Cell Culture: Implementing robotics and artificial intelligence
Most animals, including humans, are made up of different organs and tissues formed by specialized cells that perform specific roles. When tissues degenerate or become damaged, the affected cells have to be replaced so the tissues can keep performing their roles. This regenerative potential exists thanks to populations of stem cells in each tissue, which can divide to produce more stem cells – maintaining a constant pool of stem cells for repair – or differentiate into specialized cells to replace damaged cells.
The division and differentiation of stem cells needs to be in balance: if too many stem cells differentiate, the pool of stem cells could become exhausted, but if the stem cells divide uncontrollably this could lead to cancer. However, this balance often fails with age, or due to environmental or genetic reasons. One of the goals of regenerative medicine is to produce differentiated cells in the laboratory that can be used to heal tissues when they have lost the ability to regenerate for themselves (Pizevska et al., 2022). These treatments could have many potential long-term health benefits, including extending life expectancy, and research into these treatments is increasing both in the laboratory and in the clinic.
In order to use cells differentiated in the laboratory in a clinical setting, it is essential that the protocols used to produce the desired cell types reproducibly, and in high enough numbers. This is difficult to do because differentiating cells are highly sensitive to stimuli in their environments, meaning that their culture conditions have to be carefully controlled (Figure 1). Human operators introduce an added layer of variability that is difficult to control for, since each person does cell culture a little differently, and the protocols used to manufacture regenerative medicine products are often complex (Sebastian et al., 2019). Additionally, the requirements that cells and cell-derived products need to meet to be used in the clinic are changing rapidly, and the tests used to assess these requirements can also propagate variability (Simon et al., 2016). As a result of these issues, the quality of the cells produced for regenerative medicine treatments is often unreliable.
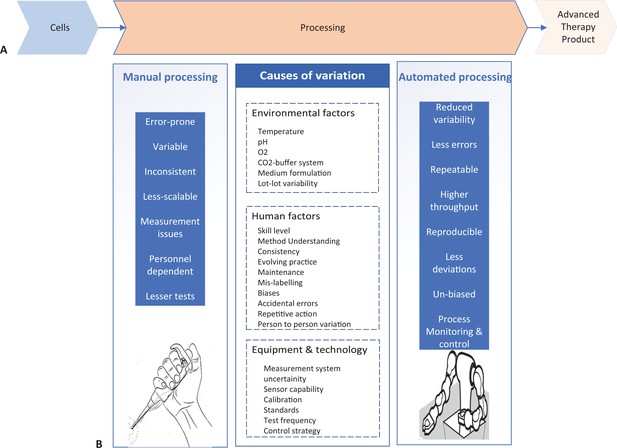
Comparison of manual and automated manufacturing of cell therapy products.
(A) Schematic of the development lifecycle of cell therapy products. Cells (left) undergo processing in the laboratory (center), where they are cultured under different conditions, often requiring several complex cell culture steps, to develop safe and efficacious therapy product (right). (B) The processing step of therapy product development can be done manually (left) or automatically (right). Manual processing has several factors (center left) that increase variation in the culture conditions. Causes of variation can be environmental factors, human factors or equipment and technology factors (center), and they lead to manufacturing being less consistent. Automated processing can correct the issues that manual processing introduces (center right).
In recent years, advanced imaging techniques and analysis have improved the reproducibility of the cell culture protocols used for the manufacture of cells in regenerative medicine (Imai et al., 2018). This suggests that further minimizing human error and variability could also greatly improve the manufacturing process. One way to do this would be to mechanize and automate the cell culture procedures. Now, in eLife, Yosuke Ozawa, Koichi Takahashi, Tohru Natsume, and colleagues (who are based at various institutions in Japan) – with Genki Kanda and Taku Tsuzuki as first authors – report on LabDroid Maholo, a robotics and artificial intelligence-based cell processing station that can reliably carry out complex manual cell culture procedures typically performed by trained scientists (Kanda et al., 2022).
Replacing a difficult and repetitive manual process with a machine is a critical step in reducing the variability that arises in cell culture protocols. Indeed, it has previously been shown that automating methods using robotics is an efficient way to reduce human error and operator-dependent variability in the laboratory (Archibald et al., 2016). Kanda et al. now demonstrate this principle in the context of cell culture by combining robotics and artificial intelligence to automate complex cell culture protocols.
The LabDroid Maholo System contains highly accurate and programmable robotic arms to manipulate the cell culture conditions, which allowed the robot to culture stem cells under different conditions. In this case, the LabDroid Maholo System was programmed to differentiate stem cells into retinal pigment epithelial cells. The resulting cells were imaged using the LabDroid Maholo’s integrated imaging system, and computer algorithms and statistical analyses were applied to analyze and quantify the level of cell differentiation against the given conditions using the captured images.
The data obtained from these images was then fed into a mathematical experimental design algorithm that uses a batch Bayesian optimization. This allowed the LabDroid Maholo System to determine which factors in the cell culture procedure needed to be optimized to produce more retinal pigment epithelial cells. Using this method allowed Kanda et al. to reduce millions of potential combinations of cell culture parameters to a more manageable number. Briefly, the batch Bayesian optimization used by Kanda et al. first compares the cell culture conditions against the obtained rate of differentiation, and then decides, with the help of artificial intelligence, whether to include further optimization steps. In this case, three successive optimization steps successfully identified the best cell culture conditions to repeatably differentiate stem cells into retinal pigment cells of adequate quality to be used in cell therapy research.
Kanda et al. provide one of the best examples of how to achieve process control and advanced optimization using a combined robotics and artificial intelligence system. The ability to characterize the cultured cells and establish an optimal cell culture protocol based on the data removes human intervention for these steps, reducing variability. Although the platform will need further improvements to provide end-to-end functionality, it is a step forward towards the reproducible manufacturing of cells and cell-derived products for regenerative treatments.
References
-
Comparability of automated human induced pluripotent stem cell culture: a pilot studyBioprocess and Biosystems Engineering 39:1847–1858.https://doi.org/10.1007/s00449-016-1659-9
-
Strategies for achieving measurement assurance for cell therapy productsStem Cells Translational Medicine 5:705–708.https://doi.org/10.5966/sctm.2015-0269
Article and author information
Author details
Publication history
Copyright
© 2022, Sebastian
This article is distributed under the terms of the Creative Commons Attribution License, which permits unrestricted use and redistribution provided that the original author and source are credited.
Metrics
-
- 4,256
- views
-
- 388
- downloads
-
- 3
- citations
Views, downloads and citations are aggregated across all versions of this paper published by eLife.
Download links
Downloads (link to download the article as PDF)
Open citations (links to open the citations from this article in various online reference manager services)
Cite this article (links to download the citations from this article in formats compatible with various reference manager tools)
Further reading
-
- Computational and Systems Biology
In eukaryotes, protein kinase signaling is regulated by a diverse array of post-translational modifications, including phosphorylation of Ser/Thr residues and oxidation of cysteine (Cys) residues. While regulation by activation segment phosphorylation of Ser/Thr residues is well understood, relatively little is known about how oxidation of cysteine residues modulate catalysis. In this study, we investigate redox regulation of the AMPK-related brain-selective kinases (BRSK) 1 and 2, and detail how broad catalytic activity is directly regulated through reversible oxidation and reduction of evolutionarily conserved Cys residues within the catalytic domain. We show that redox-dependent control of BRSKs is a dynamic and multilayered process involving oxidative modifications of several Cys residues, including the formation of intramolecular disulfide bonds involving a pair of Cys residues near the catalytic HRD motif and a highly conserved T-loop Cys with a BRSK-specific Cys within an unusual CPE motif at the end of the activation segment. Consistently, mutation of the CPE-Cys increases catalytic activity in vitro and drives phosphorylation of the BRSK substrate Tau in cells. Molecular modeling and molecular dynamics simulations indicate that oxidation of the CPE-Cys destabilizes a conserved salt bridge network critical for allosteric activation. The occurrence of spatially proximal Cys amino acids in diverse Ser/Thr protein kinase families suggests that disulfide-mediated control of catalytic activity may be a prevalent mechanism for regulation within the broader AMPK family.
-
- Computational and Systems Biology
- Genetics and Genomics
Obesity is a major risk factor for type 2 diabetes, dyslipidemia, cardiovascular disease, and hypertension. Intriguingly, there is a subset of metabolically healthy obese (MHO) individuals who are seemingly able to maintain a healthy metabolic profile free of metabolic syndrome. The molecular underpinnings of MHO, however, are not well understood. Here, we report that CTRP10/C1QL2-deficient mice represent a unique female model of MHO. CTRP10 modulates weight gain in a striking and sexually dimorphic manner. Female, but not male, mice lacking CTRP10 develop obesity with age on a low-fat diet while maintaining an otherwise healthy metabolic profile. When fed an obesogenic diet, female Ctrp10 knockout (KO) mice show rapid weight gain. Despite pronounced obesity, Ctrp10 KO female mice do not develop steatosis, dyslipidemia, glucose intolerance, insulin resistance, oxidative stress, or low-grade inflammation. Obesity is largely uncoupled from metabolic dysregulation in female KO mice. Multi-tissue transcriptomic analyses highlighted gene expression changes and pathways associated with insulin-sensitive obesity. Transcriptional correlation of the differentially expressed gene (DEG) orthologs in humans also shows sex differences in gene connectivity within and across metabolic tissues, underscoring the conserved sex-dependent function of CTRP10. Collectively, our findings suggest that CTRP10 negatively regulates body weight in females, and that loss of CTRP10 results in benign obesity with largely preserved insulin sensitivity and metabolic health. This female MHO mouse model is valuable for understanding sex-biased mechanisms that uncouple obesity from metabolic dysfunction.