Malaria: A genetic intervention
Malaria cases may have decreased over the last 15 years, but the number of parasites resistant to treatment is rising, particularly in Southeast Asia. Indeed, some Plasmodium falciparum parasites (which cause the most severe form of the disease) no longer respond to the most widely used antimalarial drugs (Blasco et al., 2017; Menard and Dondorp, 2017). This includes artemisinin-based combination therapy (ACT), the first line of defense recommended by National Malaria Control Programs (Noedl et al., 2008; Dondorp et al., 2009; Cheeseman et al., 2012; Ariey et al., 2014; Takala-Harrison et al., 2015; Amato et al., 2018; Hamilton et al., 2019; Imwong et al., 2020; Stokes et al., 2021). The reduced susceptibility to artemisinin and its partner drugs has resulted in ACT therapy failing to treat over 50% of malaria cases in some regions of Cambodia, Thailand and Vietnam (van der Pluijm et al., 2020; van der Pluijm et al., 2019).
In order to make rapid, effective decisions on how best to tackle this rise in resistance, policymakers need to be aware of which strains are present in different regions. However, clinical studies of malaria are logistically difficult and expensive to implement. An alternative approach is to study molecular markers in the blood of malaria patients, which can also provide an earlier indication of where resistant parasites have emerged (World Health Organization, 2020). Now, in eLife, Olivo Miotto and colleagues – including Christopher Jacob (Wellcome Sanger Institute) as first author – report a new genetic surveillance platform called ‘GenRe-Mekong’ which monitors the spread of resistant parasites in the Greater Mekong Subregion (Jacob et al., 2021).
Jacob et al. collaborated with various National Malaria Control Programs and scientific partners to collect 9,623 blood samples from patients diagnosed with P. falciparum malaria in eight countries (Vietnam, Laos, Cambodia, Thailand, Myanmar, Bangladesh, India and Democratic Republic of Congo). A cutting-edge sequencing technology was then applied to extract and amplify specific genes from the parasitic genome. Jacob et al. analyzed this genetic data for variants which are known to reduce parasites’ susceptibility to the most widely used treatments, including artemisinin (Figure 1). Based on the proportion of variants present in each gene, each sample was then classified as having a ‘sensitive’, ‘resistant’ or ‘undetermined’ response to an antimalarial drug.
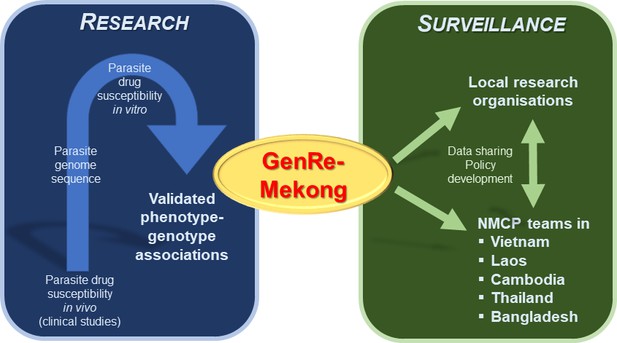
Bridging the gap between research and malaria surveillance in the Greater Mekong Subregion.
Previous research studying the genome of parasites has led to the identification of genetic variants which reduce parasites’ susceptibility to antimalarial drugs (blue box, left). Jacob et al. used this data to create a genetic surveillance platform called GenRe-Mekong, which analyzes the blood samples of malaria patients for these genetic variants (green box, right). Data from this platform is then regularly shared with local research organizations and various National Malaria Control Programs (NMCPs) in the Greater Mekong Subregion, who can use this information to help inform their policy decisions for tackling drug-resistant infections.
Data from the genetic surveillance platform were delivered to National Malaria Control Programs as maps which simply describe how the presence and absence of resistant parasites is changing over time. Notably, the team (who are based in the United States, United Kingdom, Vietnam, Laos, Cambodia, Thailand, Bangladesh, Switzerland, Democratic Republic of Congo, France, Myanmar and India) provided concrete examples of how this information can be translated into policy decisions. For example, the database found that parasites less susceptible to artemisinin and one of its partner drugs (originally identified in Cambodia and Thailand) had spread to southern provinces in Vietnam and Laos which were previously unaffected by these resistant strains. This led National Malaria Control Programs in these regions to reassess which frontline therapies to use and where to allocate resources to help combat the rise in drug resistance.
Beyond providing actionable information on the spread of resistance, the approach could also shed light on the complexity of infection, revealing whether a patient was carrying different species or strains of parasites at the same time. It could also be used to infer where the strains detected originated, which could help to reconstruct how parasites resistant to multiple drugs spread to different regions.
Although this platform could rapidly become an essential, complementary strategy for eliminating malaria, additional work is needed to overcome some drawbacks. First, the changes in drug susceptibility caused by the genetic variants is not directly tested, but assumed on the basis of earlier research (Figure 1). Second, the platform will be unable to capture new resistant strains, unless newly discovered variants are continuously added to the GenRe-Mekong database. Last, the current approach is unlikely to be applicable to countries in the African continent. This is because patients are often infected by multiple strains of P. falciparum at the same time, which makes it difficult to apply the method across many variant genes and classify the resistance profile. Nevertheless, the work by Jacob et al. demonstrates how genetic data can be a tremendously practical tool that can help policy makers rapidly adapt their treatment strategies in response to rising levels of drug resistance.
References
-
Origins of the current outbreak of multidrug-resistant malaria in Southeast Asia: a retrospective genetic studyThe Lancet Infectious Diseases 18:337–345.https://doi.org/10.1016/S1473-3099(18)30068-9
-
Artemisinin resistance in Plasmodium falciparum malariaNew England Journal of Medicine 361:455–467.https://doi.org/10.1128/microbiolspec.EI10-0013-2016
-
Evolution and expansion of multidrug-resistant malaria in Southeast Asia: a genomic epidemiology studyThe Lancet Infectious Diseases 19:943–951.https://doi.org/10.1016/S1473-3099(19)30392-5
-
Molecular epidemiology of resistance to antimalarial drugs in the Greater Mekong Subregion: an observational studyThe Lancet Infectious Diseases 20:1470–1480.https://doi.org/10.1016/S1473-3099(20)30228-0
-
Antimalarial drug resistance: a threat to malaria eliminationCold Spring Harbor Perspectives in Medicine 7:a025619.https://doi.org/10.1101/cshperspect.a025619
-
Evidence of artemisinin-resistant malaria in western CambodiaNew England Journal of Medicine 359:2619–2620.https://doi.org/10.1056/NEJMc0805011
-
Independent emergence of artemisinin resistance mutations among Plasmodium falciparum in Southeast AsiaThe Journal of Infectious Diseases 211:670–679.https://doi.org/10.1093/infdis/jiu491
-
ReportReport on Antimalarial Drug Efficacy, Resistance and Response: 10 Years of Surveillance (2010–2019) GenevaWorld Health Organization.
Article and author information
Author details
Publication history
Copyright
© 2021, Sutherland and Menard
This article is distributed under the terms of the Creative Commons Attribution License, which permits unrestricted use and redistribution provided that the original author and source are credited.
Metrics
-
- 806
- views
-
- 75
- downloads
-
- 1
- citations
Views, downloads and citations are aggregated across all versions of this paper published by eLife.
Download links
Downloads (link to download the article as PDF)
Open citations (links to open the citations from this article in various online reference manager services)
Cite this article (links to download the citations from this article in formats compatible with various reference manager tools)
Further reading
-
- Medicine
- Microbiology and Infectious Disease
- Epidemiology and Global Health
- Immunology and Inflammation
eLife has published articles on a wide range of infectious diseases, including COVID-19, influenza, tuberculosis, HIV/AIDS, malaria and typhoid fever.
-
- Epidemiology and Global Health
- Genetics and Genomics
Alzheimer’s disease (AD) is a complex degenerative disease of the central nervous system, and elucidating its pathogenesis remains challenging. In this study, we used the inverse-variance weighted (IVW) model as the major analysis method to perform hypothesis-free Mendelian randomization (MR) analysis on the data from MRC IEU OpenGWAS (18,097 exposure traits and 16 AD outcome traits), and conducted sensitivity analysis with six models, to assess the robustness of the IVW results, to identify various classes of risk or protective factors for AD, early-onset AD, and late-onset AD. We generated 400,274 data entries in total, among which the major analysis method of the IVW model consists of 73,129 records with 4840 exposure traits, which fall into 10 categories: Disease, Medical laboratory science, Imaging, Anthropometric, Treatment, Molecular trait, Gut microbiota, Past history, Family history, and Lifestyle trait. More importantly, a freely accessed online platform called MRAD (https://gwasmrad.com/mrad/) has been developed using the Shiny package with MR analysis results. Additionally, novel potential AD therapeutic targets (CD33, TBCA, VPS29, GNAI3, PSME1) are identified, among which CD33 was positively associated with the main outcome traits of AD, as well as with both EOAD and LOAD. TBCA and VPS29 were negatively associated with the main outcome traits of AD, as well as with both EOAD and LOAD. GNAI3 and PSME1 were negatively associated with the main outcome traits of AD, as well as with LOAD, but had no significant causal association with EOAD. The findings of our research advance our understanding of the etiology of AD.