Genetic Chimerism: Marmosets contain multitudes
Marmosets almost always produce non-identical twins which, unusually, share much more than just a womb. The same circulatory system connects the siblings during pregnancy, allowing two genetically distinct individuals to freely exchange the hematopoietic stem cells that give rise to all blood and immune cells (Gengozian et al., 1969; Wislocki, 1939; Hill, 1932). As a result, a drop of blood from this tiny South American primate reveals a mixture of cells originating from each twin (Benirschike and Brownhill, 1962). This is known as sibling chimerism – from Chimera, the monster from Greek mythology that is a hybrid of a lion, a goat and other creatures.
This quirk of nature has been known for decades (Benirschke et al., 1962). Yet, while studies have detected genetic information from the other twin in certain organs, it has remained difficult to precisely test whether sibling chimerism is limited to blood-related cells or extends to other cell types (Sweeney et al., 2012; Ross et al., 2007). Answering this question would help to uncover how cellular exchange takes place between marmoset twins, while also allowing researchers to investigate how genetic variations affect cell function in vivo. However, this requires technology that has only become recently available, and which makes it possible to isolate and sequence the genetic material of individual cells in a single tissue. If different genomes are identified in a heart sample, for example, these newer approaches can now distinguish whether they originate from the muscle cells of the heart, the blood cells pumped through it, or the resident immune cells that protect it. Now, in eLife, Ricardo del Rosario, Steven McCarroll and colleagues at Harvard Medical School, the Broad Institute and MIT report having addressed this knowledge gap by applying single-cell sequencing to various marmoset organs (del Rosario et al., 2024).
Rather than focusing on DNA, the team took advantage of the thousands of genetic variants transcribed into RNAs and opted instead for single-nucleus RNA-sequencing. Being able to capture all the genes being transcribed in an individual cell did double duty. del Rosario et al. could identify which tissues contained cells from the other twin; and they could also examine the impact of these genetic differences on gene expression patterns and cell function.
To investigate the first question, the team tested for sibling chimerism in the blood, liver, kidney and brain – all tissues which contain varying amounts of cell types deriving from hematopoietic stem cells. This confirmed that chimerism is prevalent and widespread in marmosets, while also allowing del Rosario et al. to precisely identify which cells were from the sampled individual, and which were from its twin. In doing so, they demonstrated that sibling chimerism is limited to cells from the two lineages (myeloid and lymphoid) that hematopoietic stem cells give rise to. Across the organs studied, all the other cell types examined originated from the twin being sampled (Figure 1).
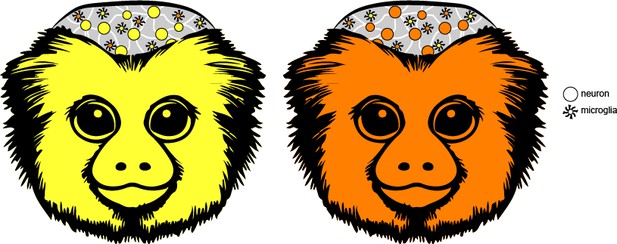
Investigating sibling chimerism in marmosets.
Marmosets almost always produce non-identical twins (also known as fraternal or dizygotic twins). However, these siblings are more alike than expected because they share a circulatory system in the womb, which allows them to exchange hematopoietic stem cells. As a result, the tissues and organs of the adult twins can contain cells carrying the genetic information of their sibling (orange cells in the yellow individual, and vice versa). Single-nucleus RNA sequencing approaches allowed del Rosario et al. to investigate which tissues contained cells from both twins, and in which proportion. This showed that only cells derived from hematopoietic stem cells display such ‘sibling chimerism’. In the brain, for example, the team found evidence for this phenomenon primarily in just one type of cell – immune cells known as microglia – with other cell types (such as neurons) being solely from the individual sampled.
The team then turned its attention to microglia, the primary immune cells of the brain, using a single-nucleus RNA-sequencing dataset of more than 2.2 million cells from various brain regions of 11 marmosets (Krienen et al., 2023). Consistent with the findings for non-brain tissues, this analysis first revealed that the proportion of sibling-derived microglia varies between the brain areas of an individual. del Rosario et al. suggest two possible explanations for this finding: (1) Such variations may be due to cell migration and proliferation taking place in a random manner, with the level of sibling-derived cells reflecting which cells arrived first and multiplied the most in a tissue. (2) Alternatively, cells from the twin may be recruited and proliferate differently across brain tissues and even local environments due to their genetic background.
To then test if genetic variations between cells could indeed impact their expression patterns, del Rosario et al. directly compared how microglia in the same brain region expressed their genes depending on whether they were from the sampled animal or its twin. When assessing if cells facing the same constraints respond differently because of variations in their genomes, this is as environmentally controlled a study can get in a living animal – nature’s very own common garden experiment, where scientists examine how populations of different genetic origins fare when facing the same conditions. The results show that the local environment, such as which brain region the cells were in, had a much larger impact in shaping gene expression than the genetic background. This does not indicate that genetic variation is unimportant, but it does highlight how cells – no matter who they come from – are very good at doing their job when they find themselves in the right place at the right time.
All told, this thoughtful and thorough study accomplishes two important goals. First, it all but closes a previously open question on the extent and cell origins of sibling chimerism. Second, it sets the stage for using this unique model system to examine, in a natural context, how genetic variation in microglia may impact brain development, function, and disease.
References
-
Further observations on marrow chimerism in marmosetsCytogenetics 1:245–257.https://doi.org/10.1159/000129734
-
The developmental history of the primatesPhilosophical Transactions of the Royal Society of London. Series B 221:45–178.https://doi.org/10.1098/rstb.1932.0002
-
Observations on twinning in marmosetsAmerican Journal of Anatomy 64:445–483.https://doi.org/10.1002/aja.1000640305
Article and author information
Author details
Publication history
- Version of Record published: April 25, 2024 (version 1)
Copyright
© 2024, Chiou and Snyder-Mackler
This article is distributed under the terms of the Creative Commons Attribution License, which permits unrestricted use and redistribution provided that the original author and source are credited.
Metrics
-
- 245
- views
-
- 18
- downloads
-
- 0
- citations
Views, downloads and citations are aggregated across all versions of this paper published by eLife.
Download links
Downloads (link to download the article as PDF)
Open citations (links to open the citations from this article in various online reference manager services)
Cite this article (links to download the citations from this article in formats compatible with various reference manager tools)
Further reading
-
- Genetics and Genomics
Uncovering the regulators of cellular aging will unravel the complexity of aging biology and identify potential therapeutic interventions to delay the onset and progress of chronic, aging-related diseases. In this work, we systematically compared genesets involved in regulating the lifespan of Saccharomyces cerevisiae (a powerful model organism to study the cellular aging of humans) and those with expression changes under rapamycin treatment. Among the functionally uncharacterized genes in the overlap set, YBR238C stood out as the only one downregulated by rapamycin and with an increased chronological and replicative lifespan upon deletion. We show that YBR238C and its paralog RMD9 oppositely affect mitochondria and aging. YBR238C deletion increases the cellular lifespan by enhancing mitochondrial function. Its overexpression accelerates cellular aging via mitochondrial dysfunction. We find that the phenotypic effect of YBR238C is largely explained by HAP4- and RMD9-dependent mechanisms. Furthermore, we find that genetic- or chemical-based induction of mitochondrial dysfunction increases TORC1 (Target of Rapamycin Complex 1) activity that, subsequently, accelerates cellular aging. Notably, TORC1 inhibition by rapamycin (or deletion of YBR238C) improves the shortened lifespan under these mitochondrial dysfunction conditions in yeast and human cells. The growth of mutant cells (a proxy of TORC1 activity) with enhanced mitochondrial function is sensitive to rapamycin whereas the growth of defective mitochondrial mutants is largely resistant to rapamycin compared to wild type. Our findings demonstrate a feedback loop between TORC1 and mitochondria (the TORC1–MItochondria–TORC1 (TOMITO) signaling process) that regulates cellular aging processes. Hereby, YBR238C is an effector of TORC1 modulating mitochondrial function.
-
- Genetics and Genomics
- Neuroscience
Adenine phosphoribosyltransferase (APRT) and hypoxanthine-guanine phosphoribosyltransferase (HGPRT) are two structurally related enzymes involved in purine recycling in humans. Inherited mutations that suppress HGPRT activity are associated with Lesch–Nyhan disease (LND), a rare X-linked metabolic and neurological disorder in children, characterized by hyperuricemia, dystonia, and compulsive self-injury. To date, no treatment is available for these neurological defects and no animal model recapitulates all symptoms of LND patients. Here, we studied LND-related mechanisms in the fruit fly. By combining enzymatic assays and phylogenetic analysis, we confirm that no HGPRT activity is expressed in Drosophila melanogaster, making the APRT homolog (Aprt) the only purine-recycling enzyme in this organism. Whereas APRT deficiency does not trigger neurological defects in humans, we observed that Drosophila Aprt mutants show both metabolic and neurobehavioral disturbances, including increased uric acid levels, locomotor impairments, sleep alterations, seizure-like behavior, reduced lifespan, and reduction of adenosine signaling and content. Locomotor defects could be rescued by Aprt re-expression in neurons and reproduced by knocking down Aprt selectively in the protocerebral anterior medial (PAM) dopaminergic neurons, the mushroom bodies, or glia subsets. Ingestion of allopurinol rescued uric acid levels in Aprt-deficient mutants but not neurological defects, as is the case in LND patients, while feeding adenosine or N6-methyladenosine (m6A) during development fully rescued the epileptic behavior. Intriguingly, pan-neuronal expression of an LND-associated mutant form of human HGPRT (I42T), but not the wild-type enzyme, resulted in early locomotor defects and seizure in flies, similar to Aprt deficiency. Overall, our results suggest that Drosophila could be used in different ways to better understand LND and seek a cure for this dramatic disease.