A retrospective cohort study of Paxlovid efficacy depending on treatment time in hospitalized COVID-19 patients
Abstract
Paxlovid, a SARS-CoV-2 antiviral, not only prevents severe illness but also curtails viral shedding, lowering transmission risks from treated patients. By fitting a mathematical model of within-host Omicron viral dynamics to electronic health records data from 208 hospitalized patients in Hong Kong, we estimate that Paxlovid can inhibit over 90% of viral replication. However, its effectiveness critically depends on the timing of treatment. If treatment is initiated three days after symptoms first appear, we estimate a 17% chance of a post-treatment viral rebound and a 12% (95% CI: 0–16%) reduction in overall infectiousness for non-rebound cases. Earlier treatment significantly elevates the risk of rebound without further reducing infectiousness, whereas starting beyond five days reduces its efficacy in curbing peak viral shedding. Among the 104 patients who received Paxlovid, 62% began treatment within an optimal three-to-five-day day window after symptoms appeared. Our findings indicate that broader global access to Paxlovid, coupled with appropriately timed treatment, can mitigate the severity and transmission of SARS-Cov-2.
Editor's evaluation
This study presents a valuable model-based analysis of how time to treatment post-symptom onset may influence Paxlovid efficacy in hospitalised COVID-19 patients. The analysis, based on a large data set, provides information on the action of the drug and supports clinical decision-making. Furthermore, it provides solid evidence for the role of the drug in reducing infectiousness in those receiving treatment.
https://doi.org/10.7554/eLife.89801.sa0Introduction
The severe acute respiratory syndrome coronavirus 2 (SARS-CoV-2) first appeared in Wuhan, China in late 2019 and quickly expanded into a global pandemic (Wan, 2020). As of March 2023, the COVID-19 pandemic has caused over 605 million reported infections, 6.8 million deaths, and substantial socioeconomic damage worldwide (Mathieu et al., 2020). Throughout the pandemic, vaccines (Basta et al., 2020) and a variety of non-pharmaceutical interventions have been widely used to mitigate transmission and avert severe clinical outcomes (Lai et al., 2020). However, the premature relaxation of restrictions, contradictory messaging, and erosion of public adherence have undermined these efforts (Han et al., 2020). Numerous variants of interest (VOI) and variants of concern (VOC) (World Health Organization, 2020) have emerged, spreading more quickly than the ancestral strain (CDC, 2021) and evading vaccine-induced and infection-induced immunity (Wang et al., 2021).
The race to develop SARS-CoV-2 antiviral drugs has yielded at least 36 therapeutics (Zimmer et al., 2020). In December of 2021, the US Food and Drug Administration (FDA) issued emergency use authorization for both molnupiravir (a small-molecule ribonucleoside prodrug of N-hydroxycytidine) and Paxlovid (a combination of nirmatrelvir, an inhibitor of the SARS-CoV-2 main protease, and ritonavir, an HIV-1 protease inhibitor and CYP3A inhibitor; Zimmer et al., 2020). Clinical studies suggest that outpatient treatment of high-risk symptomatic adult patients with molnupiravir and Paxlovid within 5 days after symptom onset could reduce the hospitalization risk by 30% (US Food and Drug Administration, 2022) and 88% (US Food and Drug Administration, 2021), respectively. In May of 2022, the US launched a national Test-to-Treat program to facilitate rapid administration of these two oral antivirals through pharmacies, health clinics, and telehealth providers (The White House, 2022). In March 2022, the Hong Kong Hospital Authority (HKHA) began regularly treating COVID-19 inpatients with molnupiravir and Paxlovid (Leung et al., 2022), which substantially reduced their risks of progression to severe disease and death (Wong et al., 2022).
Drugs that suppress viral replication not only improve patient outcomes but may also reduce infectiousness to others. Such antivirals can thus be deployed on a population scale to curb pandemic waves, either as a complement to or a replacement of socioeconomically costly measures such as travel restrictions and stay-home orders. A prior study of Baloxavir, a drug that suppresses influenza replication, demonstrates that administration to 30% of infected cases within 48 hr after symptom onset would typically avert over six thousand deaths and 22 million infections in the US during a typical epidemic season (Du et al., 2020).
Clinical trial data for Paxlovid suggests that it may similarly curtail SARS-CoV-2 viral replication, if administered shortly after symptoms appear (Wong et al., 2022). Here, we estimate the impact of the timing of Paxlovid treatment on viral load, using a within-host mathematical model of Omicron replication fitted to viral titer data for cohorts of COVID-19 inpatients in Hong Kong who either did or did not receive Paxlovid treatment.
We analyzed HKHA electronic health records (EHR) for 208 SARS-CoV-2 positive patients between ages 8 and 103 who were hospitalized for mild to moderate illness between January 6 and May 1, 2022, when the Omicron BA.2 was the dominant variant in Hong Kong. The EHR data includes age, sex, vaccination history, drug prescriptions, symptoms, and daily viral titer measurements starting from the fourth day after symptom onset (Materials and methods). To construct cohorts, we first identified 104 COVID-19 mild-to-moderate patients who received Paxlovid treatment without oxygen therapy. We then used propensity score matching to select 104 patients who were not treated with Paxlovid or molnupiravir.
Results
By fitting a mathematical model of SARS-CoV-2 kinetics within a single patient to the viral load measurements, we estimate the rates at which viral particles infect susceptible host cells (β), infected cells are cleared (δ), and infected cells release viral particles (π), as well as the maximum efficacy of Paxlovid for reducing the replication rate of SARS-CoV-2 viruses (0.91) (National Library of Medicine (U.S.), 2023; Hammond et al., 2022; Supplementary file 1, Supplementary file 2). The fitted model simulates viral load trajectories that mirror the observed data in the treated and untreated cohorts (Figure 1A and B, Figure 1—figure supplement 1).
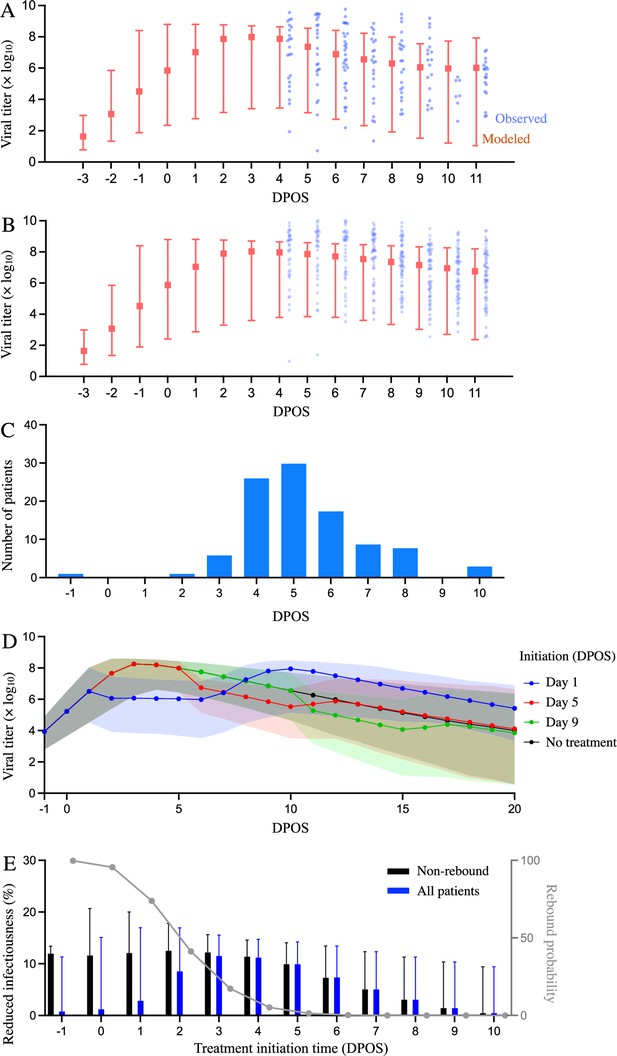
Estimated efficacy of Paxlovid for suppressing SARS-CoV-2 viral load depending on timing of treatment.
Observed and model estimated viral load kinetics for each day post onset of symptoms (DPOS) for hospitalized COVID-19 patients who (A) received Paxlovid treatment (N=104) or (B) did not receive antiviral treatment (N=104) between 6 January and 1 May 2022 in Hong Kong. Blue points correspond to actual individual patients; red squares and error bars indicate medians and 95% interpercentile ranges across simulated patients. Day zero corresponds to the first day of symptoms. (C) Distribution of treatment initiation times for the 104 patients who received Paxlovid (Supplementary file 4). (D) Estimated patient viral loads for three different Paxlovid treatment initiation times. Points and shading represent the estimated medians and 95% interpercentile ranges across 1000 simulations. (E) Chance of a post-treatment rebound (gray line, right y-axis) and expected reduction in infectiousness across all patients (blue bars, left y-axis) and patients who do not experience a rebound (black bars, left y-axis), depending on the timing of treatment initiation. Rebound probabilities are estimated by the fraction out of 1000 simulations in which the viral titer reached higher values after treatment than before treatment. Reduced infectiousness is estimated by comparing the areas under the estimated infectiousness curves for untreated versus treated patients. Bars and whiskers indicate medians and 95% interpercentile ranges across 1000 pairwise comparisons.
Of the 104 patients who received Paxlovid, 63% initiated treatment within five days post the onset of symptoms (DPOS) (Figure 1C). As we vary the treatment initiation time from one to nine DPOS, the model projects precipitous drops in viral load within the first 24 hr of receiving Paxlovid (Figure 1D). If treatment is initiated the day after symptoms appear, we estimate a 74% chance that viral growth will rebound substantially post treatment (Figure 1E, Supplementary file 3), peaking around 10 DPOS. For more delayed treatment schedules, the probability and magnitude of rebounds are lower. Patients who initiate treatment three or five DPOS have an estimated 17% or 1% chance of a rebound, respectively. The estimated overall reduction in infectiousness for patients who do not experience rebounds declines from 12% (95% CI: 0%, 16%) for patients who start treatment three DPOS to 0% (95% CI: 0%, 9%) for patients starting treatment 10 DPOS (Figure 1E, Supplementary file 3).
Discussion
Using a within-host model of viral kinetics, we estimated the efficacy of Paxlovid for reducing SARS-CoV-2 viral load for mild-to-moderate COVID-19 patients during the early 2022 Omicron wave in Hong Kong. Rapid reductions in viral load may not only benefit the patient, but also indirectly protect household members and others who come in contact with them. Recent studies suggest a logit-linear relationship between viral load and infectiousness (Marc et al., 2021). Thus broad and rapid administration of Paxlovid, to even mild and moderate cases, may be a logistically and economically viable strategy for slowing transmission on a community scale in comparison with socioeconomically burdensome social distancing measures. To this end, the U.S. Test-to-Treat program is designed so that 90% of Americans can access antivirals within five miles of their residence (The White House, 2022). However, uptake in the US has been relatively low, with only 11% COVID-19 cases receiving antiviral prescriptions between May and early July of 2022 (Kulke, 2024). The low uptake may stem from slow rollouts in some areas, complex eligibility requirements, testing, and potential drug interactions (Erman, 2022), as well as concerns about viral rebounds following Paxlovid treatment (Callaway, 2022), which our model reproduces when we assume that antiviral efficacy declines after the 5-day course of treatment (Figure 1D).
Access to Paxlovid may be even more vital in Hong Kong, which suffered the world’s highest death rate during the March and April 2022 Omicron wave. At the time, only 51% of individuals over age 80 and 76% older of individuals between 70 and 80 years had received at least one dose of vaccine (Mathieu et al., 2020). Although Paxlovid is known to be life-saving, under 40% of infected COVID-19 cases in Hong Kong over age 60 have received the drug by late July 2022 (Liu, 2022). Low rates of antiviral uptake may stem from misinformation, lack of access, and the rising proportion of cases that opt for at-home rapid tests and do not seek healthcare (Cheung et al., 2022; Kasakove, 2021). Telemedicine and online healthcare services can accelerate and expand access to antivirals (Centers for Disease Control and Prevention, 2024), but may not reach some of the older populations in Hong Kong.
Our parameter estimates for the Omicron variant are generally comparable to prior estimates based on viral titers measured in patients infected with the ancestor strain in 2020 (Ke et al., 2021). However, our estimate for the effect of innate immunity at suppressing viral replication rate is significantly lower than the prior estimate, which is consistent with a recent study suggesting that Omicron variants may be more immune evasive than ancestral variants (Willett et al., 2022). As further validation of our estimates, we compare projections of the fitted model with observed viral titers from 208 patients (Figure 1—figure supplement 1) and demonstrate that model can reproduce the rebounds experienced by some COVID-19 patients following Paxlovid treatment, under the assumption that Paxlovid efficacy begins to decline after the fifth day of treatment (Figure 1D). Our model of SARS-CoV-2 viral load dynamics following Paxlovid treatment allows us to estimate the potential benefits of early treatment for reducing infectiousness, while accounting for variation across patients and potential rebounds in viral growth. The model projections corroborate prior estimates for the impact of treatment initiation time on the duration of viral shedding (Wang et al., 2023).
Our analysis is limited by several model assumptions. First, we do not consider possible variation in viral measurements by specimen types (e.g., the deep throat saliva, sputum, throat swab, nasal swab, combined nasal and throat swab, nasopharyngeal swab Centre for Health Protection, 2021), which are not provided in the data analyzed. Second, we do not stratify our estimates by patient age group, risk group, or vaccination status, which could impact both intrinsic viral kinetics and drug efficacy (Puhach et al., 2022). Third, we do not consider the possible emergence of Paxlovid-resistant viruses, which could significantly reduce drug efficacy. Laboratory studies have identified amino acid substitutions (Jochmans et al., 2022; Zhou et al., 2022) that could confer resistance. Although such variants have not yet been found in clinical trials, broad use of Paxlovid could spur the emergence of drug-resistance, as has been documented for the SARS-CoV-2 antiviral Remdesivir (Gandhi et al., 2022).
In conclusion, fast-acting antiviral drugs like Paxlovid have the potential to reduce SARS-CoV-2 transmission while improving patient outcomes. The development of global distribution programs that provide rapid and equitable access could enhance our ability to combat COVID-19 as the virus and the landscape of immunity continue to evolve.
Materials and methods
Data
We analyzed electronic health records of hospitalized Hong Kong Hospital Authority patients between 8 and 103 years of age who were COVID-19 positive but did not receive oxygen therapy, between January 6, 2022 and May 1, 2022 (Figure 2). COVID-19 status was determined by a transcription-polymerase chain reaction (RT-qPCR) test or a rapid antigen test. Each patient record includes demographic information, drug administration data, symptoms, laboratory test results, and daily viral titer measurements (i.e. RT-qPCR cycle threshold (Ct) values) between 8 and 15 days post symptom onset. We convert Ct values to viral load as given by log10(Viral load [copies/mL])=–0.32 Ct+14.11 (Jeong et al., 2021; Peiris et al., 2003).
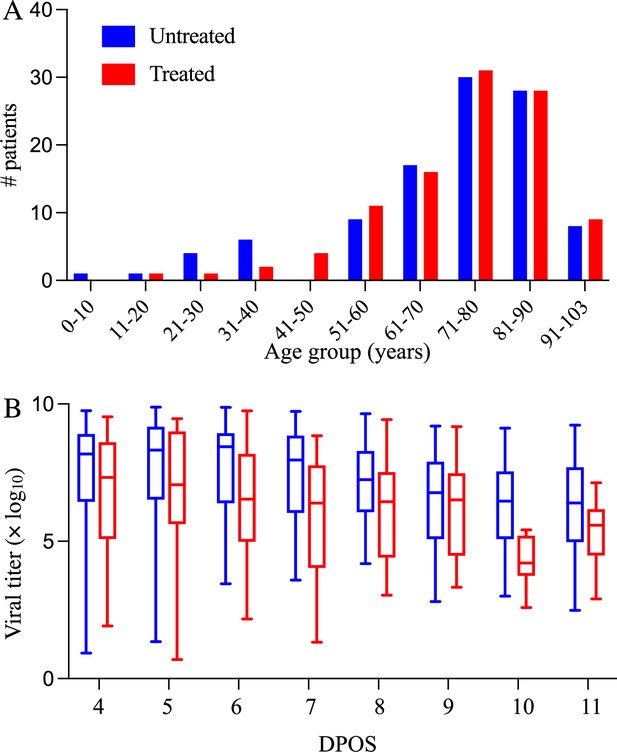
Demographic and virologic characteristics of the 208 hospitalized COVID-19 patients in the study.
The distribution of (A) ages and (B) daily viral titers across the 104 hospitalized COVID-19 patients who received Paxlovid treatment (red) and the 104 patients who did not receive any antiviral drug (red) between January 6 and May 1, 2022 in Hong Kong. The box plots in panel B indicate the median, interquartile range, and range of the observed viral titers across patients for each day after symptom onset (DPOS). Day zero corresponds to the first day that symptoms occur.
To select cohorts of patients with mild-to-moderate illness, we classify patients according to their daily reported clinical conditions as follows: (1) critical: in intensive care unit, intubated, in shock, or require extracorporeal membrane oxygenation; (2) serious: require oxygen supplement of 3 L or more per minute; (3) stable: mild influenza-like illness symptoms; (4) satisfactory: progressing well and likely to be discharged soon. We select only patients who maintain stable or satisfactory levels throughout their hospital stay.
Cohort selection
Request a detailed protocolAfter identifying 104 patients who received Paxlovid but not oxygen therapy in the data set, we used propensity score matching to create a cohort of 104 other patients who received neither an antiviral drug (Paxlovid or molnupiravir) nor oxygen therapy. We matched based on age group (5~17, 18~50, 51~65, >65), gender, and vaccination status (i.e. fully vaccinated or not).
Within-host model of SARS-CoV-2 viral kinetics and antiviral treatment
Request a detailed protocolWithin a given host i, susceptible cells (Ui) can be infected by active viruses Vi at a rate and thereby transition to cells in the eclipse phase (Ei) and infected cells (Ii), according to the following system of equations by including a prototypical innate response (e.g. type-I interferon) (Ke et al., 2021) that makes cells refractory to viral infection (Ri):
where the death rate and the replication rates of infected cells are and , respectively, and the viral death rate is c. The interferon-induced conversion of target cells to refractory cells has the rate Φ. And the rate at which refractory cells become target cells again is ρ. The antiviral efficacy is , which is the rate at which the drug inhibits the replication of infected cells. The initial number of infected cells (E0),the initial number of target cells (U0), the virus clearance rate c, and the rate of the eclipse phase are fixed as 1 cell, 8×107 cells, 10 per day, and 4 per day, respectively (Ke et al., 2021). We incorporate a gradual decline in Paxlovid efficacy following a five-day course of treatment, using a pharmacokinetic model introduced in a recent study of Paxlovid rebound dynamics. Our model assumes that Paxlovid efficacy () is 0 prior to the first dose and then given by:
where is the time elapsed since receiving the first dose and is the maximum antiviral effectiveness, which we estimate by fitting the model to clinical trial data. EC50 is the concentration at which the drug effectiveness is half-maximal (62 nM) (US Food and Drug Administration, 2021); is the product of the bioavailability of the drug and the mass of the drug administered in a dose per volume (6.25×103 nM) (US Food and Drug Administration, 2021; National Center for Biotechnology Information, 2024); is the number of doses administered in the period up to time ; is the elimination rate (2.8 /day) (US Food and Drug Administration, 2021); is the absorption rate (17.5 /day) (Dixit and Perelson, 2004; Perelson et al., 2023); is the dosing interval (1/2 day) (Dixit and Perelson, 2004; Perelson et al., 2023).
The incubation period of the SARS-CoV-2 Omicron variant is estimated to be 3.6 days (Du et al., 2022). We calibrate parameters in the model using a nonlinear mixed-effect model with both the fixed effect (population scale) and random effect (individual scale) in software MONOLIX 2021R1 (Traynard et al., 2020). Fixed effects are population parameters that govern all patients and random effects are variable across individuals. To estimate the six model parameters governing the viral load dynamics, we fitted the within-host model to the observed SARS-Cov-2 RNA titer (log10 copies/mL) measured across 208 patients adults treated with or without Paxlovid between January 6, 2022 and May 1, 2022. We used the Stochastic Approximation Expectation-Maximization (SAEM) algorithm to estimate these parameters (Miao et al., 2011; Traynard et al., 2020) assuming fixed values for the initial numbers of infecting virions and susceptible target cells following Ke et al., 2021 and confirmed the convergence of the estimates via trace plots. The SAEM algorithm is an established method in population pharmacology modeling with clear convergence indicators (Delyon et al., 1999; Population parameter using SAEM algorithm, 2016).
Incorporating uncertainty into viral load projections
Request a detailed protocolWe use the within-host model to simulate the viral load trajectories of patients under various treatment scenarios. For each simulation, we randomly select parameter values from triangular distributions with modes and ranges set to the medians and 95% CI estimated from the clinical data (Supplementary file 1).
To estimate the impact of treatment time on viral load dynamics (Figure 1D) and infectiousness (Figure 1E), we compare pairs of model simulations (with versus without Paxlovid treatment). Each pair shares the same randomly-generated parameter values, including an incubation period randomly selected from a previously-estimated distribution ( days) (Du et al., 2022).
In order to translate differences in the simulated viral titers into differences in infectiousness, we used the following published model relating household transmission risk to viral load (Marc et al., 2021).
where denotes the probability that individual i infects a susceptible household member at time, =–2.94, which corresponds to a baseline probability of transmission of 5% (Marc et al., 2021), and β = 0.49 (Marc et al., 2021). We assume that the relative infectiousness of a patient throughout their infection can be approximated by the area under the household infectivity curve from the time of infection () until 15 days post the onset of symptoms (), as given by
For a given pair of simulations (i), we then compare the projected infectiousness of the treated patient to that of the untreated patient , as given by
For each treatment initiation time considered, we report the median and 95% confidence intervals of the reduction in infectiousness (Δ) based on 1000 pairs of simulations.
Data availability
All data used in this study can be accessed through Github: https://github.com/ZhanweiDU/PaxHK/.
References
-
WebsiteInfection Control Advice on Specimen Collection to Test for COVID-19Accessed June 13, 2022.
-
Lessons learned from the fifth wave of COVID-19 in Hong Kong in early 2022Emerging Microbes & Infections 11:1072–1078.https://doi.org/10.1080/22221751.2022.2060137
-
Convergence of a stochastic approximation version of the EM algorithmThe Annals of Statistics 27:94–128.https://doi.org/10.1214/aos/1018031103
-
Complex patterns of viral load decay under antiretroviral therapy: influence of pharmacokinetics and intracellular delayJournal of Theoretical Biology 226:95–109.https://doi.org/10.1016/j.jtbi.2003.09.002
-
Modeling mitigation of influenza epidemics by baloxavirNature Communications 11:2750.https://doi.org/10.1038/s41467-020-16585-y
-
Shorter serial intervals and incubation periods in SARS-CoV-2 variants than the SARS-CoV-2 ancestral strainJournal of Travel Medicine 29:taac052.https://doi.org/10.1093/jtm/taac052
-
Oral Nirmatrelvir for high-risk, nonhospitalized adults with Covid-19The New England Journal of Medicine 386:1397–1408.https://doi.org/10.1056/NEJMoa2118542
-
The allocation of COVID-19 vaccines and antivirals against emerging SARS-CoV-2 variants of concern in East Asia and Pacific region: A modelling studyThe Lancet Regional Health. Western Pacific 21:100389.https://doi.org/10.1016/j.lanwpc.2022.100389
-
On identifiability of nonlinear ode models and applications in viral dynamicsSIAM Review. Society for Industrial and Applied Mathematics 53:3–39.https://doi.org/10.1137/090757009
-
WebsiteFACT SHEET: Biden administration announces launch of first federally-supported test to treat siteThe White House. Accessed January 20, 2023.
-
WebsiteFact sheet for healthcare providers: emergency use authorization for PaxlovidAccessed August 27, 2022.
-
SARS-CoV-2 Omicron is an immune escape variant with an altered cell entry pathwayNature Microbiology 7:1161–1179.https://doi.org/10.1038/s41564-022-01143-7
-
Real-world effectiveness of early molnupiravir or nirmatrelvir-ritonavir in hospitalised patients with COVID-19 without supplemental oxygen requirement on admission during Hong Kong’s omicron BA.2 wave: a retrospective cohort studyThe Lancet. Infectious Diseases 22:1681–1693.https://doi.org/10.1016/S1473-3099(22)00507-2
Article and author information
Author details
Funding
Innovation and Technology Commission - Hong Kong (AIR@InnoHK)
- Zhanwei Du
Health and Medical Research Fund (07210147)
- Zhanwei Du
National Natural Science Foundation of China (82304204)
- Yuan Bai
National Institutes of Health (AI151176)
- Lauren A Meyers
Centers for Disease Control and Prevention (U01IP001136)
- Lauren A Meyers
The funders had no role in study design, data collection and interpretation, or the decision to submit the work for publication.
Acknowledgements
Individual patient-informed consent was not required in this study using anonymized data. Financial support was provided by the AIR@InnoHK Programme from Innovation and Technology Commission of the Government of the Hong Kong Special Administrative Region, Health and Medical Research Fund Research Fellowship Scheme, Food and Health Bureau, Government of the Hong Kong Special Administrative Region (grant no. 07210147), National Natural Science Foundation of China (grant no. 82304204), the US National Institutes of Health (grant no. R01 AI151176), the Centers for Disease Control and Prevention COVID (grant no. U01IP001136).
Ethics
Human subjects: Individual patient-informed consent was not required in this study using anonymized data.
Copyright
© 2024, Du, Wang, Bai et al.
This article is distributed under the terms of the Creative Commons Attribution License, which permits unrestricted use and redistribution provided that the original author and source are credited.
Metrics
-
- 1,857
- views
-
- 229
- downloads
-
- 3
- citations
Views, downloads and citations are aggregated across all versions of this paper published by eLife.
Download links
Downloads (link to download the article as PDF)
Open citations (links to open the citations from this article in various online reference manager services)
Cite this article (links to download the citations from this article in formats compatible with various reference manager tools)
Further reading
-
- Epidemiology and Global Health
- Microbiology and Infectious Disease
Background:
In many settings, a large fraction of the population has both been vaccinated against and infected by severe acute respiratory syndrome coronavirus 2 (SARS-CoV-2). Hence, quantifying the protection provided by post-infection vaccination has become critical for policy. We aimed to estimate the protective effect against SARS-CoV-2 reinfection of an additional vaccine dose after an initial Omicron variant infection.
Methods:
We report a retrospective, population-based cohort study performed in Shanghai, China, using electronic databases with information on SARS-CoV-2 infections and vaccination history. We compared reinfection incidence by post-infection vaccination status in individuals initially infected during the April–May 2022 Omicron variant surge in Shanghai and who had been vaccinated before that period. Cox models were fit to estimate adjusted hazard ratios (aHRs).
Results:
275,896 individuals were diagnosed with real-time polymerase chain reaction-confirmed SARS-CoV-2 infection in April–May 2022; 199,312/275,896 were included in analyses on the effect of a post-infection vaccine dose. Post-infection vaccination provided protection against reinfection (aHR 0.82; 95% confidence interval 0.79–0.85). For patients who had received one, two, or three vaccine doses before their first infection, hazard ratios for the post-infection vaccination effect were 0.84 (0.76–0.93), 0.87 (0.83–0.90), and 0.96 (0.74–1.23), respectively. Post-infection vaccination within 30 and 90 days before the second Omicron wave provided different degrees of protection (in aHR): 0.51 (0.44–0.58) and 0.67 (0.61–0.74), respectively. Moreover, for all vaccine types, but to different extents, a post-infection dose given to individuals who were fully vaccinated before first infection was protective.
Conclusions:
In previously vaccinated and infected individuals, an additional vaccine dose provided protection against Omicron variant reinfection. These observations will inform future policy decisions on COVID-19 vaccination in China and other countries.
Funding:
This study was funded the Key Discipline Program of Pudong New Area Health System (PWZxk2022-25), the Development and Application of Intelligent Epidemic Surveillance and AI Analysis System (21002411400), the Shanghai Public Health System Construction (GWVI-11.2-XD08), the Shanghai Health Commission Key Disciplines (GWVI-11.1-02), the Shanghai Health Commission Clinical Research Program (20214Y0020), the Shanghai Natural Science Foundation (22ZR1414600), and the Shanghai Young Health Talents Program (2022YQ076).
-
- Epidemiology and Global Health
Background:
The role of circulating metabolites on child development is understudied. We investigated associations between children’s serum metabolome and early childhood development (ECD).
Methods:
Untargeted metabolomics was performed on serum samples of 5004 children aged 6–59 months, a subset of participants from the Brazilian National Survey on Child Nutrition (ENANI-2019). ECD was assessed using the Survey of Well-being of Young Children’s milestones questionnaire. The graded response model was used to estimate developmental age. Developmental quotient (DQ) was calculated as the developmental age divided by chronological age. Partial least square regression selected metabolites with a variable importance projection ≥1. The interaction between significant metabolites and the child’s age was tested.
Results:
Twenty-eight top-ranked metabolites were included in linear regression models adjusted for the child’s nutritional status, diet quality, and infant age. Cresol sulfate (β=–0.07; adjusted-p <0.001), hippuric acid (β=–0.06; adjusted-p <0.001), phenylacetylglutamine (β=–0.06; adjusted-p <0.001), and trimethylamine-N-oxide (β=–0.05; adjusted-p=0.002) showed inverse associations with DQ. We observed opposite directions in the association of DQ for creatinine (for children aged –1 SD: β=–0.05; pP=0.01;+1 SD: β=0.05; p=0.02) and methylhistidine (–1 SD: β = - 0.04; p=0.04;+1 SD: β=0.04; p=0.03).
Conclusions:
Serum biomarkers, including dietary and microbial-derived metabolites involved in the gut-brain axis, may potentially be used to track children at risk for developmental delays.
Funding:
Supported by the Brazilian Ministry of Health and the Brazilian National Research Council.