Actin networks modulate heterogeneous NF-κB dynamics in response to TNFα
Figures
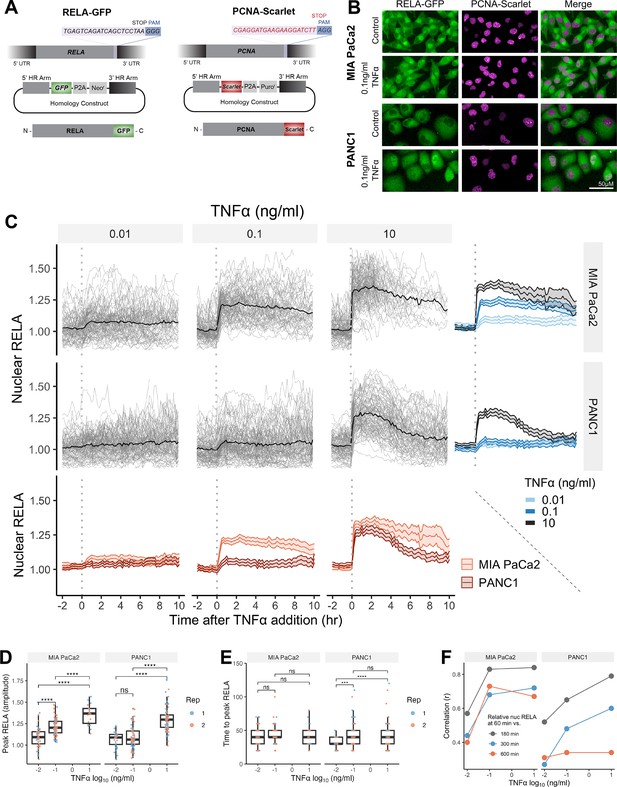
Single-cell analysis of live endogenous RELA-GFP dynamics with TNFα in human pancreatic cell lines.
(A) Schematic of CRISPR gRNA and homology constructs, with neomycin (neo) resistance for tagging of the RELA C-terminus with GFP and puromycin (puro) resistance for tagging of the PCNA C-terminus with Scarlet. (B) Confocal microscopy images of MIA PaCa2 and PANC1 monoclonal cell lines expressing endogenously tagged RELA-GFP and PCNA-Scarlet and treated with 1 hr solvent control or 0.1 ng/ml TNFα. (C) Tracks of single-cell nuclear RELA-GFP intensity measurements in MIA PaCa2 and PANC1 cells from –120 min to +600 min relative to TNFα addition (0.01, 0.1, and 10 ng/ml). n = 50–60 tracked cells per TNFα dose for each of the two experimental repeats. (D) Amplitude and (E) time of first nuclear RELA-GFP intensity peak in MIA PaCa2 cand PANC1 single cells. Boxplots show median and interquartile range. M = median per cluster. σ = standard deviation. Statistical significance shown for t-tests with Benjamini–Hochberg correction. ns (non-significant) = p>0.05, *p<0.05, **p<0.01, ***p<0.001, ****p<0.0001. (F) Pearson’s correlation coefficient (r) between nuclear RELA-GFP intensity at 60 min versus 180, 300, or 600 min within single-cell RELA-GFP tracks. Data are normalised within each track to nuclear RELA-GFP intensity at 0 min.
-
Figure 1—source data 1
Excel file with raw data and data replication information associated with Figure 1 and Figure 1—figure supplement 1.
Raw data from live microscopy single-cell analysis of MIA PaCa2 and PANC1 cells treated with various TNFα doses. Frequency of experimental and technical (well) repeats performed and frequency of single cells analysed per condition.
- https://cdn.elifesciences.org/articles/86042/elife-86042-fig1-data1-v2.xlsx
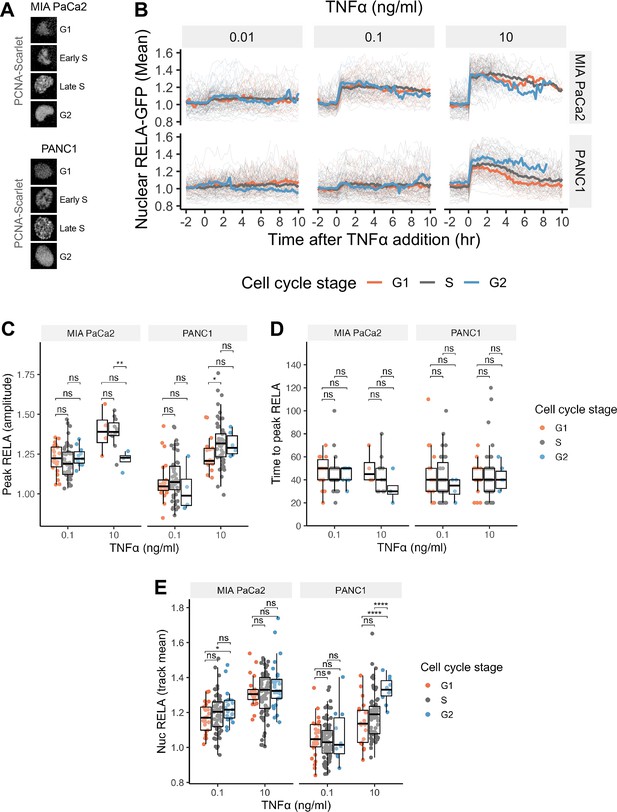
Cell cycle independence of TNFα-mediated RELA dynamics in PDAC cells.
(A) Confocal microscopy images of PCNA-Scarlet in representative MIA PaCa2 and PANC1 nuclei in G1, early S, late S or G2 cell cycle stage. (B) Single-cell nuclear RELA-GFP tracks for MIA PaCa2 and PANC1 cells categorised by cell cycle stage at the time of TNFα addition (0.01, 0.1, or 10 ng/ml), based on the intensity and appearance of PCNA-Scarlet. (C) Single-cell measurements of nuclear RELA intensity at track peak and (D) time to track peak by cell cycle stage at TNFα addition. (E) Mean nuclear RELA intensity per single-cell track, by cell cycle stage at TNFα addition. (C–E) show statistical significance from t-test comparisons with Benjamini–Hochberg correction: ns (non-significant) = p>0.05, *p<0.05, **p<0.01, ***p<0.001, ****p<0.0001.
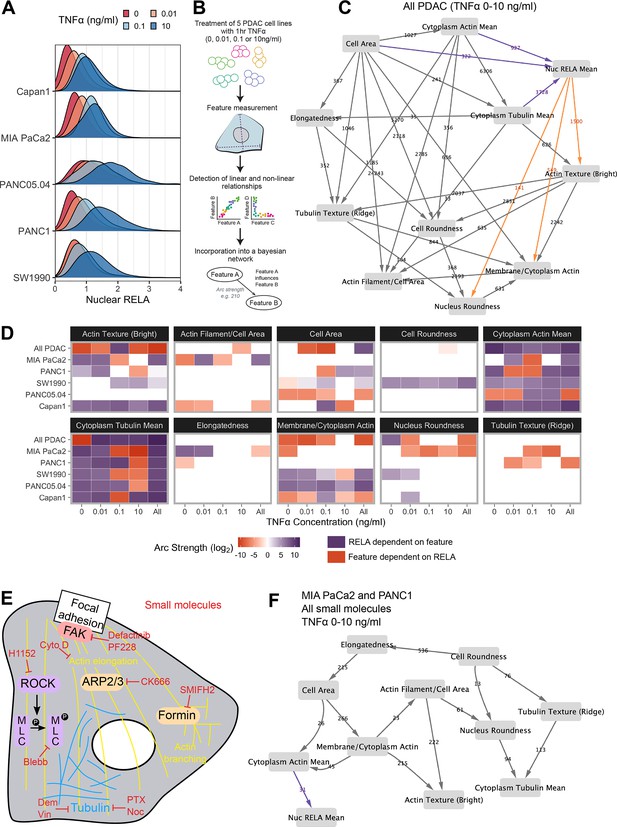
Bayesian network analysis predicts statistical dependence of RELA on actin.
(A) Single-cell nuclear RELA intensity distributions by immunofluorescence and automated image analysis. (B) Schematic depicting generation of a Bayesian network model for probabilistic relationships between cell features in PDAC cells. PDAC cells were treated with 0, 0.01, 0.1, or 10 ng/ml TNFα for 1 hr. Using automated image analysis of cell markers, features are measured on a single-cell basis and Bayesian analysis is used to detect linear and non-linear relationships between the features. These relationships are incorporated into a Bayesian network model, which is an influence diagram consisting of nodes, each representing a measured feature, and arcs between the nodes that depict predicted dependencies between the nodes. Network was generated using single-cell data with 1000 single cells sampled per cell line (MIA PaCa2, PANC1, Capan1, SW1990, and PANC05.04), TNFα dose and biological repeat (n = 3 biological repeats, each with four wells/technical replicates). (C) Bayesian network model incorporating data from all PDAC lines treated for 1 hr with 0 (solvent control), 0.01, 0.1, or 10 ng/ml TNFα. Values next to arcs represent the strength of the probabilistic relationship expressed by the arc (arc strength). Orange arcs connect features predicted to depend on nuclear RELA mean, and purple arcs connect features predicted to influence nuclear RELA mean. (D) Dependencies involving nuclear RELA mean in Bayesian network models generated with single-cell data for individual treatments or cell lines, or for all cell lines collated (top row in each cell feature section), or all treatments collated (rightmost column in each cell feature section). Purple indicates that nuclear RELA mean is predicted to depend on the cell feature in the Bayesian network model. Orange represents that a cell feature is predicted to depend on nuclear RELA intensity. Dependency strengths are calculated as log2(|arc strength|), multiplied by –1 for dependencies of cell features on nuclear RELA intensity. (E) Schematic indicating small molecules targeting the cytoskeleton. CK666 inhibits the ARP2/3 complex that mediates actin filament nucleation and branching (Mullins et al., 1998). SMIFH2 inhibits formins (Rizvi et al., 2009), which produce long straight filaments by promoting actin nucleation and filament elongation (Pruyne et al., 2002). Cytochalasin D binds to the growing end of actin filaments and inhibits polymerisation (Schliwa, 1982). H1152 targets Rho-kinase (ROCK), preventing ROCK phosphorylation of myosin light chain that normally promotes actin-binding and contractility, while blebbistatin blocks myosin II ATPase and actin contractility (Sasaki et al., 2002; Kovács et al., 2004). Tubulin-targeting drugs prevent MT assembly (vinblastine and nocodazole), limit MT formation and cause MT depolymerisation (demecolcine), or stabilise MTs and prevent disassembly (paclitaxel) (Spencer and Faulds, 1994; Vasquez et al., 1997; Gigant et al., 2005). Focal adhesion kinase (FAK) regulates turnover of focal adhesions, which are integrin-containing complexes linking intracellular actin to extracellular substrates. (F) Bayesian network model generated by single-cell data from MIA PaCa2 and PANC1 cells treated separately with the small molecules in (E) for 2 hr, then simultaneously treated with TNFα (0, 0.01, 0.1, or 10 ng/ml) for 1 hr. Numbers indicate arc strengths. In the presence of small molecule inhibition of the cytoskeleton, nuclear RELA mean is predicted to be dependent on cytoplasm actin mean alone, indicated by the purple arc connecting ‘cytoplasm actin mean’ and ‘nuc RELA mean’. Cells were analysed from three biological repeats, each with four wells/technical replicates. Numbers of cells per treatment and cell line are included in Figure 2—source data 1 (range 9,800–20,530 cells per treatment/cell line).
-
Figure 2—source data 1
Excel file with raw data and Bayesian arc strengths for Figure 2 and Figure 2—figure supplements 1–3.
Raw data from live microscopy image analysis (cell feature measurements) and downstream Bayesian arc strengths calculated using the Bnlearn package in R.
- https://cdn.elifesciences.org/articles/86042/elife-86042-fig2-data1-v2.xlsx
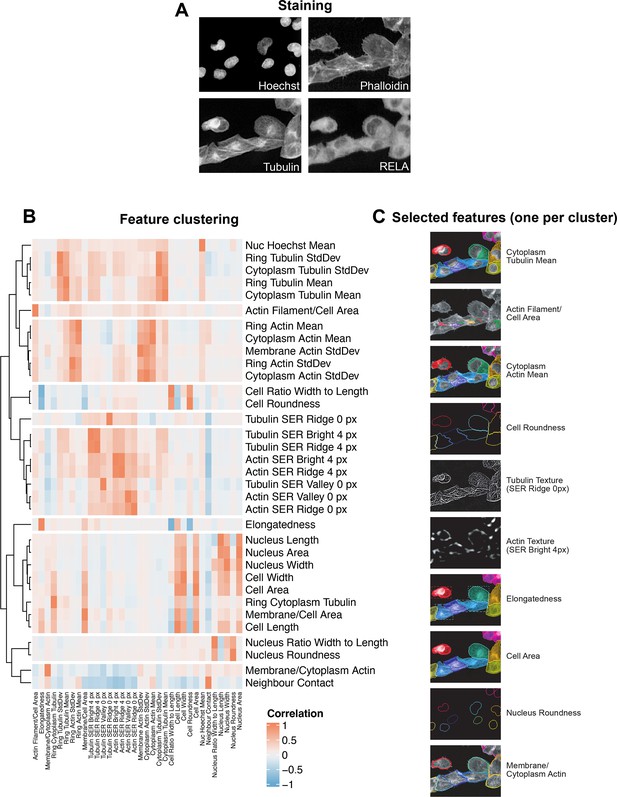
Automated image analysis of RELA localisation, cell shape, and the cytoskeleton in human cells.
(A) Staining by immunofluorescence (α-tubulin and RELA) and dyes marking DNA (Hoechst) and F-actin (phalloidin). Cell regions were segmented using Hoechst and α-tubulin stains and features were measured in these regions using the four stains. (B) Hierarchical clustering of 35 normalised cell features (excluding RELA measurements) measured in five PDAC cell lines. (C) Images and names of the selected features (one per cluster) in the reduced feature set.
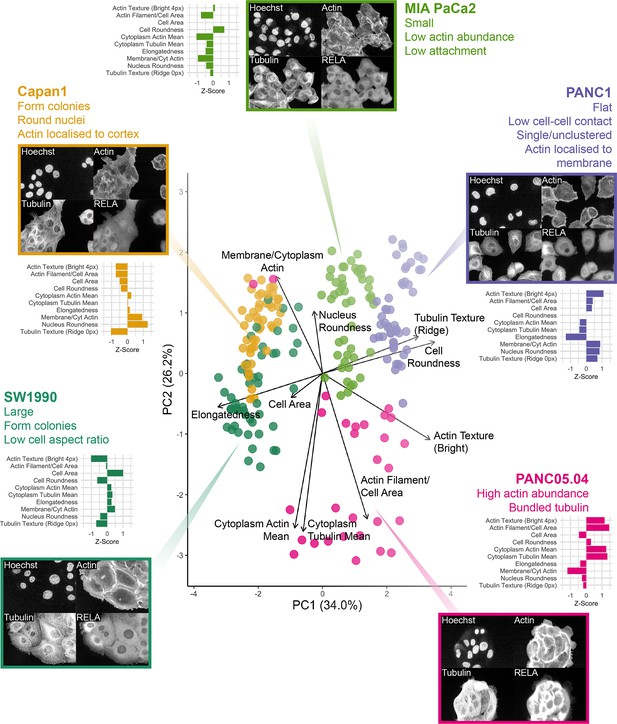
Principal component analysis (PCA) of five human PDAC cell lines with a reduced set of cytoskeletal and cell shape features.
PCA of the 10 reduced features used in Bayesian analysis for five PDAC cell lines using two principal components (PC1 and PC2). Overlayed is a biplot (arrows) as an indication of the contribution of each cell feature to the two PCs. Circles represent technical replicates. n = 4 biological repeats. Horizontal bar graphs for individual cell lines show mean z-scores for each feature, calculated across all cell lines to compare differences in each feature between the cell lines. Z-scores were calculated using the mean and standard deviation for control measurements across all cell lines for each biological repeat. Displayed is the mean z-score across all biological repeats (n = 4). Images for each cell line show Hoechst, phalloidin marking F-actin (Actin), α-tubulin (Tubulin), and RELA staining by immunofluorescence.
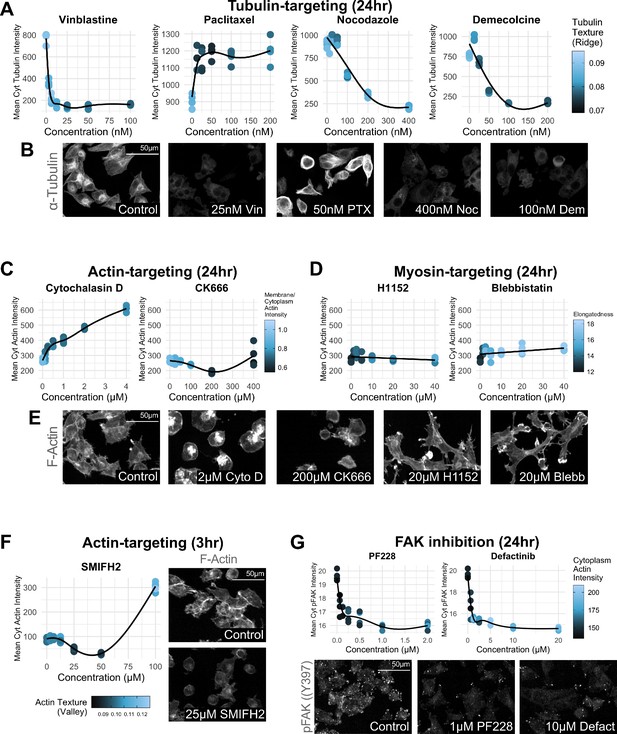
Dose–responses for cytoskeleton-targeting drugs.
Dose–response curves and representative images of MIA PaCa2 cells treated with small molecules in the absence of TNFα. n = 4 technical replicates per drug dose. (A, B) 24 hr treatment with tubulin-targeting drugs: vinblastine (0–100 nM), paclitaxel (0–200 nM), nocodazole (0–400 nM), and demelcoline (0–200 nM). (C, E) 24 hr treatment with actin-targeting drugs: cytochalasin D (0–4 µM) and CK666 (0–400 µM). (D, E) 24 hr treatment with myosin-targeting drugs: H1152 (0–40 µM) and blebbistatin (0–40 µM). (F) 3 hr treatment with the actin-targeting drug SMIFH2 (0–100 µM). (G) 24 hr treatment with the FAK inhibitors PF-573228 (PF228; 0–2 µM) and defactinib (0–20 µM).
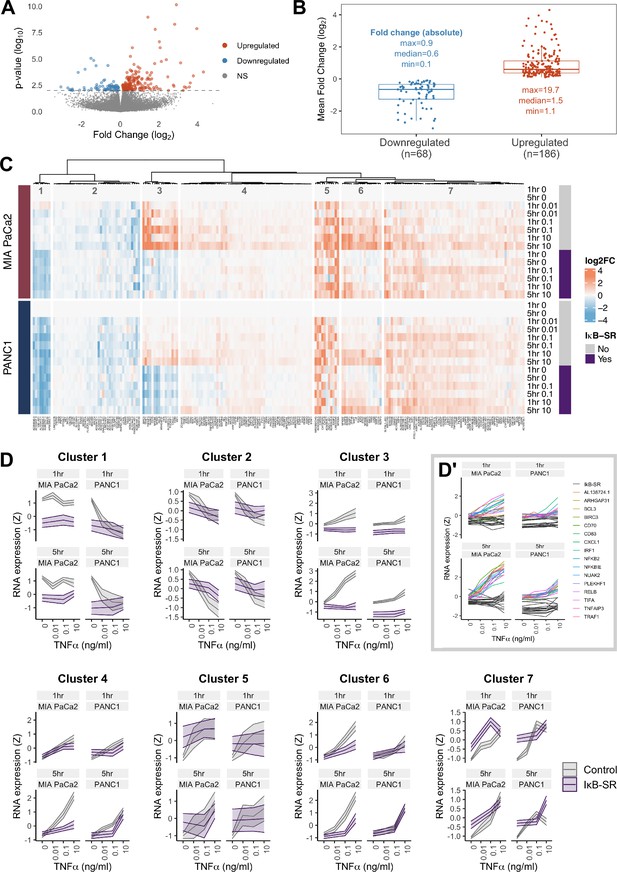
RNA-seq analysis of genes regulated by TNFα and RELA in human PDAC cells.
(A) Volcano plot of p-value against mean fold-change (log2) per gene comparing RNA expression with high TNFα (0.1 or 10 ng/ml) to control conditions (no TNFα) across MIA PaCa2 and PANC1 cells, using abundance at both 1 and 5 hr (handled as pseudo-replicates). Counts were normalised and log2 fold-changes were calculated using DESeq2. Two technical replicates were processed per cell line and treatment. (B) Mean fold-change (log2) across MIA PaCa2 and PANC1 cells for genes significantly downregulated (n = 68) or upregulated (n = 186) by TNFα (p<0.01) from (A). Also displayed are the maximum, median and minimum absolute fold-changes for downregulated and upregulated genes. (C) Clustered heatmap of TNFα regulated genes. Normalised counts from DESeq2 were log2 transformed and relative to the respective control (no TNFα or IκB-SR) per timepoint (1 or 5 hr) and cell line (MIA PaCa2 or PANC1), then z-scored across all samples independent per gene. Columns are annotated by gene and rows are annotated by cell line, TNFα dose and time, and presence of IκB-SR. (D) Z-scores for all genes per cluster, faceted by cell line and treatment time (1 or 5 hr). Ribbons show the 95% confidence interval and the middle line depicts the mean. Colour corresponds to the presence of IκB-SR. (D’) Individual genes within cluster 3, coloured by gene for control (no IκB-SR) data and grey for all data with IκB-SR (all genes).
-
Figure 3—source data 1
Excel file with bulk RNA-seq data and protein abundance from mass-spectrometry following co-immunoprecipitation of RELA-GFP.
Bulk RNA-seq expression data, results from DESeq2 analysis (R), and fold-changes calculated in R for Figure 3 and Figure 3—figure supplement 1. FASTQ files and processed counts are available from GEO under accession GSE268743. Protein abundance of RELA interactors from mass spectrometry analysis of MIA PaCa2 cells expressing RELA-GFP with GFP-Trap co-immunoprecipitation, shown in Figure 3—figure supplement 1B.
- https://cdn.elifesciences.org/articles/86042/elife-86042-fig3-data1-v2.xlsx
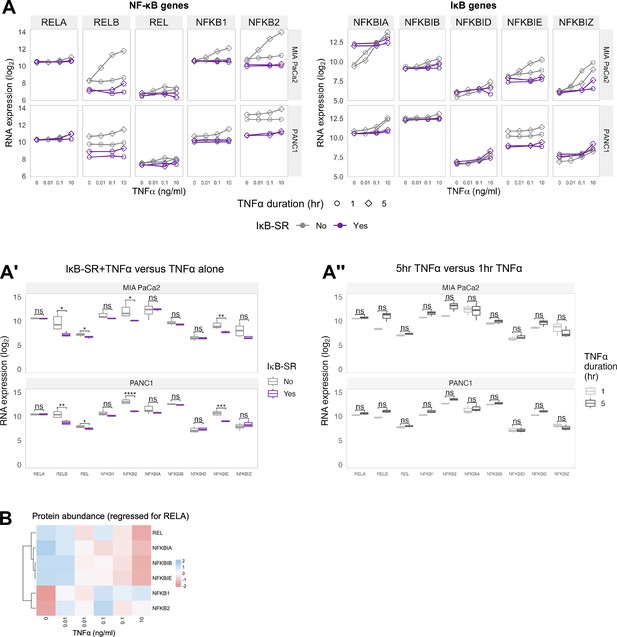
Hierarchical clustering of TNFα/RELA-regulated gene expression in PDAC cells.
(A) RNA-seq expression of genes encoding NF-κB (left) and IκB (proteins) in MIA PaCa2 and PANC1 cells treated with TNFα for 1 or 5 hr in the presence of IκB-SR (constitutively active IκB) or a DMSO control. Normalised counts were generated by DESeq2 and log2 transformed. (A’’, A’’’) Statistical analysis (Wilcoxon tests with Benjamini–Hochberg correction) of data in (A) comparing RNA expression of each gene (A’’) between cells treated with TNFα (data from 0.01, 0.1, and 10 ng/ml, and 1 and 5 hr treatment collated) in the presence or absence of IkB-SR; or (A’’’) between cells with 1 or 5 hr TNFα treatment (0.01, 0.1, and 10 ng/ml collated) in the absence of IkB-SR. ns (non-significant) = p>0.05, *p<0.05, **p<0.01. (B) Summary of proteins detected by Co-IP and mass spectrometry using GFP-Trap in MIA PaCa2 RELA-GFP cells. Cells were treated with TNFα (0, 0.01, 0.1, or 10 ng/ml) for 1 hr. Pull-downs were analysed using mass spectrometry. n = 1 experimental repeat for 0 and 10 ng/ml TNFα and n = 2 experiment repeats for 0.01 and 0.1 ng/ml TNFα. Protein abundances at each TNFα dose adjusted to RELA abundance.
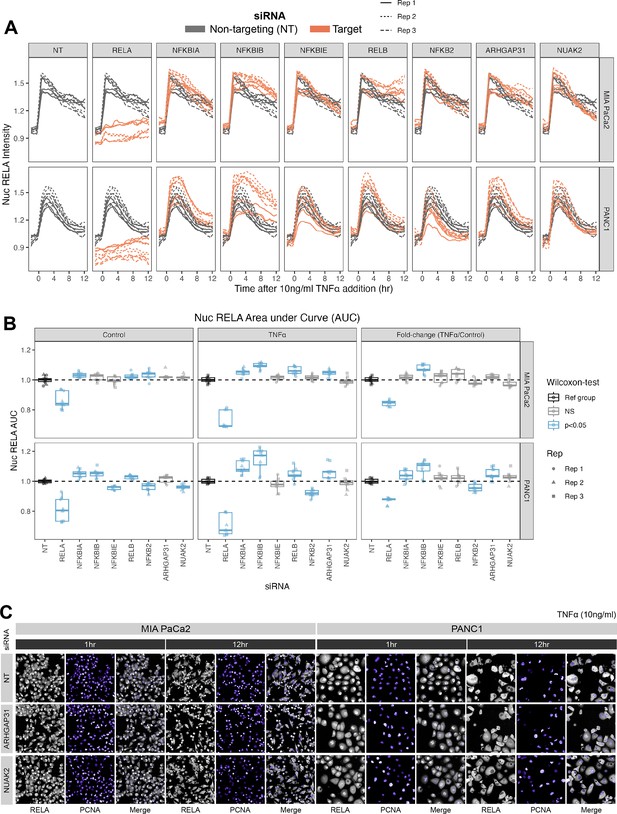
Effects of siRNA knockdown of genes involved in TNFα and actin signalling on RELA dynamics.
(A) Tracks of RELA-GFP intensity measurements relative to the timing of 10 ng/ml TNFα addition (–2 hr to +12 hr) following 48 hr siRNA incubation, across two PDAC cell lines: MIA PaCa2 and PANC1. Each panel corresponds to an siRNA, with the three biological/experimental replicates shown in different line styles (solid, dotted, and dashed). Each line depicts well mean data (n = 3 wells/technical replicates per biological repeat). Grey lines represent RELA-GFP measurements with non-targeting (NT) siRNA and are identical in all facets. Orange lines show RELA-GFP tracks from cells with siRNA depletion for the target gene indicated in the column facet. (B) Area under the curve (AUC) measurements (see ‘Materials and methods’) for nuclear RELA intensity over time for each siRNA gene knockdown, for MIA PaCa2 and PANC2 cells treated with 10 ng/ml TNFα or control treatment. All data are normalised by dividing the nuclear RELA AUC by the mean AUC for the NT siRNA control by biological repeat, cell line and treatment (TNFα versus control). Statistical results are shown for Wilcoxon tests with Benjamini–Hochberg correction for multiple comparisons, testing for differences in AUC measurements between technical replicates for each siRNA to the non-targeting siRNA control, independently by cell line and treatment (TNFα versus control). Blue boxplots represent statistically significant (p<0.05) results and grey boxplots show statistically non-significant results. n = 3 experimental repeats, each with three wells/technical replicates per treatment (siRNA, TNFα dose, and cell line). Experimental repeats are depicted by shape (Rep 1 = circle, Rep 2 = triangle, Rep 3 = square). AUC fold-changes (rightmost facet) are calculated by dividing each measurement by the mean of the control (no TNFα) measurements for each siRNA. (C) Snapshots from confocal microscopy timelapse imaging of endogenous RELA-GFP (grey) and PCNA-Scarlet (nuclear marker, shown in purple) in MIA PaCa2 and PANC1 cells following NT, ARHGAP31, or NUAK2 48 hr siRNA treatment, followed by 1 or 12 hr 10 ng/ml TNFα addition.
-
Figure 4—source data 1
Excel file with nuclear RELA intensity measurements from live imaging analysis with siRNA gene knockdown.
Raw data from live microscopy image analysis of nuclear RELA and the area under the curve (AUC) from Figure 4 and Figure 4—figure supplement 2. Quantification by qPCR of RNA abundance for MIA PaCa2 cells expressing RELA-GFP and treated with various siRNAs for 48 hr prior to control/TNFα treatment.
- https://cdn.elifesciences.org/articles/86042/elife-86042-fig4-data1-v2.xlsx
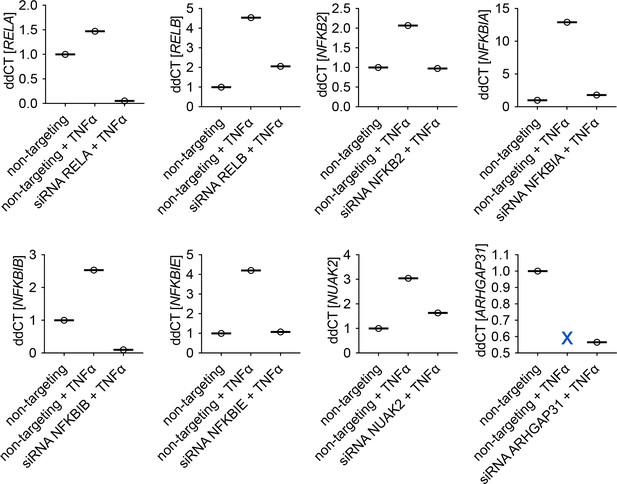
Validation of siRNA knockdown by qRT-PCR.
MIA PaCa2 cells expressing endogenous RELA-GFP were transfected with siRNA for 48 hr, then treated with 1 hr 10 ng/ml TNFα or vehicle control. Gene expression was determined by qRT-PCR (n = 3 technical replicates) and normalised to GAPDH expression.
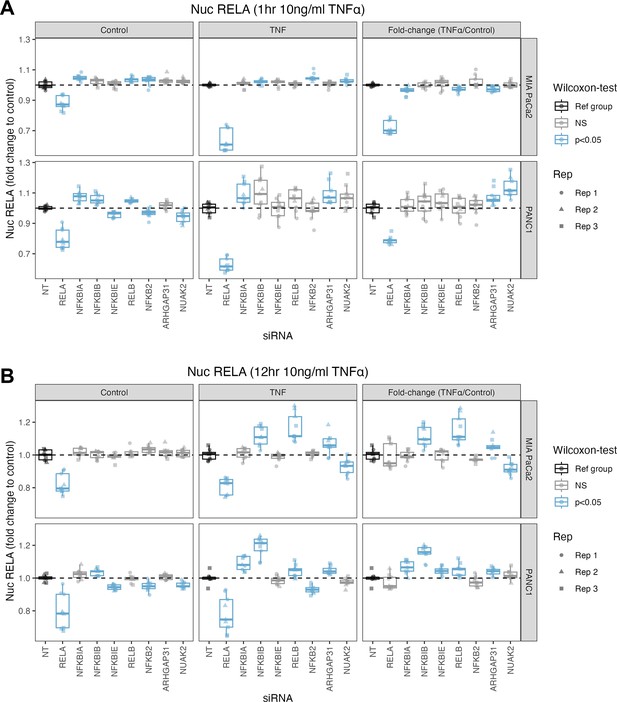
Mean nuclear RELA intensity in MIA PaCa2 and PANC1 cells following siRNA and TNFα treatment.
(A, B) Nuclear RELA-GFP intensity measurements for cells at (A) 1 hr or (B) 12 hr following 10 ng/ml TNFα addition. n = 3 biological repeats, each with three technical/well replicates per siRNA, TNFα dose, and cell line. All data are normalised to the mean nuclear RELA intensity of the non-targeting control by experimental repeat, cell line and treatment (TNFα or control). Statistical results are shown for multiple Wilcoxon tests with Benjamini–Hochberg correction comparing technical replicates from each siRNA to the non-targeting siRNA control, independently by cell line and treatment (0 or 10 ng/ml TNFα). Blue boxplots represent statistically significant (p<0.05) results and grey boxplots show statistically non-significant results. Experimental repeats are depicted by circles (Rep 1), triangles (Rep 2), or squares (Rep 3). The nuclear RELA fold-change (rightmost facets in each graph) is calculated by dividing each measurement by the mean of the control (no TNFα) measurements by each siRNA.