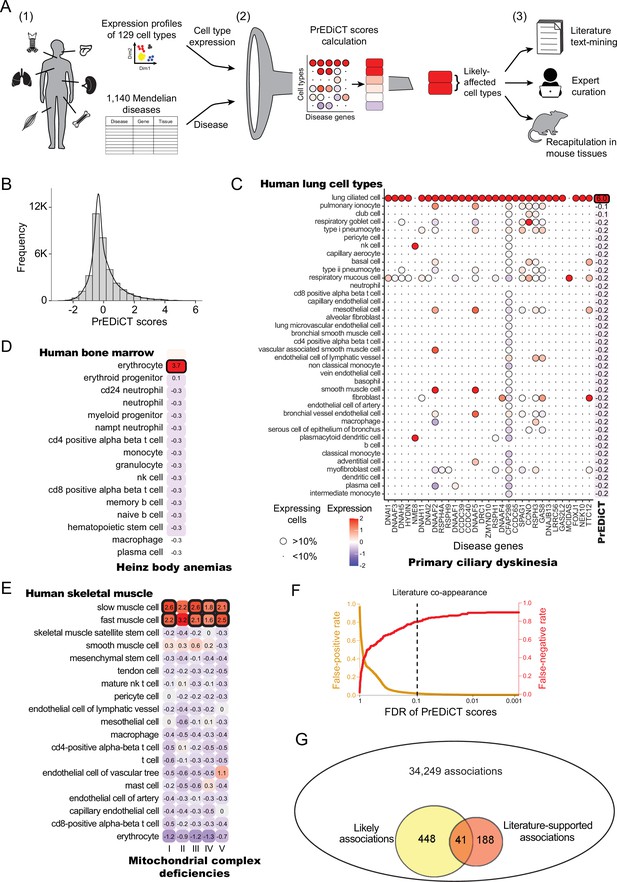
Overview of PrEDiCT calculation and assessment.
(A) The PrEDiCT workflow. In step 1, we analyzed single-cell expression data from six human tissues and 129 cell types, and associated 1,140 Mendelian diseases with their affected tissues. In step 2, we calculated the preferential expression of disease genes in cell types of disease-affected tissues, used their median to produce the PrEDiCT score per disease and cell type, and assessed significance of each score. In step 3, we validated likely disease–cell-type associations (i.e., PrEDiCT ≥1, FDR <0.1) via literature text-mining, expert curation, and analysis of mouse single-cell expression data. (B) The distribution of PrEDiCT scores in human (median –0.25±0.93). (C) The preferential expression of genes causal for primary ciliary dyskinesia (PCD) and the PrEDiCT scores of PCD in lung cell types. Preferential expression values and the percentage of cells expressing a gene are indicated by the color and the size of each circle, respectively. The resulting PrEDiCT score is displayed on the right, colored by the score value. Bold outline marks likely disease-affected cell types. (D) PrEDiCT scores of Heinz body anemias across human bone marrow cell types depicted as described in panel C. (E) PrEDiCT scores of mitochondrial complex deficiencies across human skeletal muscle cell types depicted as described in panel C. Mitochondrial complex deficiencies were likely to affect slow and fast muscle cells, except for mitochondrial complex II deficiency whose PrEDiCT scores were highest yet insignificant in these cell types (FDR = 0.61 and 0.23, respectively). (F) False-positive (red) and false-negative (orange) rates of disease–cell-type associations (y-axis) across FDR thresholds (x-axis). Rates were estimated based on literature-supported pairs. The dashed line marks the FDR cutoff for likely associations. (G) The overlap between likely disease–cell-type associations (total of 489, left circle) and literature-supported associations (total of 229, right circle) out of all 34,249 possible associations. The overlap of 41 associations was significant (p<E-15, Fisher’s exact test), supporting the validity of likely associations.
-
Figure 1—source data 1
The Source Data file contains data used to generate Figure 1B-G.
- https://cdn.elifesciences.org/articles/84613/elife-84613-fig1-data1-v2.xlsx
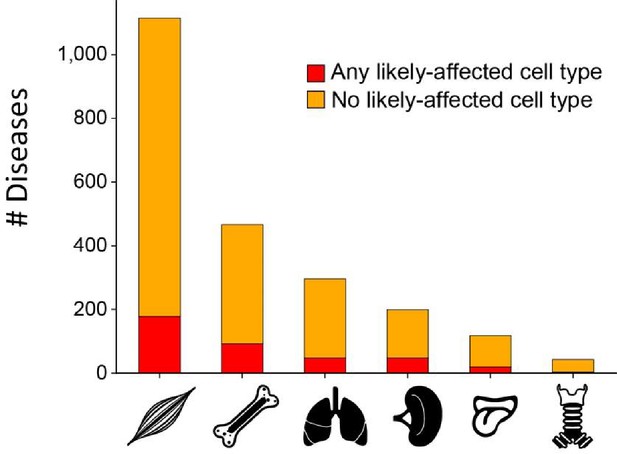
Disease-affected tissues.
Bar plot of the number of diseases per affected tissue that were either associated with any likely affected cell type (red) or not (orange).
-
Figure 1—figure supplement 1—source data 1
The Source Data file contains the number of diseases with and without likely affected cell types per tissue.
- https://cdn.elifesciences.org/articles/84613/elife-84613-fig1-figsupp1-data1-v2.xlsx
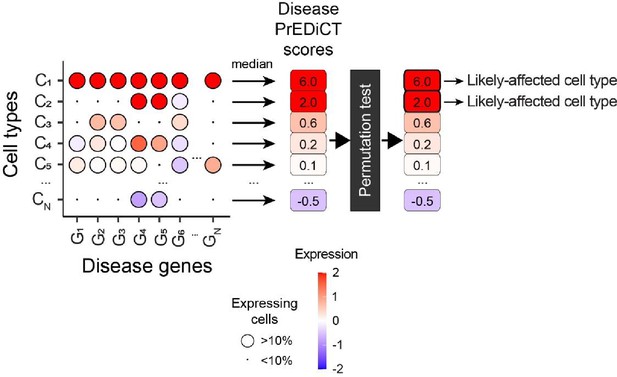
The PrEDiCT scheme.
To calculate PrEDiCT scores, we first calculated the preferential expression of disease genes in each cell type relative to other cell types of the disease-affected tissue. Next, we set the PrEDiCT score of a disease in each cell type to the median preferential expression of disease genes, and applied permutation tests to assess PrEDiCT score statistical significance. Cell types with significant PrEDiCT scores ≥1 were considered as likely affected.
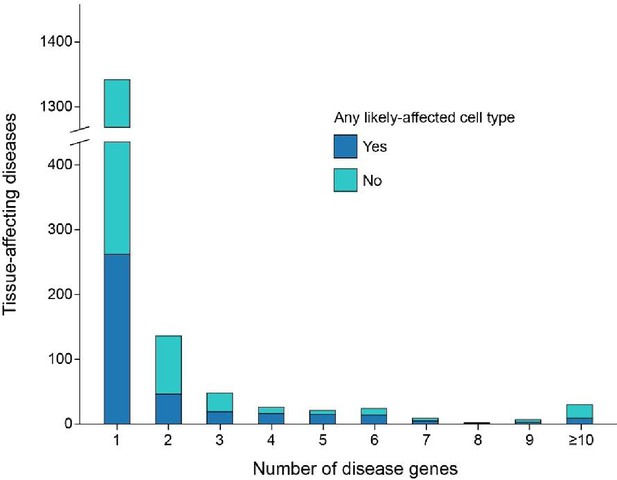
Distribution of diseases by the number of disease genes.
We considered pairs of diseases and affected tissues (briefly ‘Disease-tissue pairs’), and plotted their distribution by the number of disease genes that were expressed in that tissue. Pairs that were associated with a likely affected cell type appear in blue, otherwise in turquoise.
-
Figure 1—figure supplement 3—source data 1
The Source Data file contains data of numbers of diseases per number of disease-assoociated genes with and without likely affected cell types.
- https://cdn.elifesciences.org/articles/84613/elife-84613-fig1-figsupp3-data1-v2.xlsx
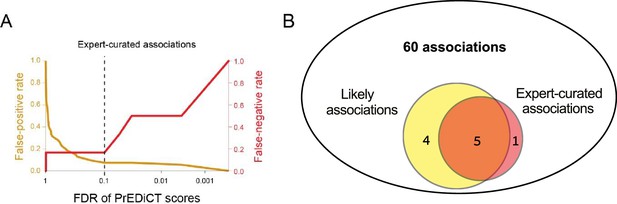
PrEDiCT scheme assessment using expert-curated associations.
(A) False-positive and false-negative rates (y-axes; colored red and orange, respectively) across varying FDR thresholds (x-axis) of expert-curated disease–cell-type associations. (B) The overlap between the number of likely disease–cell-type associations (total of 9; left circle) and the number of expert-curated associations (total of 6; right circle). Likely disease–cell-type associations were enriched for verified expert-curated pairs (5/9, 56%) relative to their frequency among the expert-analyzed pairs (6/60, 10%; p=1.3E-4, Fisher’s exact test).
-
Figure 1—figure supplement 4—source data 1
The Source Data file contains data used to generate Figure 1—figure supplement 4A, B.
- https://cdn.elifesciences.org/articles/84613/elife-84613-fig1-figsupp4-data1-v2.xlsx
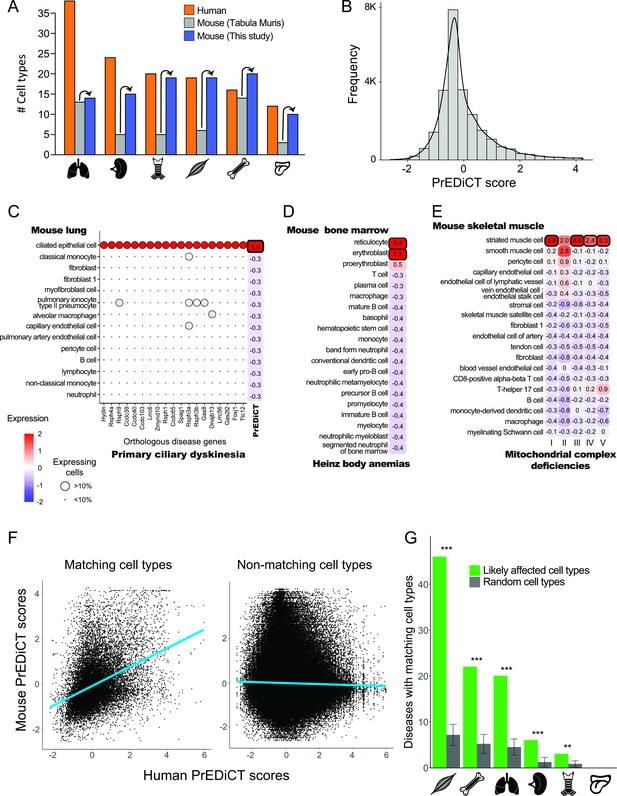
Recapitulation of disease-affected cell types in mouse.
(A) The number of human cell types annotated by Tabula Sapiens [(Jones et al., 2022); red], and the number of mouse cell types annotated by [Tabula Muris, 2018; grey] and this study (blue). (B) The distribution of PrEDiCT scores in mouse. (C) The preferential expression of mouse orthologs of PCD disease genes and the PrEDiCT scores of PCD in mouse lung cell types. Preferential expression values and the percentage of cells expressing a gene are indicated by the color and the size of each circle, respectively. The resulting PrEDiCT score is indicated on the right colored by the score value. Bold outline marks likely disease-affected cell types. (D) PrEDiCT scores of Heinz body anemias across mouse bone marrow cell types depicted as described in panel C. (E) PrEDiCT scores of mitochondrial complex deficiencies across mouse skeletal muscle cell types depicted as described in panel C. Mitochondrial complex deficiencies were likely associated with striated muscle cells, except for mitochondrial complex II deficiency whose PrEDiCT scores were highest yet insignificant in these cell types (FDR = 0.65). (F) The correlation between PrEDiCT scores in human (X-axis) and mouse (Y-axis) cell types. Each dot represents a distinct pair. PrEDiCT scores of non-matching cell types did not correlate (left; r=−0.02, Spearman correlation), in contrast to PrEDiCT scores of matching cell types (right; r=0.38, p<E-15). (G) The cell types affected by the same disease in human and mouse tended to match each other (green) more than expected by chance (grey) according to 1,000 repeats in a permutation test. Error bars represent the standard deviation of the number of randomly matching cell types between the species. Adjusted **p<0.01 and ***p<0.001, permutation test.
-
Figure 2—source data 1
The Source Data file contains data used to generate Figure 2A-G.
- https://cdn.elifesciences.org/articles/84613/elife-84613-fig2-data1-v2.xlsx
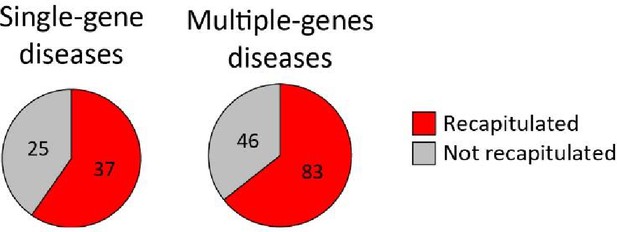
The fraction of likely disease–cell-type associations in human that were recapitulated in mouse.
The comparison included likely associations for diseases with expressed mouse ortholog(s), and cell types with any matching cell type in the mouse corresponding tissue. The fraction of likely disease–cell-type associations that were recapitulated was similar between diseases with a single disease gene (37/62, 60%) and those with multiple disease genes (83/129, 64%; p=0.63, Fisher’s exact test).
-
Figure 2—figure supplement 1—source data 1
The Source Data file contains the numbers of diseases with a single, or multiple, disease-associated genes that were or were not recapitulated using mouse expression data.
- https://cdn.elifesciences.org/articles/84613/elife-84613-fig2-figsupp1-data1-v2.xlsx
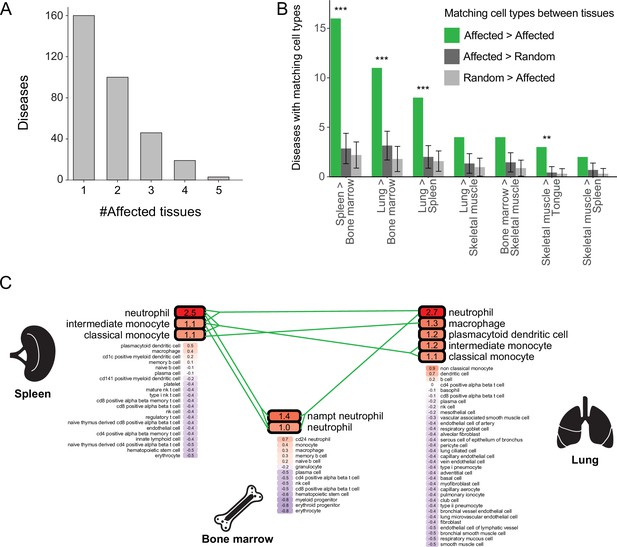
Multi-tissue diseases tend to affect similar cell types in those tissues.
(A) The numbers of diseases with likely affected cell type across tissues (Y-axis) grouped by the number of affected tissues (X-axis). (B) The number of diseases that likely affect at least one pair of matching cell types between tissues (green). This number was higher than expected by chance (dark and light grey correspond to selecting cell types at random from the first tissue or the second one, respectively) according to 1,000 repeats in a permutation test. Only pairs of tissues with ≥2 shared diseases are shown. Error bars represent the standard deviation of the number of randomly matching cell types between the tissues. Shown are maximal adjusted p-value for each pairwise randomization: **p<0.01, ***p<0.001. (C) PrEDiCT scores of cell types affected by chronic granulomatous disease (CGD) in spleen, lung, and bone marrow. Bold outline marks likely affected cell types. Likely affected cell types that were matching among the tissues were connected by green lines.
-
Figure 3—source data 1
The Source Data file contains data used to generate Figure 3A-C.
- https://cdn.elifesciences.org/articles/84613/elife-84613-fig3-data1-v2.xlsx
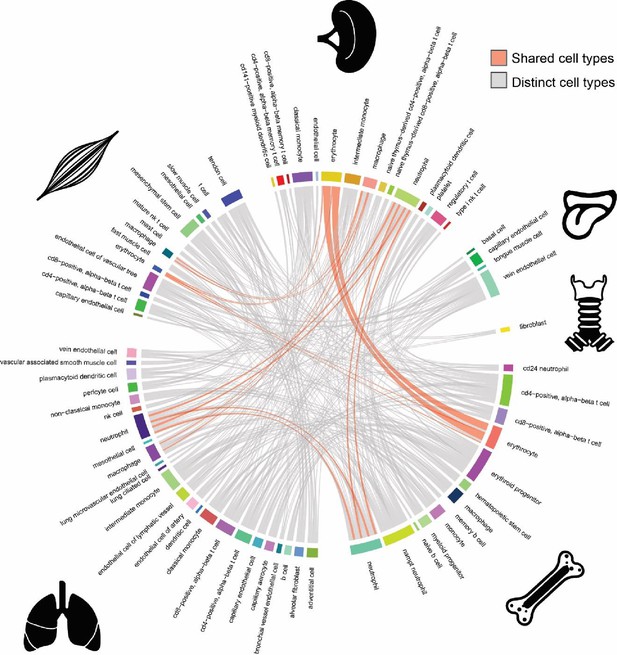
Cell-type similarity among human tissues.
Circos plot representing similarity between cell types among human tissues. Width and color of lines that connect each cell-type pair indicate the fraction of diseases likely affecting each cell type and whether the cell types match (red: matching, grey: non-matching; Supplementary file 5), respectively.
-
Figure 3—figure supplement 1—source data 1
The Source Data file contains data used to generate the circos plot that represents the similarity between cell types of distinct human tissues.
- https://cdn.elifesciences.org/articles/84613/elife-84613-fig3-figsupp1-data1-v2.xlsx
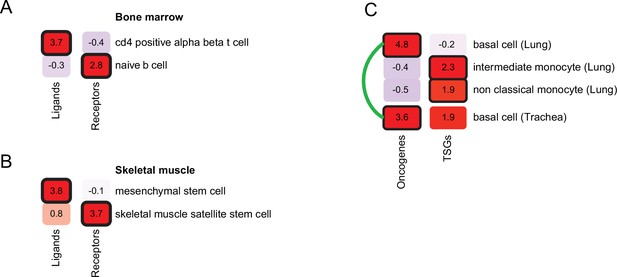
Refining cell-type inference using gene functions.
(A) PrEDiCT scores of cell types likely affected by hyper-IgM immunodeficiency in bone marrow, calculated separately for ligand- or receptor-encoding disease genes. (B) PrEDiCT scores of cell types likely affected by autosomal recessive limb-girdle muscular dystrophy in skeletal muscle, calculated separately for ligand- and receptor-encoding disease genes. (C) PrEDiCT scores of cell types likely affected by heritable cancers in lung and trachea, calculated separately for oncogenes and tumor suppressor genes. Likely affected cell types that matched between the tissues were connected by a green line. Bold outline marks likely-affected cell types.
-
Figure 4—source data 1
The Source Data file contains data used to generate Figure 4A-C.
- https://cdn.elifesciences.org/articles/84613/elife-84613-fig4-data1-v2.xlsx
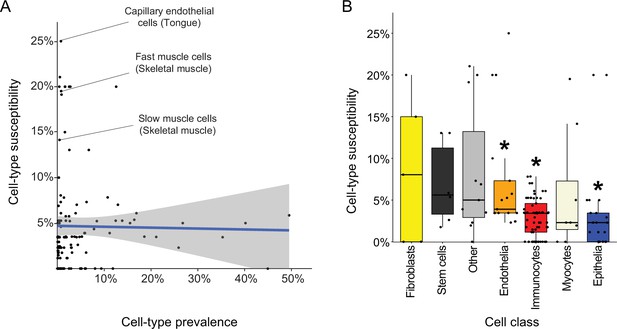
Characteristics of disease-affected cell types.
(A) Cell-type susceptibility (Y-axis) did not correlate with cell-type prevalence (X-axis). The blue line represents linear correlation (r=−0.02, Pearson correlation). (B) Cell-type susceptibility varied between cell classes (p=1.5E-3, ANOVA test). Among the cell classes with many cell types shared among tissues, immunocytes and epithelia were the least susceptible, and endothelia were the most susceptible compared to other cell types (adjusted p<0.05, Mann-Whitney U test; Methods).
-
Figure 5—source data 1
The Source Data file contains data used to generate Figure 5A, B.
- https://cdn.elifesciences.org/articles/84613/elife-84613-fig5-data1-v2.xlsx
Additional files
-
Supplementary file 1
Diseases, disease genes, and likely affected cell types of each tissue.
- https://cdn.elifesciences.org/articles/84613/elife-84613-supp1-v2.xlsx
-
Supplementary file 2
Diseases and their PrEDiCT scores across human cell types per tissue.
- https://cdn.elifesciences.org/articles/84613/elife-84613-supp2-v2.xlsx
-
Supplementary file 3
Names of disease and cell type co-appearance in PubMed records per tissue.
- https://cdn.elifesciences.org/articles/84613/elife-84613-supp3-v2.xlsx
-
Supplementary file 4
Mouse cell clusters annotations.
- https://cdn.elifesciences.org/articles/84613/elife-84613-supp4-v2.xlsx
-
Supplementary file 5
Matching cell types between human and mouse tissues.
- https://cdn.elifesciences.org/articles/84613/elife-84613-supp5-v2.xlsx
-
Supplementary file 6
Diseases and their PrEDiCT scores across mouse cell types per tissue.
- https://cdn.elifesciences.org/articles/84613/elife-84613-supp6-v2.xlsx
-
Supplementary file 7
Tissues affected by heritable cancers.
- https://cdn.elifesciences.org/articles/84613/elife-84613-supp7-v2.xlsx
-
Supplementary file 8
Prevalence, susceptibility and classes of cell types.
- https://cdn.elifesciences.org/articles/84613/elife-84613-supp8-v2.xlsx
-
Supplementary file 9
The percentage of cells that express a gene per cell type in human tissues.
- https://cdn.elifesciences.org/articles/84613/elife-84613-supp9-v2.xlsx
-
Supplementary file 10
The percentage of cells that express a gene per cell type in mouse tissues.
- https://cdn.elifesciences.org/articles/84613/elife-84613-supp10-v2.xlsx
-
Supplementary file 11
The preferential expression of genes in cell types of human tissues.
- https://cdn.elifesciences.org/articles/84613/elife-84613-supp11-v2.xlsx
-
Supplementary file 12
The preferential expression of genes in cell types of mouse tissues.
- https://cdn.elifesciences.org/articles/84613/elife-84613-supp12-v2.xlsx
-
Supplementary file 13
Summary of PrEDiCT scores.
- https://cdn.elifesciences.org/articles/84613/elife-84613-supp13-v2.xlsx
-
MDAR checklist
- https://cdn.elifesciences.org/articles/84613/elife-84613-mdarchecklist1-v2.pdf