Robust single-nucleus RNA sequencing reveals depot-specific cell population dynamics in adipose tissue remodeling during obesity
Figures
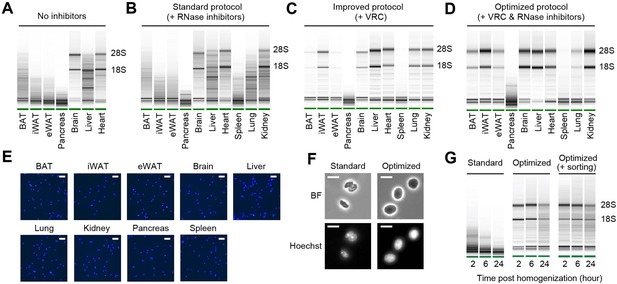
RNA quality and nucleus integrity are preserved by robust nucleus isolation protocols.
(A–D) Analysis of RNA quality in isolated nuclei using Agilent Bioanalyzer. The presence of distinct 18S and 28S bands indicates high RNA quality. Nuclei were isolated from indicated tissue types using different protocols: (A) No RNase inhibitor included, (B) standard protocol with RNase inhibitors (0.4 U/µl), (C) improved protocol with vanadyl ribonucleoside complex (VRC; 10 mM), and (D) optimized protocol using both VRC and RNase inhibitors. (E) Immunofluorescence microscopy images of nucleus suspensions stained with Hoechst (1 µM) from indicated tissue types. Scale bars: 20 µm. (F) Brightfield (BF) and immunofluorescence microscopy images of Hoechst-stained nuclei isolated from epididymal white adipose tissue (eWAT) using the standard and optimized protocols. Scale bars: 10 µm. (G) RNA quality assessed by Agilent Bioanalyzer of isolated nuclei from eWAT stored at 4°C for the indicated duration post homogenization.
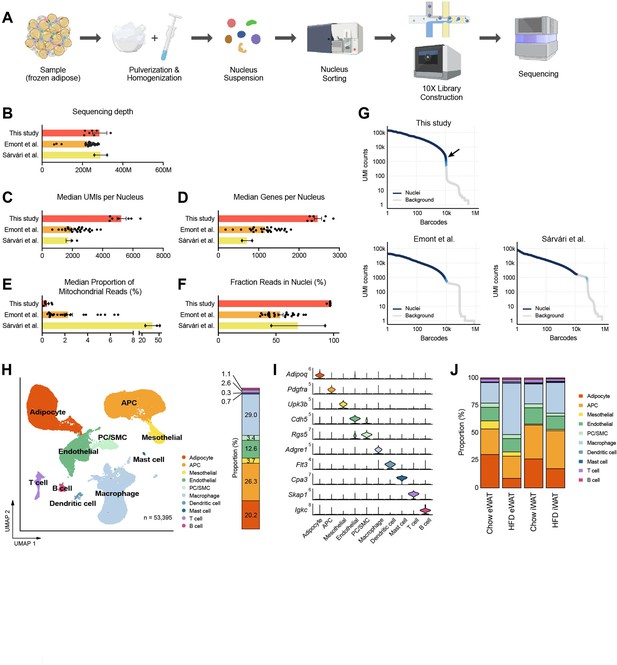
A single-nucleus atlas of white adipose tissue from lean and obese mouse generated by the optimized single-nucleus RNA sequencing (snRNA-seq) protocol.
(A) Schematic of the workflow for the optimized protocol for snRNA-seq of mouse frozen tissue samples. (B–F) Comparison of the quality of snRNA-seq datasets included in this study (n = 7) with those from previous studies (Emont et al., 2022, n = 27; Sárvári et al., 2021, n = 2) using mouse adipose tissue. Bars indicate mean ± SEM, with each dot corresponding to an individual dataset. (B) Sequencing depth, (C) median numbers of unique molecular identifiers (UMIs) detected per nucleus, (D) median number of genes detected per nucleus, (E) median proportion of reads originating from mitochondrial genes, and (F) fraction of reads in nucleus-associated barcodes. (G) Barcode rank plots show the ranks of barcodes based on UMI counts, generated from this study and previous studies (Sárvári et al., 2021; Emont et al., 2022). Darker blue shading indicates a higher proportion of nucleus versus background barcodes. The arrow indicates the steep cliff between nucleus versus background barcodes. (H) Uniform manifold approximation and projection (UMAP) of all 53,395 single nuclei isolated from epididymal white adipose tissue (eWAT) and inguinal white adipose tissue (iWAT) of lean and obese mice. The UMAP-based clustering was performed with a resolution of 0.6, and clusters were annotated as specific cell types with the indicated colors based on their marker genes. The bar on right side displays the overall relative proportions of individual cell types across the samples. (I) Violin plots showing the expression levels of selected marker genes representing each cell type in mouse adipose tissue. (J) Bar graphs showing the relative proportions of individual cell types in adipose tissue by fat depot and diet. APC, adipocyte progenitor cells; PC, pericytes; SMC, smooth muscle cells.
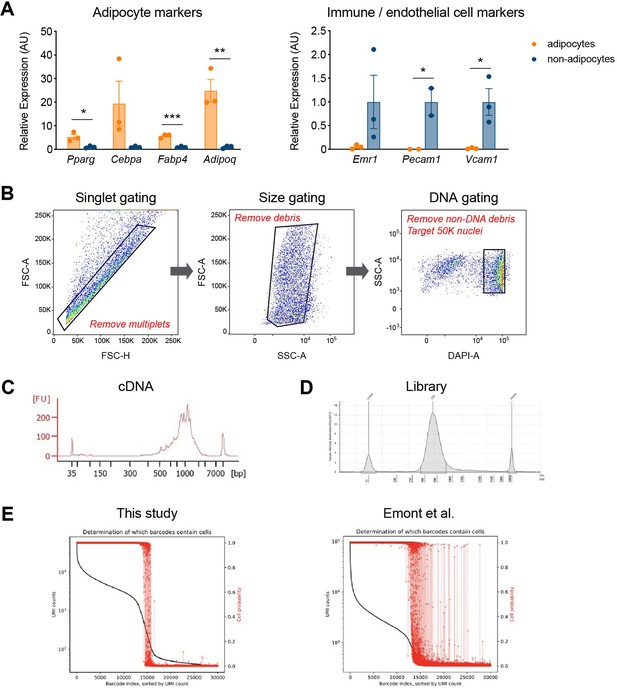
Precise and robust gene expression profiling by our optimized single-nucleus RNA sequencing (snRNA-seq) protocol.
(A) Gene expression analysis of adipocyte and immune/endothelial cell markers in adipocyte and non-adipocyte nuclei (n = 3, each) from epididymal white adipose tissue (eWAT) of NuTRAP mice, utilizing the optimized protocol with vanadyl ribonucleoside complex (VRC). Data are presented as mean ± SEM, and statistical analysis was performed using Student’s t test. *p < 0.05, **p < 0.01, ***p < 0.001. (B) Sequential gating strategies for fluorescence-activated nucleus sorting (FANS) used to sort all Hoechst-positive nuclei from adipose tissue for snRNA-seq. Exemplary profiles of (C) cDNA and (D) sequencing library fragment size distribution, assessed by Agilent Bioanalyzer and TapeStation, respectively. (E) Levels of ambient RNA in the samples of this study (left) and Emont et al., 2022 (GSE176171, right), examined using CellBender. The plots describe both the rank-ordered unique molecular identifier (UMI) counts per droplet (represented by a black line) and the probabilities of each droplet containing a cell (indicated by red dots with dashed lines).
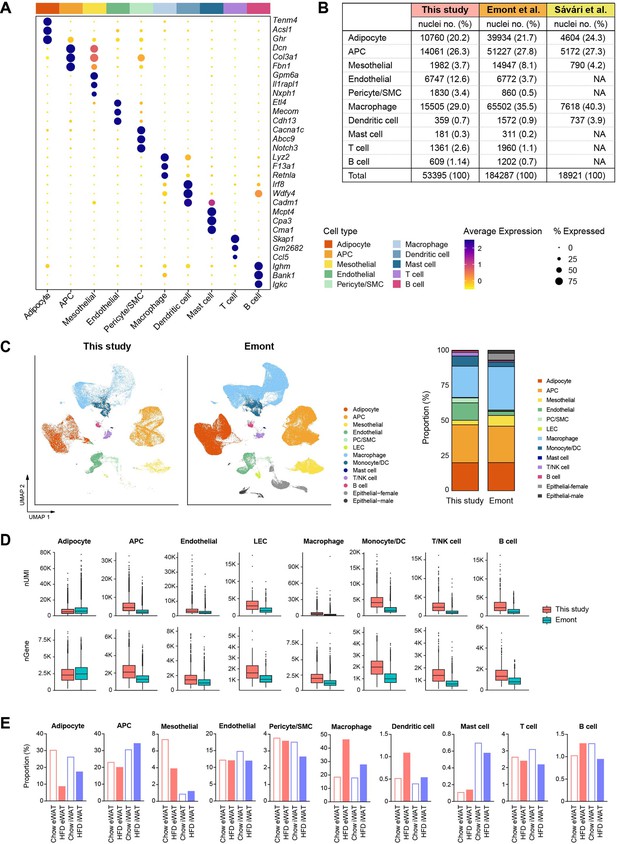
Profile of our single-nucleus atlas encompassing diverse cell populations.
(A) Dot plots showing average scaled expression of the top 3 cell type marker genes in each cell population in mouse adipose tissue. (B–D) Comparison of mouse adipose tissue single-nucleus RNA sequencing (snRNA-seq) data from this study with data from previous snRNA-seq studies (Sárvári et al., 2021; Emont et al., 2022). (B) The absolute numbers and relative proportions of nuclei recovered for each cell type. (C) Integration of all cell types from this study with those from Emont et al., 2022. Bar graphs on the right side display the relative proportions of individual cell types in each dataset. (D) Comparison of unique molecular identifier (UMI) counts and genes detected per nucleus by cell type between this study and Emont et al., 2022. (E) Bar graphs showing the relative proportions of individual cell types by fat depot and diet in this study.
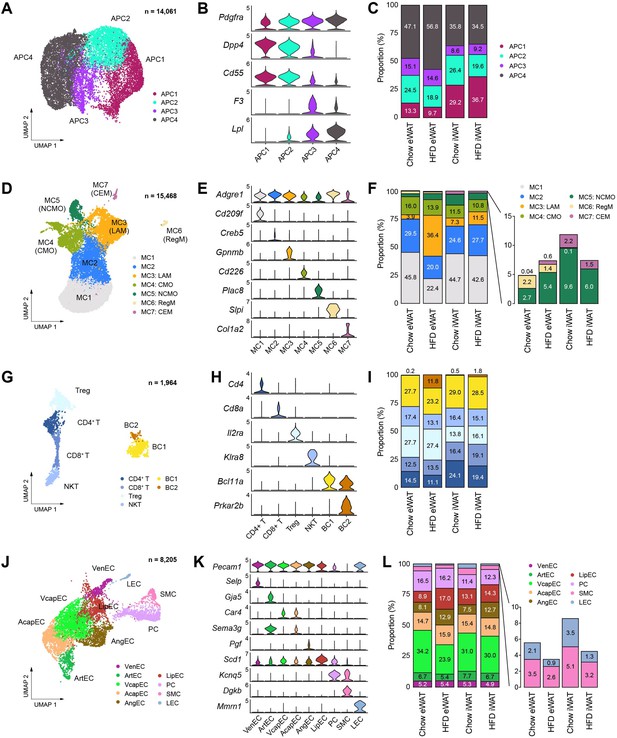
Subpopulations of cell types within the stromal vascular fraction of mouse adipose tissue that vary across fat depot and diet.
(A–C) Identification of adipocyte progenitor cell (APC) subpopulations. (A) Uniform manifold approximation and projection (UMAP), (B) marker genes, and (C) the relative proportions of APC subpopulations (n = 14,061). (D–F) Identification of macrophage/monocyte subpopulations. (D) UMAP projection, (E) marker genes, and (F) the relative proportions of macrophage/monocyte subpopulations (n = 15,468). (G–I) Identification of lymphocyte subpopulations. (G) UMAP projection, (H) marker genes, and (I) the relative proportions of lymphocyte subpopulations (n = 1964). (J–L) Identification of vascular cell subpopulations. (J) UMAP projection, (K) marker genes, and (L) the relative proportions of vascular cell subpopulations (n = 8205). CEM, collagen-expressing macrophages; CMO, classical monocytes; LAM, lipid-laden macrophage; LEC, lymphatic endothelial cells; LipEC, lipid-associated endothelial cells; NCMO, non-classical monocytes; NKT, natural killer T cells; PC, pericytes; RegM, regulatory macrophages; SMC, smooth muscle cells; Treg, regulatory T cells; VcapEC, venous capillary endothelial cells; VenEC, venous endothelial cells.
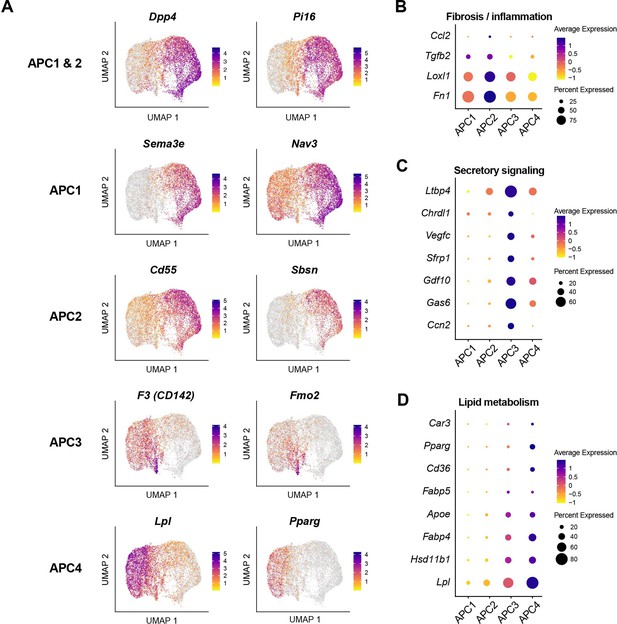
Gene expression profiles of adipocyte progenitor cell (APC) subpopulations indicating distinct functional states.
(A) Uniform manifold approximation and projection (UMAP) visualization of marker genes for APC subpopulations. Dot plots showing the expression of genes associated with fibrosis/inflammation (B), secretory signaling (C), and lipid metabolism (D) across APC subpopulations.

Characterization of diverse immune cell subpopulations.
(A) Uniform manifold approximation and projection (UMAP) visualization of marker genes for macrophage/monocyte subpopulations. (B) Dot plot illustrating pathways enriched within the top 100 marker genes of macrophage/monocyte subpopulations, as determined by clusterProfiler. (C) UMAP visualization of major histocompatibility complex (MHC) genes in macrophage/monocyte subpopulations. (D) Dot plot showing the expression of genes associated with lipid metabolism across macrophage/monocyte subpopulations. (E-F) UMAP visualization of marker genes for lymphocyte subpopulations, including (E) T cells and (F) B cells.
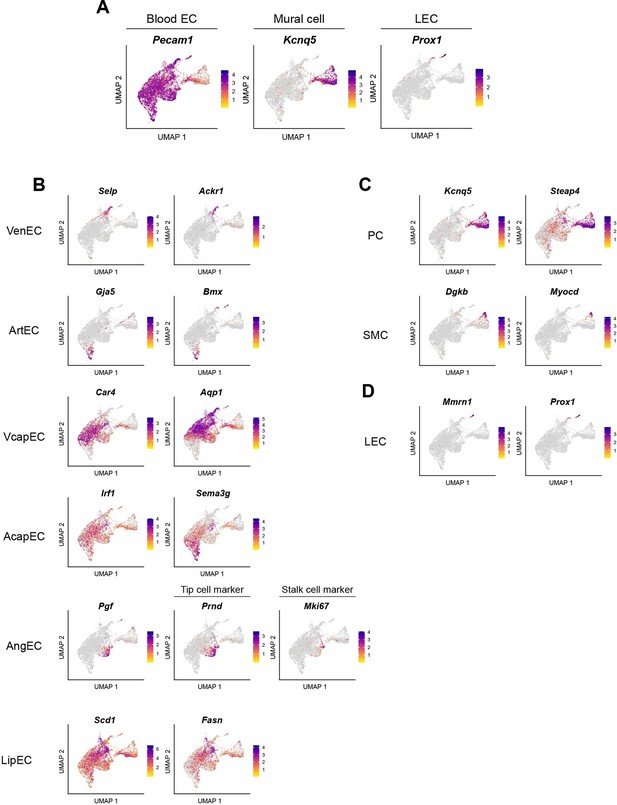
Characterization of diverse vascular cell subpopulations.
(A) Uniform manifold approximation and projection (UMAP) visualization using Pecam1 for blood EC, Kcnq5 for mural cells, and Prox1 for lymphatic endothelial cells (LEC) within vascular cells. UMAP visualization of marker genes for (B) blood EC subpopulations, (C) mural cell subpopulations, and (D) LEC subpopulation.
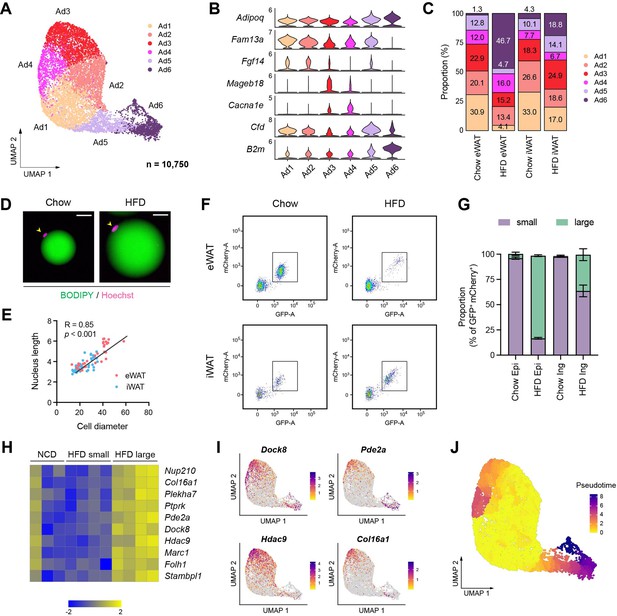
Distinct functional and cellular states of adipocytes affected by obesity.
(A–C) Identification of adipocyte subpopulations. (A) Uniform manifold approximation and projection (UMAP), (B) marker genes, and (C) the relative proportions of adipocyte subpopulations (n = 10,750). (D) Immunofluorescence microscopy images of BODIPY- and Hoechst-stained adipocytes isolated from epididymal white adipose tissue (eWAT) in chow- and high fat diet (HFD)-fed mice. Scale bars: 20 µm. (E) Scatterplot illustrating the correlation between adipocyte size, measured by diameter, and nucleus size, measure by length, in eWAT (red) and inguinal white adipose tissue (iWAT, blue). (F) Scatterplot illustrating the size distribution of mCherry- and GFP-labeled adipocyte nuclei from NuTRAP mice across different fat depots and diets. (G) The relative proportions of small and large nuclei within mCherry- and GFP-labeled adipocyte nuclei across different fat depots and diets. Data are presented as mean ± SEM (n = 4). See Figure 4—figure supplement 1D for gating criteria. (H) Heatmap showing Z-scored expression of hypertrophy signature genes in iWAT adipocyte nuclei from chow-fed mice and in small and large adipocyte nuclei from HFD-fed mice. The signature genes were identified based on enrichment in large versus small adipocyte nuclei in HFD-fed mice. (I) UMAP visualization of select hypertrophy signature genes in adipocytes. (J) Representation of pseudotime within adipocytes, as identified by Monocle3.
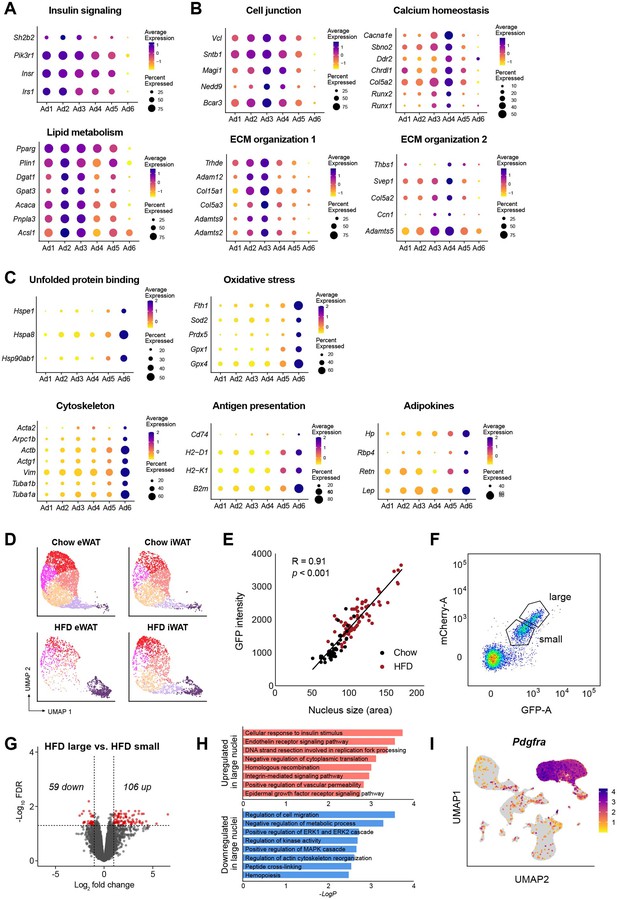
Identification of functional attributes of adipocyte subpopulations.
Dot plots representing the expression of genes linked to distinct biological processes enriched in different adipocyte subpopulations: (A) for Ad1–2, (B) for Ad3–4, and (C) for Ad6. (D) Uniform manifold approximation and projection (UMAP) plots of adipocyte subpopulations by fat depot and diet. (E) Correlation between nucleus size and GFP intensity in adipocyte nuclei isolated from adipose tissues of chow-fed (black) or high fat diet (HFD)-fed (red) Ad-NuTRAP mice. p value was calculated using an F-test with the null hypothesis that the slope is equal to 0. (F) Sorting criteria for gating adipocyte nuclei isolated from inguinal white adipose tissue (iWAT) of HFD-fed NuTRAP mice into small and large nuclei. (G) Volcano plot showing statistical significance (−log10FDR values) versus fold change of gene expression between large and small adipocyte nuclei from iWAT of HFD-fed mice. Red dots represent genes that were differentially expressed between groups with an FDR <0.05 and |log2 fold change| > 1. (H) Bar graphs illustrating the top GO terms (GO: BP) enriched in upregulated (top) and downregulated (bottom) genes in large versus small adipocyte nuclei. (I) UMAP visualization of an adipocyte progenitor cell (APC) marker gene, Pdgfra.
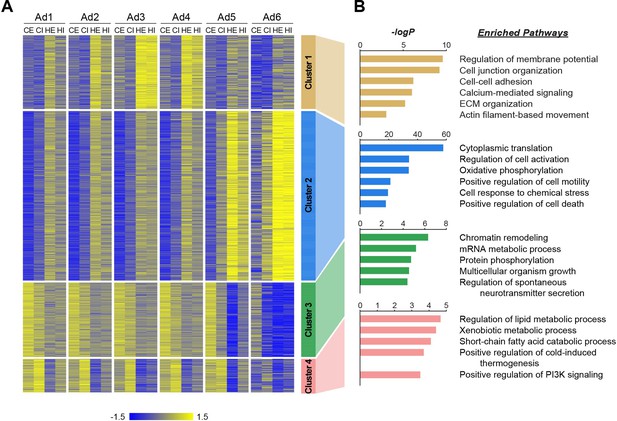
Distinct biological pathways enriched in different adipocyte subpopulations during obesity.
(A) Heatmap illustrating Z-scored expression of high fat diet (HFD)-induced genes in either epididymal white adipose tissue (eWAT) or inguinal white adipose tissue (iWAT) within each adipocyte subpopulation. Those genes are classified into four different clusters by K-means clustering based on their expression patterns across the samples. (B) Top pathways enriched within each cluster of genes with their corresponding −logP values, as determined by Metascape. CE, chow eWAT; CI, chow iWAT; HE, HFD eWAT; HI, HFD iWAT.
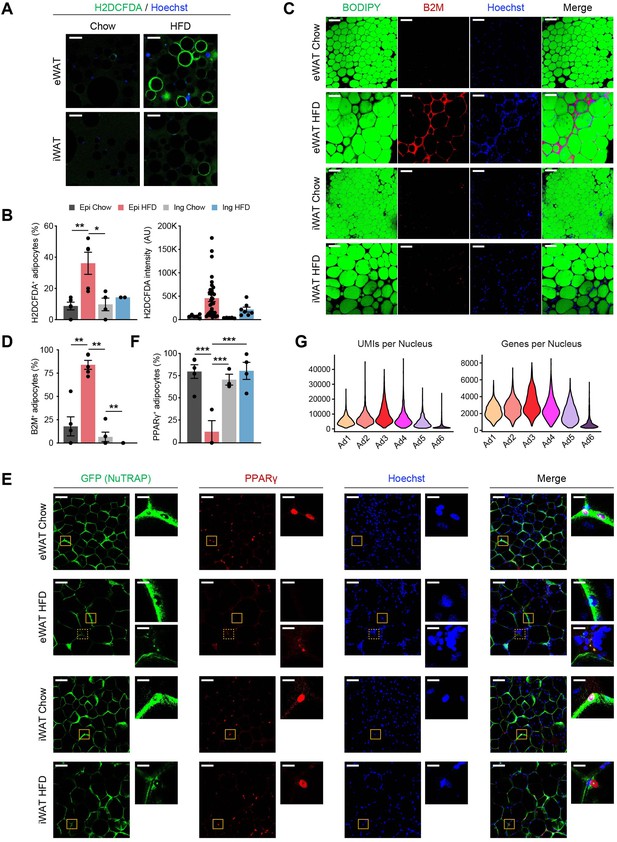
The Ad6 subpopulation represents the pathological state of adipocytes during obesity.
(A) Representative immunofluorescence microscopy images of adipocytes isolated from epididymal white adipose tissue (eWAT) and inguinal white adipose tissue (iWAT) of chow- and high fat diet (HFD)-fed mice stained with Hoechst and the chemical reactive oxygen species (ROS) reporter, H2DCFDA. Scale bars: 100 µm. (B) Quantification of H2DCFDA-positive adipocyte fraction (left) and intensity (right) per image in eWAT (n = 5) and iWAT (n = 2–4) from chow- and HFD-fed mice. (C) Representative images of whole-mount adipose tissue stained with BODIPY, B2M, and Hoechst by fat depot and diet. Scale bars: 50 µm. (D) Quantification of B2M-positive adipocyte fraction per image by fat depot and diet (n = 5, each). (E) Representative images of whole-mount eWAT and iWAT from chow- and HFD-fed NuTRAP mice stained with GFP, PPARγ, and Hoechst. The insets display select areas of GFP-labeled adipocyte nuclei. Note: The inset with the dotted line on eWAT HFD represents autofluorescence, not PPARγ. Scale bars: 100 and 20 µm (insets). (F) Quantification of PPARγ-positive adipocyte nucleus fraction among GFP-labeled adipocyte nuclei in eWAT (n = 3–5) and iWAT (n = 3–4) from chow- and HFD-fed mice. (G) Numbers of unique molecular identifiers (UMIs; left) and genes (right) detected per nucleus in each adipocyte subpopulation. Statistical analysis was performed using one-way ANOVA with Tukey post hoc test. *p < 0.05, **p < 0.01, ***p < 0.001.
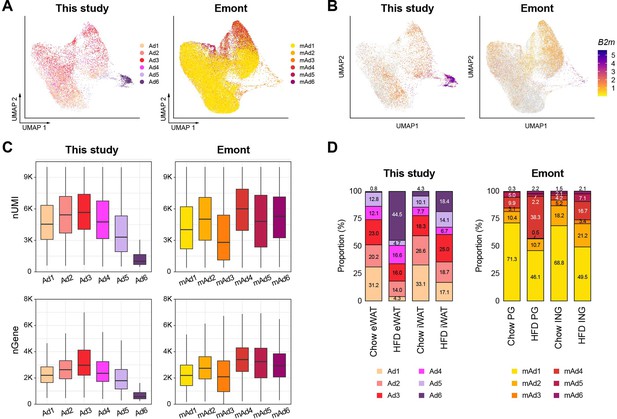
Comparison of adipocyte subpopulations identified in this study versus Emont et al., 2022.
(A) Uniform manifold approximation and projection (UMAP) visualization of integrated adipocyte nuclei from this study and Emont et al., 2022. (B) B2M gene expression in adipocyte nuclei from each dataset. (C) Unique molecular identifier (UMI) counts and genes detected per nucleus by adipocyte subpopulations in this study and .Emont et al., 2022. (D) Bar graphs displaying the relative proportions of adipocyte subpopulations identified in this study and Emont et al., 2022.
Additional files
-
Supplementary file 1
Distinct biological pathways affected in adipocyte subpopulations by high fat diet (HFD).
K-means clustering of differentially expressed genes (n = 4236) in at least one adipocyte subpopulation in either epididymal white adipose tissue (eWAT) or inguinal white adipose tissue (iWAT), based on their expression patterns across samples, results in four clusters. Each tab includes pathways enriched in each gene cluster, as determined by Metascape. Specific pathways highlighted in Figure 5 are marked with borders.
- https://cdn.elifesciences.org/articles/97981/elife-97981-supp1-v1.xlsx
-
Supplementary file 2
Sequences of primers used for quantitative real-time PCR (qRT-PCR).
- https://cdn.elifesciences.org/articles/97981/elife-97981-supp2-v1.docx
-
Source code 1
An R script containing Seurat analysis for data integration, clustering, and annotation.
- https://cdn.elifesciences.org/articles/97981/elife-97981-code1-v1.zip
-
Source code 2
An R script containing adipocyte-specific analyses.
- https://cdn.elifesciences.org/articles/97981/elife-97981-code2-v1.zip
-
Source code 3
An R script containing adipocyte-specific pseudobulk differential expression analysis.
- https://cdn.elifesciences.org/articles/97981/elife-97981-code3-v1.zip
-
Source code 4
An R script containing adipocyte-specific pseudotime analysis using Monocle 3.
- https://cdn.elifesciences.org/articles/97981/elife-97981-code4-v1.zip
-
Source code 5
An R script containing analyses specific to cell types other than adipocytes.
- https://cdn.elifesciences.org/articles/97981/elife-97981-code5-v1.zip
-
Source code 6
An R script containing a comparative analysis to the previously published work by Emont et al., 2022.
- https://cdn.elifesciences.org/articles/97981/elife-97981-code6-v1.zip
-
MDAR checklist
- https://cdn.elifesciences.org/articles/97981/elife-97981-mdarchecklist1-v1.pdf