Motion Processing: How the brain stays in sync with the real world
In professional baseball the batter has to hit a ball that can be travelling as fast as 170 kilometers per hour. Part of the challenge is that the batter only has access to outdated information: it takes the brain about 80–100 milliseconds to process visual information, during which time the baseball will have moved about 4.5 meters closer to the batter (Allison et al., 1994; Thorpe et al., 1996). This should make it virtually impossible to consistently hit the baseball, but the batters in Major League Baseball manage to do so about 90% of the time. How is this possible?
Fortunately, baseballs and other objects in our world are governed by the laws of physics, so it is usually possible to predict their trajectories. It has been proposed that the brain can work out where a moving object is in almost real time by exploiting this predictability to compensate for the delays caused by processing (Hogendoorn and Burkitt, 2019; Kiebel et al., 2008; Nijhawan, 1994). However, it has not been clear how the brain might be able to do this.
Since predictions must be made within a matter of milliseconds, highly time-sensitive methods are needed to study this process. Previous experiments were unsuccessful in determining the exact timing of brain activity (Wang et al., 2014). Now, in eLife, Philippa Anne Johnson and colleagues at the University of Melbourne and the University of Amsterdam report new insights into motion processing (Johnson et al., 2023).
Johnson et al. used a combination of electroencephalogram (EEG) recordings and pattern recognition algorithms to investigate how long it took participants to process the location of objects that either flashed in one place (static objects) or moved in a straight line (moving objects). Using machine learning techniques, Johnson et al. first identified how the brain represents a non-moving object (Grootswagers et al., 2017). They accurately mapped patterns of neural activity, which corresponded to the location of the static object during the experiment. Participants took about 80 milliseconds to process this information (Figure 1).
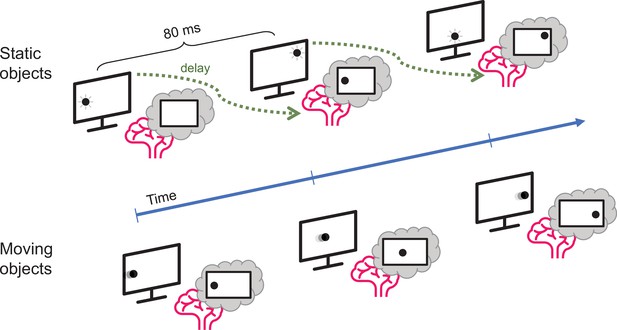
Motion processing in the human brain.
Johnson et al. compared how long it takes the brain to process visual information about static objects and moving objects. The static objects (top) did not move but were briefly shown in unpredictable locations on the screen: the delay between the appearance of the object and the representation of its location in the brain was about 80 milliseconds. However, when the object moved in a predictable manner (bottom), the delay was much smaller.
Strikingly, Johnson et al. discovered that the brain represented the moving object at location different to where one would expect it to be (i.e., not at the location from 80ms ago). Instead, the internal representation of the moving object was aligned to its actual current location so that the brain was able to track moving objects in real time. The visual system must therefore be able to correct the position by at least 80 milliseconds worth of movement, indicating that the brain can effectively compensate for temporal processing delays by predicting (or extrapolating) where a moving object will be located in the future.
To fully grasp how motion prediction processes compensate for the lag between the external world and the brain, it is important to know where in the visual system this compensatory mechanism occurs. Johnson et al. showed that the delay was already fully compensated for in the visual cortex, indicating that the compensation happens early during visual processing. There is evidence to suggest that some degree of motion prediction occurs in the retina, but Johnson et al. argue that this on its own is not enough to fully compensate for the delays caused by neural processing (Berry et al., 1999).
Another possibility is that a brain area involved in a later stage of motion perception, called the middle temporal area, may also play a role in predicting the location of an object (Maus et al., 2013). This region is thought to provide predictive feedback signals that help to compensate for the neural processing delay between the real world and the brain (Hogendoorn and Burkitt, 2019). More research is needed to test this theory, for example, by directly recording neurons in the middle temporal area in primates and rodents using intracranial electrodes. Gaining access to such accurate spatial and temporal neural information might be key to identifying where predictions are made and what they foresee exactly.
The work of Johnson et al. confirms that motion prediction of around 80–100 milliseconds can almost completely compensate for the lag between events in the real world and their internal representation in the brain. As such, humans are able to react to incredibly fast events – if they are predictable, like a baseball thrown at a batter. Neural delays need to be accounted for in all types of information processing within the brain, including the planning and execution of movements. A deeper understanding of such compensatory processes will ultimately help us to understand how the human brain can cope with a fast world, while the speed of its internal signaling is limited. The evidence here seems to suggest that we overcome these neural delays during motion perception by living in our brain’s prediction of the present.
References
-
Decoding dynamic brain patterns from evoked responses: a tutorial on multivariate pattern analysis applied to time series neuroimaging dataJournal of Cognitive Neuroscience 29:677–697.https://doi.org/10.1162/jocn_a_01068
-
A hierarchy of time-scales and the brainPLOS Computational Biology 4:e1000209.https://doi.org/10.1371/journal.pcbi.1000209
-
Motion direction biases and decoding in human visual cortexThe Journal of Neuroscience 34:12601–12615.https://doi.org/10.1523/JNEUROSCI.1034-14.2014
Article and author information
Author details
Publication history
Copyright
© 2023, Koevoet, Sahakian et al.
This article is distributed under the terms of the Creative Commons Attribution License, which permits unrestricted use and redistribution provided that the original author and source are credited.
Metrics
-
- 1,900
- views
-
- 127
- downloads
-
- 0
- citations
Views, downloads and citations are aggregated across all versions of this paper published by eLife.
Download links
Downloads (link to download the article as PDF)
Open citations (links to open the citations from this article in various online reference manager services)
Cite this article (links to download the citations from this article in formats compatible with various reference manager tools)
Further reading
-
- Neuroscience
Data-driven models of neurons and circuits are important for understanding how the properties of membrane conductances, synapses, dendrites, and the anatomical connectivity between neurons generate the complex dynamical behaviors of brain circuits in health and disease. However, the inherent complexity of these biological processes makes the construction and reuse of biologically detailed models challenging. A wide range of tools have been developed to aid their construction and simulation, but differences in design and internal representation act as technical barriers to those who wish to use data-driven models in their research workflows. NeuroML, a model description language for computational neuroscience, was developed to address this fragmentation in modeling tools. Since its inception, NeuroML has evolved into a mature community standard that encompasses a wide range of model types and approaches in computational neuroscience. It has enabled the development of a large ecosystem of interoperable open-source software tools for the creation, visualization, validation, and simulation of data-driven models. Here, we describe how the NeuroML ecosystem can be incorporated into research workflows to simplify the construction, testing, and analysis of standardized models of neural systems, and supports the FAIR (Findability, Accessibility, Interoperability, and Reusability) principles, thus promoting open, transparent and reproducible science.
-
- Neuroscience
The central amygdala (CeA) has emerged as an important brain region for regulating both negative (fear and anxiety) and positive (reward) affective behaviors. The CeA has been proposed to encode affective information in the form of valence (whether the stimulus is good or bad) or salience (how significant is the stimulus), but the extent to which these two types of stimulus representation occur in the CeA is not known. Here, we used single cell calcium imaging in mice during appetitive and aversive conditioning and found that majority of CeA neurons (~65%) encode the valence of the unconditioned stimulus (US) with a smaller subset of cells (~15%) encoding the salience of the US. Valence and salience encoding of the conditioned stimulus (CS) was also observed, albeit to a lesser extent. These findings show that the CeA is a site of convergence for encoding oppositely valenced US information.