Whole blood transcriptional profiles and the pathogenesis of tuberculous meningitis
Figures

Objectives and cohorts flow.
TBM: TB meningitis, HIV: human immunodeficiency virus, PTB: pulmonary TB, HC; healthy controls.
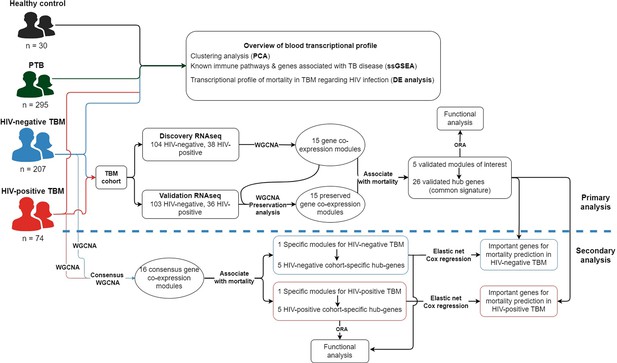
Analysis workflow diagram.
Transcriptional profiling was conducted across four cohorts: 207 HIV-negative TBM patients, 74 HIV-positive TBM patients, 295 pulmonary tuberculosis (PTB) patients, and 30 healthy controls. To identify transcriptional signatures associated with TBM mortality, RNA-seq data from all 281 TBM participants were analyzed. For reproducibility, the RNA-seq TBM cohort was randomly split into a discovery cohort (n=142) and a validation cohort (n=139). Weighted gene co-expression network analysis (WGCNA) was initially performed to identify common hub genes and pathways related to TBM mortality. Additionally, consensus WGCNA was applied to identify signals specific to either the HIV-negative or HIV-positive TBM cohorts. Outcome prediction models were then developed based on the common and specific hub genes associated with TBM. Finally, the association of hub genes with the outcome and prediction models was validated using qPCR on an independent cohort of HIV-negative TBM patients (n=132).
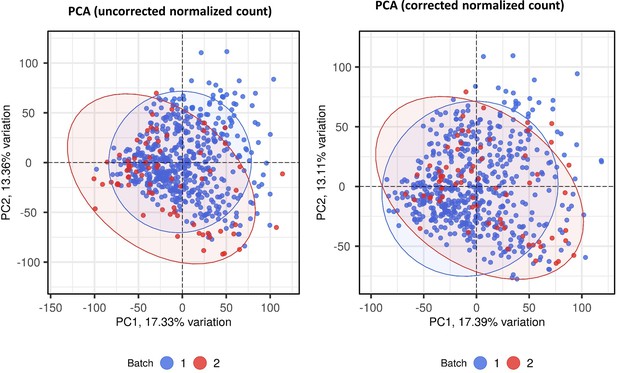
Batch correction for RNA-seq normalized count data.
Principle component analysis (PCA) of transcriptomic data before (A) and after (B) batch correction by combat function in SVA r package. Each symbol represents one individual with color coding different cohorts. The x-axis represents principle component (PC) 1, while y-axis represents PC2.
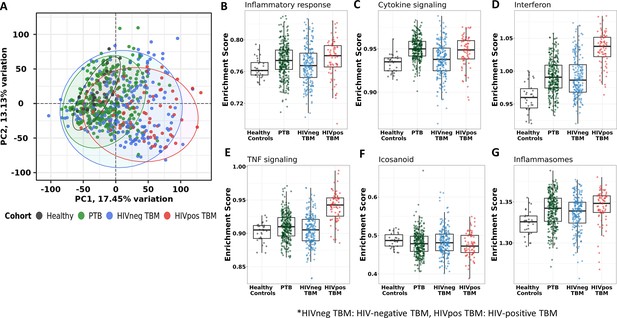
Blood transcriptomic profiles of four cohorts: healthy controls (n=30), PTB (n=295), HIV-negative TBM (n=207), and HIV-positive TBM (n=74).
(A) Principle component analysis (PCA) of whole transcriptomic profile of HC, PTB and TBM with and without HIV. Each symbol represents one individual with color coding different cohorts. The x-axis represents principle component (PC) 1, while y-axis represents PC2. (B–G) Enrichment scores of known innate immunity pathways associated with TBM pathogenesis. Pathway enrichment scores were calculated using single sample GSEA algorithm (ssGSEA) (Barbie et al., 2009). Each dot represents one participant. The box presents median, 25th to 75th percentile and the whiskers present the minimum to the maximum points in the data.
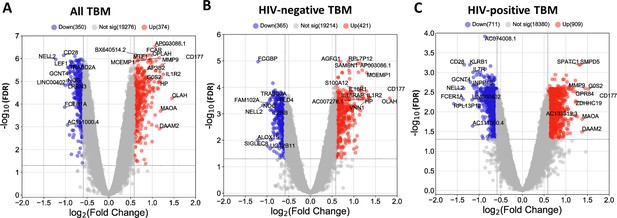
Blood transcriptomic profiles of three-month mortality at baseline in all TBM and TBM stratified by HIV status.
Volcano plot showed differentially expressed (DE) genes by fold change (FC) between death and survival in all TBM (A), HIV-negative (B) and HIV-positive TBM (C). Each dot represents one gene. The x-axis represents log2 FC. The y-axis showed –log10 FDR of genes. DE genes were colored with red indicating up-regulated, blue indicating down-regulated genes which having fold discovery rate (FDR) <0.05 and absolute FC >1.5.
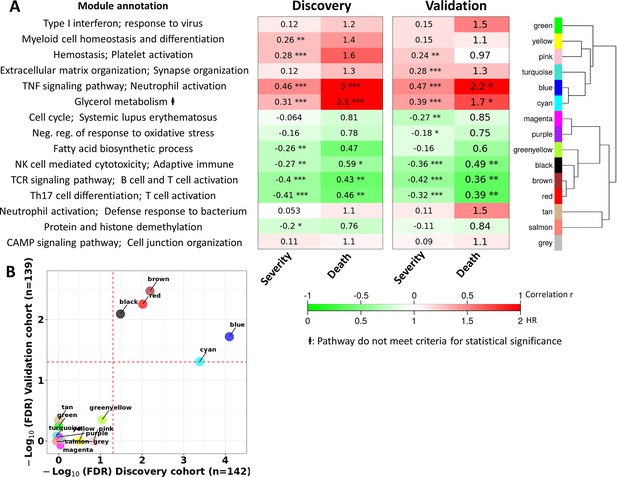
Blood transcriptional modules associated with mortality in TBM.
(A) Associations between WGCNA modules with two clinical phenotypes TBM disease severity (MRC grade) and three-month mortality in discovery and validation cohorts, and their associated biological processes. The heatmap showed the association between principle component 1 (PC1) of each module and the phenotypes, particularly Spearman correlation r for MRC grade and hazard ratio per increase 1/10 unit of PC1 (HR) for mortality. The HRs were estimated using a Cox regression model adjusted for age, HIV status and dexamethasone treatment. False discovery rate (FDR) corrected based on Benjamini & Yekutieli procedure, with significant level denoted as *<0.05, **<0.01 and ***<0.001. Gradient colors were used to fill the cell with green indicating negative r or HR <1, red color indicating positive r or HR >1. The order of modules was based on hierarchical clustering using Pearson correlation distance for module eigengene. On the left, biological processes, corresponding to modules, were identified using Gene Ontology and KEGG database. (B) Validation of the association between WGCNA modules and mortality in discovery and validation cohorts. X-axis represents –log10 FDR in discovery cohort and Y-axis represents –log10 FDR in validation cohort. Red dash lines indicate FDR = 0.05 as the threshold for statistically significant in both cohorts. Five modules (blue, brown, red, black and cyan) with FDR <0.05 were validated.
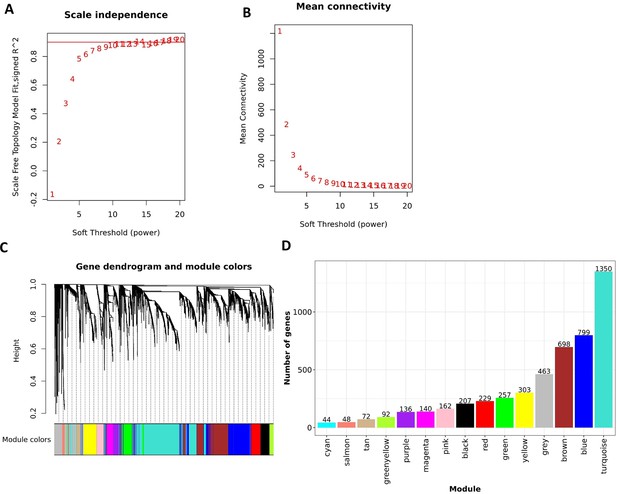
Construction of WGCNA in discovery cohort.
Analysis of network topology for various soft-thresholding powers of the top 5000 most variant genes based on the scale-free network model. (A) The scale-free fit index (y-axis) as a function of the soft-thresholding power (x-axis). The red horizontal line corresponds to and soft-thresholding power =8, which was chosen for the construction gene-expression network. (B) The mean connectivity on the y-axis as a function of the soft-thresholding power on the x-axis. The adjacency matrix of the scale-free network between genes was determined as where . (C) The dendrogram resulted from the hierarchical clustering analysis using topological overlap of the adjacency matrix A served as a dissimilarity metric. Each cluster was referred as a module and assigned with a color. (D) Bar-plots indicate the number of genes contained in each module.
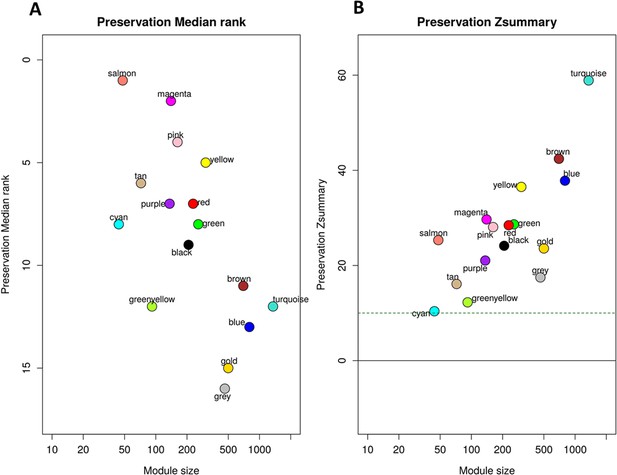
Preservation of discovery modules in validation cohort.
(A) The median rank preservation statistics of the modules. Each module was represented by a point, labeled with the corresponding color and name. The Y-axis represents the median rank of observed preservation statistics per module, while the X-axis indicates the number of genes within each module. A low preservation median rank value indicates a high level of preservation. The gold module was an artificial module comprised of 500 randomly selected genes. The grey module consisted of non-connected genes identified in the WGCNA analysis of the discovery cohort. These two modules exhibited the highest median rank, indicating low preservation, which ensured our preservation analysis controlling well the background noise signal. (B) The Zsummary preservation statistics of the modules. Each module was represented by a point, labeled with the corresponding color and name. The Y-axis represents the Zsummary statistic of each module based on 1,000 permutations of module labels, while the X-axis indicates the number of genes within each module. The horizontal green dash line corresponds to the threshold of Zsummary >10 indicating strongly persevered modules. The presence of all modules in discovery were validated in validation cohort with Zsummary >10.
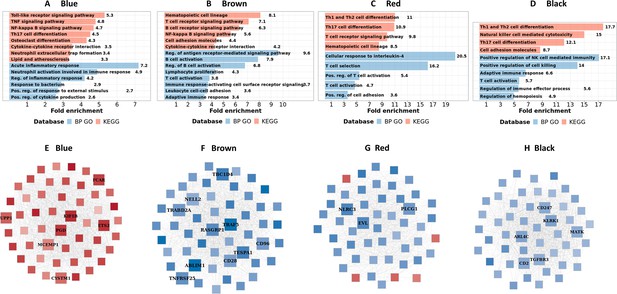
Biological processes, pathways and hub genes of validated modules associated with mortality.
(A–D) showed biological processes and pathways identified in four mortality associated modules: blue, brown, red and black module, by over representation analysis (ORA). Bar plots show the top representative GO biological processes or KEGG pathways. The bars indicates biological processes or pathways having ORA FDR <0.05 and size corresponding to fold enrichment calculated as the ratio of gene number of pathway in the input list divided by the ratio of gene number of the pathway in reference. (E–H) showed gene co-expression networks and hub genes of blue, brown, red and black module, respectively. Each node represents one gene. Each edge represents the link between two genes. Hub genes were shown by bigger nodes and bold text. The gradient color of node corresponds to its HR per 1 log2 unit increase in gene expression related to mortality, with red indicating HR >1, and blue HR <1.
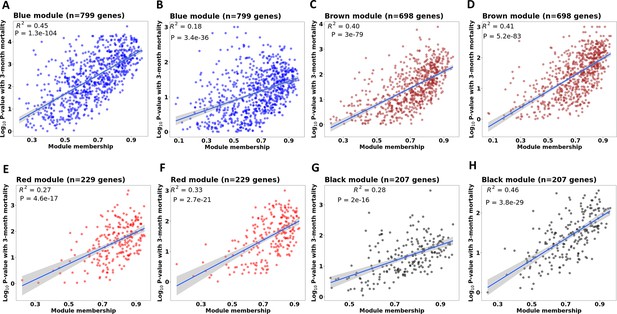
Correlations between gene module membership and gene significance with mortality in four associated modules in discovery and validation cohorts.
(A, C, E and G) are scatter plots for blue, brown, red and black modules in discovery cohort. (B, D, F and H) are scatter plots for blue, brown, red and black modules in validation cohort. For each module, each dot represents one gene in the module. The X-axis represents module membership calculated by Pearson correlation between gene expression level and its corresponding PC1 of that module. The Y-axis represents –log10(p-value) of the association between gene expression and 3-month mortality based on the Cox regression model adjusted for age, HIV, and dexamethasone treatment. Hub-genes were those on the right corner of the plot. The displayed R2 and p value were taken from linear regression models.
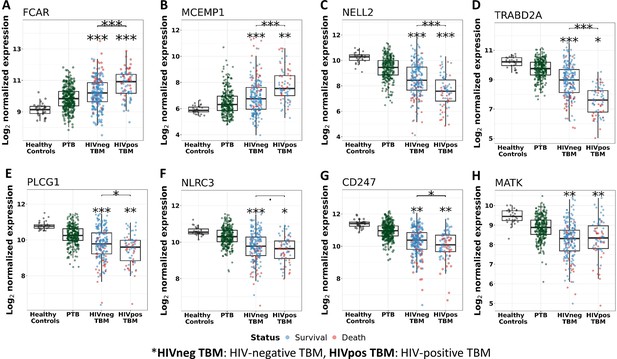
Gene expression of representative hub genes in healthy controls (n=30), PTB (n=295), HIV-negative TBM (n=207), and HIV-positive TBM (n=74).
Each dot represents gene expression from one participant. (A, B) expression of FCAR and MCEMP1 hub genes from the blue module. (C, D) expression of NELL2 and TRABD2A hub genes from the brown modules. (E, F) expression of PLCG1 and NLRC3 hub genes from the red module. (G, H) expression of CD247 and MATK hub genes from the black module. The box presents median, 25th to 75th percentile and the whiskers present the minimum to the maximum points in the data. Comparisons were made between death (red) with survival (blue) or between HIV-negative and HIV-positive TBM by Wilcoxon rank sum test with p-values displayed as significance level above the boxes and the horizontal bars (*<0.05, **<0.01, ***<0.001).
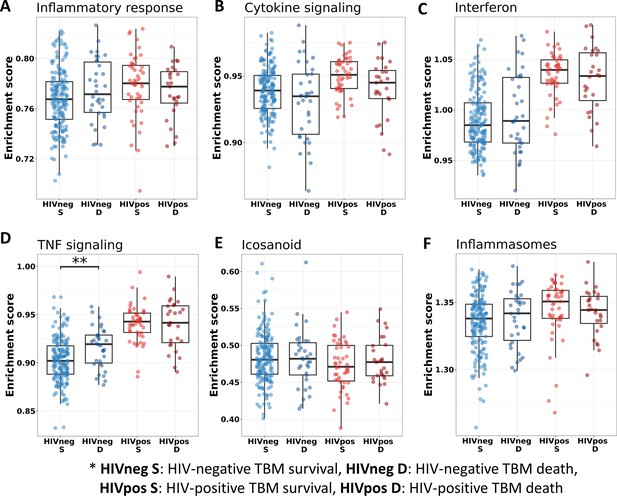
Relationship between known pathways associated with TBM pathogenesis and mortality.
(A–F) Enrichment scores of known immune pathways associated with TBM pathogenesis. Pathway enrichment scores were calculated using single sample GSEA algorithm (Barbie et al., 2009). Each dot represents one participant. The box presents median, 25th to 75th percentile and the whiskers present the minimum to the maximum points in the data. The comparisons were made between survival and death using Wilcoxon rank sum test. Only significant results are presented with *<0.05, **<0.01, ***<0.001.
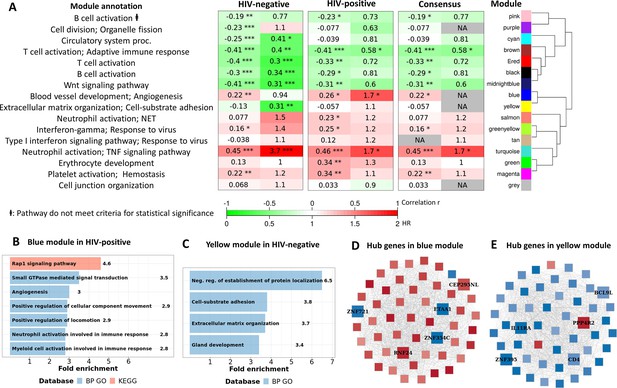
Consensus transcriptional modules associated with TBM mortality stratified by HIV-infection.
(A) Associations between 16 consensus WGCNA modules with two clinical phenotypes TBM severity (MRC grade) and mortality in HIV-negative (n=207) and HIV-positive (n=74) TBM participants, and their associated BP Gene ontology or KEEG database. The heatmap showed the association between modules and the phenotypes, with Spearman correlation r for MRC grade and hazard ratio per increase 1/10 unit of PC1 of module (HR) for mortality in HIV-positive and HIV-negative cohorts. The consensus sub-panel presented associations of the consensus modules and clinical phenotypes with same trend detected in both HIV cohorts, otherwise were annotated with missing (NA) values. False discovery rate (FDR) corrected using Benjamini & Yekutieli procedure, with significant level denoted as *<0.05, **<0.01 and ***<0.001. Gradient colors were used to fill the cell with green indicating negative r or HR <1, red color indicating positive r or HR >1. The order of modules was based on hierarchical clustering using Pearson correlation distance for module eigengene. It is noted that these consensus modules were not identical to the identified modules in the primary analysis in Figure 4A. (B–C) Functional enrichment analysis of HIV-positive pathway (blue module) and HIV-negative pathway (yellow module), respectively. (D–E) Gene co-expression network of blue and yellow modules. Each node represents one gene. Each edge represents the link between two genes. Hub genes were shown by bigger nodes with bold text. The gradient color of node corresponds to its HR per 1 log2 unit increase in gene expression related to mortality, with red indicating HR >1, and blue HR <1.
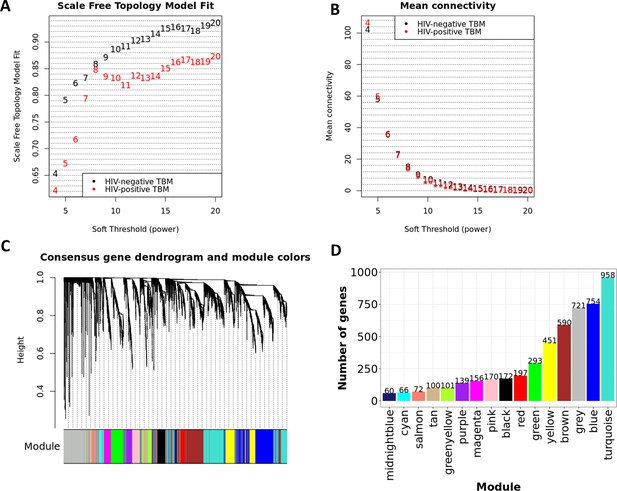
Construction of consensus WGCNA in HIV-negative (n=207) and HIV-positive (n=74).
Consensus analysis of network topology for various soft-thresholding powers of the top 5000 most variant genes based on the scale-free network model. (A, B) The summary network indices (y-axes) as functions of the soft thresholding power (x-axes). Numbers in the plots indicate the corresponding soft thresholding powers. The plots indicated that approximate scale-free topology is attained around the soft-thresholding power of 8 for both sets, which is the lowest power that satisfies the approximate scale-free topology criterion for both cohorts, HIV-positive (black dots) and HIV-negative (red dots). The adjacency matrix of the scale-free network between genes of each cohort was determined and scaled. The consensus topological overlap of two adjacency matrix were identified and served as dissimilarity metric for the hierarchical clustering. (C) The dendrogram of the consensus gene co-expression network. Each cluster was referred as a module and assigned with a color. (D) The bar-plot indicates the number of genes contained in each module.
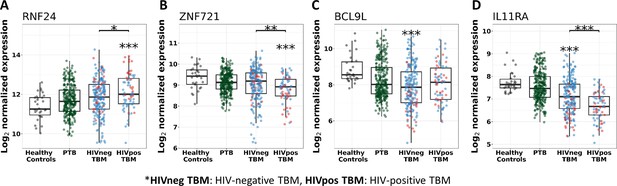
Gene expression of representative hub genes in healthy controls (n=30), PTB (n=295), HIV-negative TBM (n=207), and HIV-positive TBM (n=74).
Each dot represents gene expression from one participant. (A, B) expression of RNF24 and ZNF721 hub genes from the specific blue module. (C, D) expression of BCL9L and IL11RA hub genes from the specific yellow modules. The box presents median, 25th to 75th percentile and the whiskers present the minimum to the maximum points in the data. Comparisons were made between death (red) with survival (blue) or between HIV-negative and HIV-positive TBM by Wilcoxon rank sum test with p-values displayed as significance level above the boxes and the horizontal bars, respectively (*<0.05, **<0.01, ***<0.001).
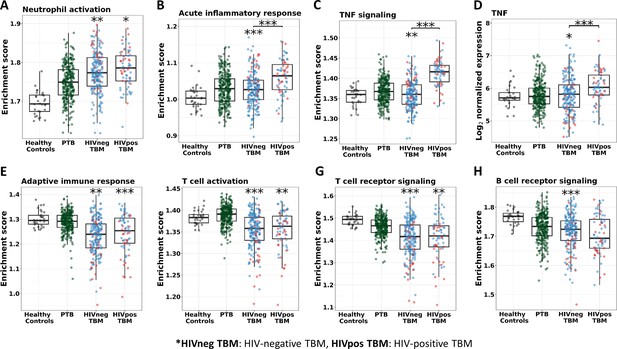
Enrichment score of immunity pathways in healthy controls (n=30), PTB (n=295), HIV-negative TBM (n=207), and HIV-positive TBM (n=74).
Pathway enrichment scores were calculated using single sample GSEA algorithm (Barbie et al., 2009). Each dot represents one participant. (A–C) showed box-plots depicting enrichment scores of the innate immunity pathways from the blue module. (E–H) enrichment scores of the adaptive immunity pathways from the red and brown modules and (D) normalized expression of TNF. The box presents median, 25th to 75th percentile and the whiskers present the minimum to the maximum points in the data. Comparisons were made between death (red) with survival (blue) or between HIV-negative and HIV-positive TBM by Wilcoxon rank sum test with p-values displayed as significance level above the boxes and the horizontal bars, respectively (*<0.05, **<0.01, ***<0.001).
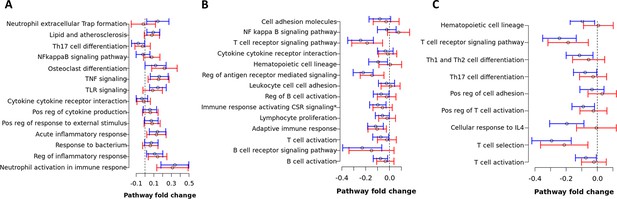
Pathway fold change enrichment to mortality of top hit pathways in blue, brown and red modules from the primary analysis.
Pathway fold change enrichment to mortality of each biological pathway defined as the distribution of the total of mean differential expression between death and survival TBM of all genes involved in the pathway based on Qusage method (Yaari et al., 2013). Median and 95% confidence interval of pathway fold change of each top-hit biological pathway in the blue (A), brown (B), and red module (C) in the primary analysis. Blue lines are from HIV-negative cohort and red lines from HIV-positive cohort. Pathway fold change enrichment above 0 indicates up-regulation while below 0 indicates down-regulation in death. Vertical dashed lines indicate no difference in death compared to survival TBM.
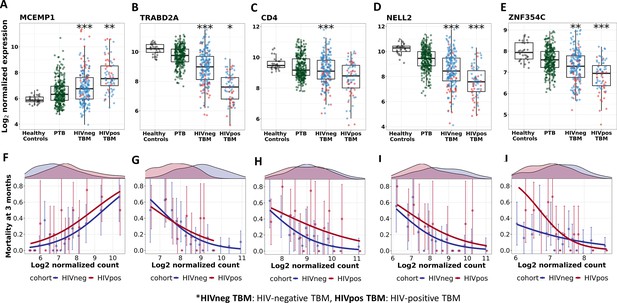
Gene expression and mortality of selected hub genes in TBM, PTB and healthy participants.
(A–E) Boxplots visualized distribution of gene expression of MCEMP1, TRABD2A, CD4, NELL2 and ZNF354C in healthy (n=30), PTB (n=295), TBM without HIV (n=207) and with HIV (n=74). Boxes indicate median and inter-quantile range. Dots indicate data in individuals. The comparisons were made between death (red) and survival (blue) by Wilcoxon rank sum test with p-value displayed as *<0.05, **<0.01 and ***<0.001. (F–J) Associations between mortality and gene expression of MCEMP1, TRABD2A, CD4, NELL2 and ZNF354C. In each figure, the upper panel corresponds to gene distribution in HIV-negative (blue) and HIV-positive (red), the lower panel presents the approximation of the association between that gene and mortality. Their gene expression were divided into 15 groups using equal-distant quantiles (1/15,…, 14/15) intervals and the proportion of mortality within those groups of patients were computed. Each point (error bar) presented the proportion with its confidence interval of mortality per group. The line represents the logistic curve that illustrates the mortality trend corresponding to a twofold increase in gene expression.
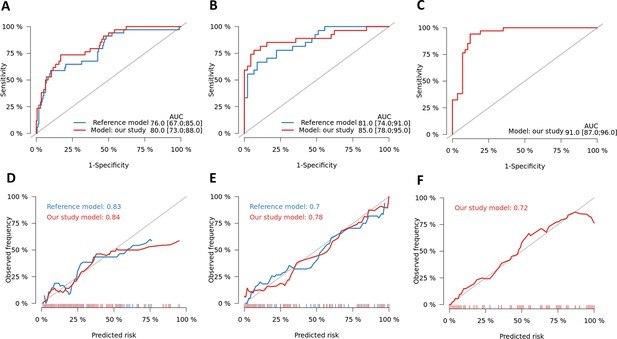
Performance of optimal gene set for TBM mortality prediction.
Receiver operating characteristic (ROC) curves for 3-month mortality for our developed model using expression level of four genes (MCEMP1, NELL2, CD4, and ZNF354C) with two clinical predictors (age, MRC grade) (red line) and the model develop by Thao et al. (blue line) for HIV-negative cohort (A), for HIV-positive cohort (B) and for HIV-negative qPCR validation cohort (C). The optimism corrected area under the curve (AUC) was calculated based on 1000 times bootstrap subsampling as described in the methods. Calibration plots were presented for the corresponding prediction models for HIV-negative (D), HIV-positive cohort (E) and HIV-negative qPCR validation cohort (E).
Tables
Baseline characteristics of TBM, PTB, and healthy controls.
Characteristics | RNA-seq cohorts | qPCR validation cohort | ||||||||||
---|---|---|---|---|---|---|---|---|---|---|---|---|
HIV-negative TBM n = 207 | HIV-positive TBM n = 74 | PTB n=295 | Healthy controls n = 30 | HIV-negative TBM n = 132 | ||||||||
n | Summary | n | Summary | n | Summary | n | Summary | n | Summary | |||
Age (years) | 207 | 46 (34, 58) | 74 | 34 (29, 40) | 295 | 44 (31, 52) | 30 | 33 (29, 37) | 132 | 48 (35, 60) | ||
Male sex | 207 | 127 (61) | 74 | 56 (76) | 295 | 228 (77) | 30 | 11 (37) | 132 | 84 (65) | ||
BMI (kg/m2) | 205 | 20.0 (18.2, 22.3) | 72 | 19.3 (17.2, 20.4) | 295 | 19.4 (17.7, 21.6) | 132 | 20.0 (18.2, 22.3) | ||||
Symptom duration (days) | 207 | 14 (11, 20) | 73 | 16 (10, 30) | 294 | 20 (10, 30) | 132 | 16 (13, 24) | ||||
History of TB treatment | 204 | 5 (2.5) | 74 | 15 (20) | 295 | 100 (34) | 130 | 12 (9.2) | ||||
Glasgow coma score | 207 | 14 (12, 15) | 72 | 14 (13, 15) | 132 | 14 (13, 15) | ||||||
Cavity chest X-ray | 270 | 129 (48) | ||||||||||
TB microbiological tests | ||||||||||||
MGIT culture positive | 199 | 50 (25) | 69 | 41 (59) | 295 | 279 (95) | ||||||
Xpert/Ultra positive | 198 | 42 (21) | 70 | 39 (56) | 295 | 287 (97) | ||||||
Microscopy positive | 205 | 48 (23) | 67 | 35 (52) | 203 | 169 (83) | 105 | 18 (17) | ||||
Blood (106 cells/ml) | ||||||||||||
Leucocyte count | 204 | 9.4 (7.0, 11.9) | 74 | 6.4 (5.0, 9.2) | 242 | 9.2 (7.4, 11.4) | 26 | 6.4 (5.6, 7.2) | 129 | 10.0 (7.7, 12.4) | ||
Neutrophil count | 204 | 7.1 (4.8, 9.1) | 74 | 5.0 (3.3, 6.9) | 241 | 6.1 (4.7, 8.2) | 26 | 3.4 (3.1, 4.1) | 129 | 7.8 (6.9, 8.5) | ||
Lymphocytes count | 204 | 1.2 (0.9, 2.0) | 74 | 0.7 (0.4, 1.2) | 242 | 1.9 (1.4, 2.3) | 26 | 2.2 (1.9, 2.6) | 129 | 1.2 (0.7, 1.8) | ||
CSF (103 cells/ml) | ||||||||||||
Leucocyte count | 207 | 142 (19, 323) | 73 | 124 (10, 453) | 106 | 122 (38, 328) | ||||||
Neutrophil count | 207 | 0 (0, 39) | 73 | 17 (0, 144) | 60 | 20 (3, 73) | ||||||
Lymphocyte count | 207 | 106 (18, 223) | 73 | 58 (10, 216) | 106 | 95 (79, 100) | ||||||
CD4 cell count (cells/mm3) | 71 | 67 (19, 124) | ||||||||||
Antiretroviral therapy | 70 | 28 (39%) | ||||||||||
HIV load (103 cells/ml) | 73 | 77.3 (0.8, 672) | ||||||||||
-
Values were displayed as median (1st and 3rd interquartile) for continuous variables and frequency (%) for categorical variables.
-
TBM = PTB = tuberculosis, andCSF = TBM = PTB = tuberculosis, and CSF = TBM = tuberculous meningitis, PTB = pulmonary tuberculosis, and CSF = cerebrospinal fluid.
Association between baseline clinical characteristics with TBM mortality in RNA-seq cohorts.
Characteristics | All TBM | Discovery cohort | Validation cohort | |||||||
---|---|---|---|---|---|---|---|---|---|---|
n | Survival n=220 | Death n=61 | HR* | 95% CI | p value* | Survival n=111 | Death n=31 | Survival n=109 | Death n=30 | |
Male sex | 281 | 144 (65) | 39 (64) | 0.92 | 0.55, 1.56 | 0.3 | 70 (63) | 19 (61) | 74 (68) | 20 (67) |
Age (years) | 281 | 41 (32, 53) | 39 (30, 60) | 1.01 | 0.99, 1.02 | 0.8 | 41 (32, 53) | 47 (33, 64) | 41 (32, 53) | 35 (29, 59) |
HIV infection | 281 | 47 (21) | 27 (44) | 2.34 | 1.41, 3.89 | <0.001 | 24 (22) | 14 (45) | 23 (21) | 13 (43) |
†Diagnostic category | 280 | |||||||||
definite TBM | 101 (46) | 32 (52) | 55 (50) | 17 (55) | 46 (42) | 15 (50) | ||||
possible TBM | 43 (20) | 6 (9.8) | 1.01 | 0.59, 1.72 | 0.9 | 20 (18) | 2 (6.5) | 23 (21) | 4 (13) | |
probable TBM | 75 (34) | 23 (38) | 0.50 | 0.21, 1.19 | 0.12 | 35 (32) | 12 (39) | 40 (37) | 11 (37) | |
‡MRC grade | 281 | |||||||||
grade 1 | 114 (52) | 7 (11) | 58 (52) | 3 (9.7) | 56 (51) | 4 (13) | ||||
grade 2 | 100 (45) | 35 (57) | 4.96 | 2.20, 11.2 | <0.001 | 51 (46) | 18 (58) | 49 (45) | 17 (57) | |
grade 3 | 6 (2.7) | 19 (31) | 26.4 | 11.0, 63.2 | <0.001 | 2 (1.8) | 10 (32) | 4 (3.7) | 9 (30) | |
Blood (106 cells/ml) | ||||||||||
Leukocyte count | 278 | 8.1 (6.2, 10.7) | 10.1 (6.7, 12.2) | 1.57 | 1.04, 2.38 | 0.033 | 7.8 (6.2, 10.6) | 8.6 (6.4, 12.0) | 8.4 (6.4, 10.7) | 10.8 (7.6, 12.4) |
Neutrophil count | 278 | 6.0 (4.0, 8.1) | 8.0 (5.0, 10.3) | 1.83 | 1.29, 2.59 | <0.001 | 5.9 (4.0, 8.1) | 6.7 (4.9, 9.6) | 6.4 (4.0, 8.2) | 9.0 (5.8, 10.3) |
Lymphocyte count | 278 | 1.2 (0.8, 1.9) | 0.9 (0.5, 1.2) | 0.70 | 0.56, 0.88 | 0.002 | 1.2 (0.7, 2.0) | 1.0 (0.6, 1.3) | 1.3 (0.9, 1.9) | 0.8 (0.5, 1.2) |
CSF (103 cells/ml) | ||||||||||
Leukocyte count | 280 | 129 (19, 340) | 148 (15, 360) | 0.99 | 0.91, 1.09 | 0.9 | 130 (17, 348) | 148 (15, 444) | 124 (27, 335) | 146 (25, 283) |
Neutrophil count | 117 | 78 (28, 192) | 135 (54, 402) | 1.23 | 1.01, 1.49 | 0.040 | 64 (30, 228) | 260 (163, 516) | 90 (29, 161) | 75 (50, 173) |
Lymphocyte count | 280 | 101 (18, 229) | 91 (14, 176) | 0.95 | 0.86, 1.05 | 0.3 | 104 (16, 208) | 123 (10, 214) | 97 (21, 248) | 86 (14, 147) |
-
Values were displayed as median (1st and 3rd interquartile) for continuous variables and frequency (%) for categorical variables.
-
TBM = CSF = TBM = tuberculous meningitis, CSF = cerebrospinal fluid.
-
*
Association of the corresponding variables with three-month mortality using a Cox regression model. Hazard ratio (HR) and p-value from the Cox regression model were presented in the table. For blood and CSF cells, the HR was calculated for each increase in log2 units.
-
†
Diagnostic categories were assigned according to the consensus case definition (Marais et al., 2010). Definite TBM refers to cases that are microbiologically confirmed TB by microscopy, culture and Xpert using CSF samples.
-
‡
MRC grade denotes modified British Medical Research Council criteria (Streptomycin in Tuberculosis Trials Commitee, Medical Research Council, 1948).
Validation of hub genes in PCR validation cohort.
No. | Genes | RNA-seq cohort HIV-negative TBM (n=207) | qPCR validation cohort HIV-negative TBM (n=132) | ||||
---|---|---|---|---|---|---|---|
HR | 95% CI | p value | HR | 95% CI | p value | ||
1 | MCEMP1 | 1.93 | 1.57, 2.37 | 4.07E-10 | 1.74 | 1.27, 2.37 | 4.99E-04 |
2 | FCAR | 2.37 | 1.70, 3.30 | 3.52E-07 | 0.59 | 0.40, 0.87 | 7.87E-03 |
3 | ETS2 | 2.65 | 1.85, 3.81 | 1.24E-07 | 0.27 | 0.16, 0.44 | 3.27E-07 |
4 | PGD | 2.65 | 1.84, 3.81 | 1.47E-07 | 0.18 | 0.09, 0.34 | 2.69E-07 |
5 | NELL2 | 0.56 | 0.45, 0.71 | 6.08E-07 | 0.80 | 0.66, 0.96 | 1.53E-02 |
6 | TRABD2A | 0.43 | 0.31, 0.58 | 4.94E-08 | 0.64 | 0.51, 0.8 | 1.05E-04 |
7 | TRAF5 | 0.31 | 0.2, 0.49 | 4.52E-07 | 0.47 | 0.36, 0.61 | 1.66E-08 |
8 | CD28 | 0.57 | 0.42, 0.76 | 1.42E-04 | 0.60 | 0.47, 0.76 | 2.83E-05 |
9 | TESPA1 | 0.36 | 0.25, 0.52 | 2.74E-08 | 0.65 | 0.48, 0.89 | 7.35E-03 |
10 | ABLIM1 | 0.31 | 0.2, 0.49 | 2.21E-07 | 0.47 | 0.36, 0.63 | 1.55E-07 |
11 | RASGRP1 | 0.45 | 0.31, 0.67 | 6.10E-05 | 0.59 | 0.45, 0.77 | 9.77E-05 |
-
Association of the hub genes with three-month mortality using a univariate Cox regression model. Hazard ratio (HR), 95% CI of HR and p-value from the Cox regression model were presented in the table. HR or 95% mean per increase 1 unit of log2 normalized expression of gene in RNA-seq cohort or decrease 1 unit of cycle threshold in qPCR validation cohort.
Comparison of gene signatures in distinguishing survival and death in TBM prognostic models.
RNA-seq cohorts | |||||||
---|---|---|---|---|---|---|---|
No. | Predictor set | All TBM n=281 | HIV-negative TBM n=207 | HIV-positive TBM n=74 | |||
AUC | Brier score | AUC | Brier score | AUC | Brier score | ||
1 | Reference model (Thao et al., 2018) | 0.78 | 0.14 | 0.77 | 0.12 | 0.82 | 0.18 |
2 | Gene set 1 (MCEMP1, TRABD2A, and CD4) | 0.78 | 0.14 | 0.78 | 0.11 | 0.65 | 0.23 |
3 | Gene set 2 (MCEMP1, NELL2 and ZNF354C) | 0.78 | 0.14 | 0.77 | 0.11 | 0.75 | 0.20 |
4 | Gene set 3 (MCEMP1, TRABD2A, CD4 and ZNF354C) | 0.78 | 0.14 | 0.77 | 0.11 | 0.73 | 0.21 |
5 | Gene set 4 (MCEMP1, NELL2, CD4 and ZNF354C) | 0.79 | 0.14 | 0.77 | 0.11 | 0.75 | 0.20 |
6 | Gene set 3 and clinical risk factors | 0.82 | 0.13 | 0.80 | 0.11 | 0.84 | 0.15 |
7 | Gene set 4 and clinical risk factors | 0.82 | 0.13 | 0.80 | 0.11 | 0.86 | 0.14 |
qPCR validation cohort | |||||||
No. | Predictor set | HIV-negative TBM (n=132) | |||||
AUC | Brier score | ||||||
8* | MCEMP1, NELL2, ZNF354C and clinical predictors | 0.91 | 0.12 |
-
Clinical risk factors were age, MRC grade and CSF lymphocytes (Streptomycin in Tuberculosis Trials Commitee, Medical Research Council, 1948; Thao et al., 2018). The prediction models for three-month mortality were based on multivariable logistic regression models with top-hit genes and clinical risk factors. Area under the curve (AUC) and Brier score were corrected for optimism using internal bootstrap resampling over 1000 iterations to evaluate the model performance. The Brier score is an overall performance measure, calculated as the mean squared difference between the predicted probability and the actual outcome, with smaller values indicating superior model performance.
-
*
CD4 data is unavailable for the analysis.
Additional files
-
Supplementary file 1
Additional analysis and results.
- https://cdn.elifesciences.org/articles/92344/elife-92344-supp1-v1.xlsx
-
MDAR checklist
- https://cdn.elifesciences.org/articles/92344/elife-92344-mdarchecklist1-v1.docx