Unveiling the influence of tumor and immune signatures on immune checkpoint therapy in advanced lung cancer
Figures
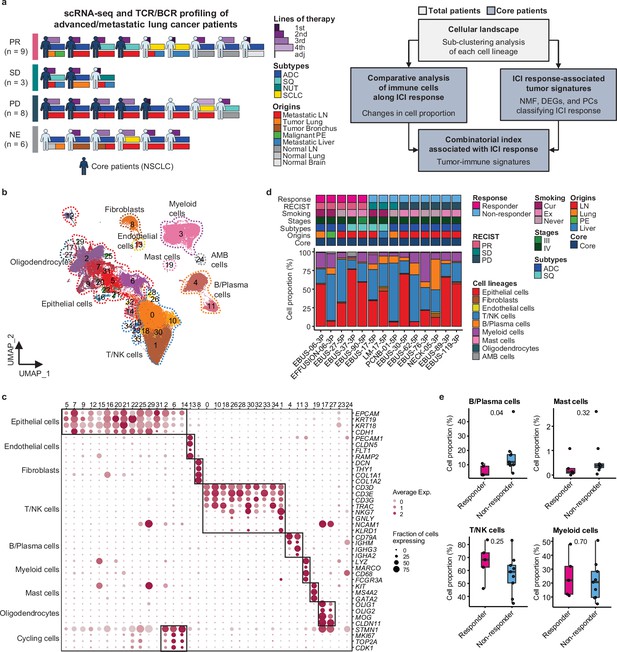
Cell lineage identification of 96,505 single cells from 26 patients with lung cancer treated with immune checkpoint inhibitor (ICI).
(a) Workflow of sample collection and single-cell analysis of lung cancer patients treated with ICI. (b) Uniform Manifold Approximation and Projection (UMAP) plot of 96,505 single cells from 33 samples acquired from 26 advanced lung cancer patients, colored by clusters. AMB cells, ambiguous cells. (c) Dot plot of mean expression of canonical marker genes for cell lineages. (d) Proportions of the cell lineages in non-small cell lung cancer (NSCLC) tissue from core patients shown by individual samples aligned with clinical data. Labels for origins indicate LN, metastatic lymph node; Lung, tumor lung; PE, malignant pleural effusion; Liver, metastatic liver. (e) Box plot of the percentage of cell lineages in responder and non-responder groups. Label represents p-value calculated via two-tailed Student’s t-test. Each box represents the median and the interquartile range (IQR, the range between the 25th and 75th percentile), whiskers indicate the 1.5 times of IQR.
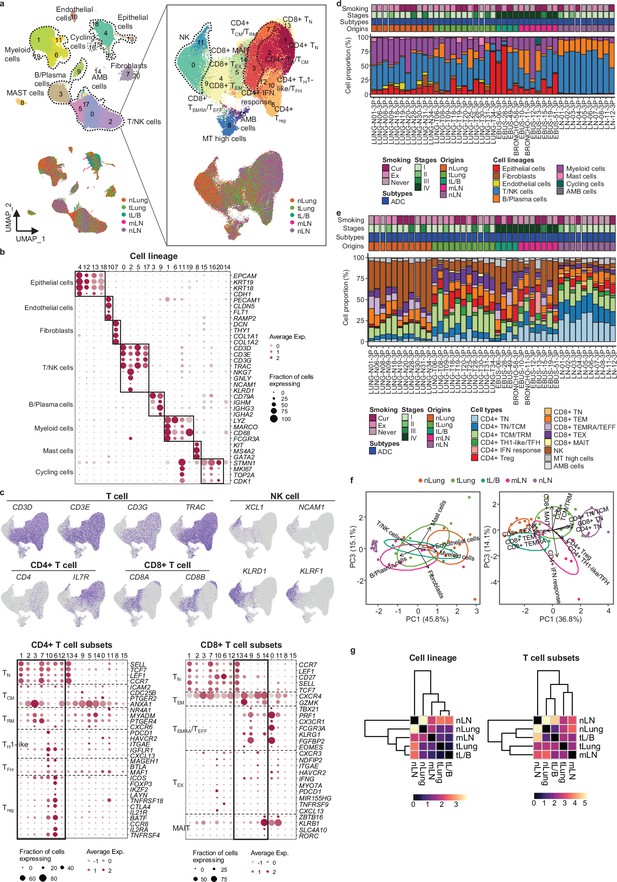
Immune cell profiles for sample collection sites in lung adenocarcinoma (LUAD).
(a) Uniform Manifold Approximation and Projection (UMAP) plot of 162,651 single cells (left) and 74,071T/NK cells (right) from 43 samples acquired from 33 LUAD patients, colored by clusters (top) and tissue origins (bottom). AMB cells, ambiguous cells. (b) Dot plot of mean expression of selected marker genes in each cell cluster of 162,651 cells from 43 samples acquired from 33 LUAD patients. (c) UMAP plot of T/NK cells, colored by expression of T, NK, CD4+ T, and CD8+ T cell marker genes (top). Dot plot of mean expression of selected CD4+ (bottom left) and CD8+ (bottom right) T cell marker genes in each cell cluster. (d, e) Proportions of the cell lineages (d) and T cell subsets (e) in LUAD tissue from 43 specimens shown by individual samples aligned with clinical data. Cur, current smoker; Ex, ex-smoker; Never, never smoker; ADC, adenocarcinoma; nLung, normal lung; tLung, early-stage tumor lung; tL/B, advanced-stage tumor lung; mLN, lymph node metastases; nLN, normal lymph node. (f) Biplot of principal component analysis (PCA) for cell lineage (left) and T/NK cell subset (right) proportions. (g) Euclidean distance map of centroids between groups of samples based on their tissue of origin in the PC1 and PC3 plot in f.
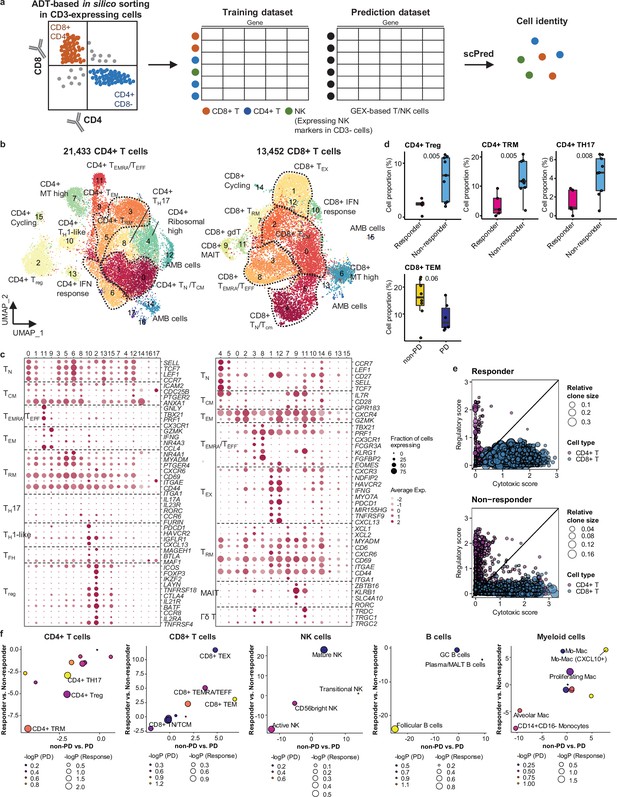
Classification and characterization of CD4+ and CD8+ T cell subtypes.
(a) Prediction strategy to classify CD4+, CD8+ T, and natural killer (NK) cells by applying antibody-derived tag (ADT) data from lung cancer. (b) Uniform Manifold Approximation and Projection (UMAP) plot of CD4+ and CD8+ T cells, colored by clusters. (c) Dot plot of mean expression of selected CD4+ (left) and CD8+ (right) T cell marker genes in each cell cluster. (d) Box plot of the percentage of CD4+ and CD8+ T cell types within total CD4+ plus CD8+ cells for sample groups representing responses to immune checkpoint inhibitor (ICI). Label represents p-value calculated via two-tailed Student’s t-test. Each box represents the median and the interquartile range (IQR), whiskers indicate the 1.5 times of IQR. (e) Association of T cell functional features with clonal expansion. Dot size depicts the relative clone size of each cell, which is divided by the total number of CD4+ and CD8+ T cells, respectively. Color indicates the cell lineage. (f) Comparisons of proportional changes in cell subtypes along ICI responses within each immune cell lineage. The quantitative values shown on the axis represent the mean difference in % cell proportions between sample groups. Dot size and color represent –log (p-value) for responder vs. non-responder and non-progressive disease (PD) vs. PD, respectively. The lower left quadrant shows cell types overrepresented in the poor responder groups, while the upper right quadrant indicates cell types overrepresented in the better responder groups. p-Value, two-tailed Student’s t-test.
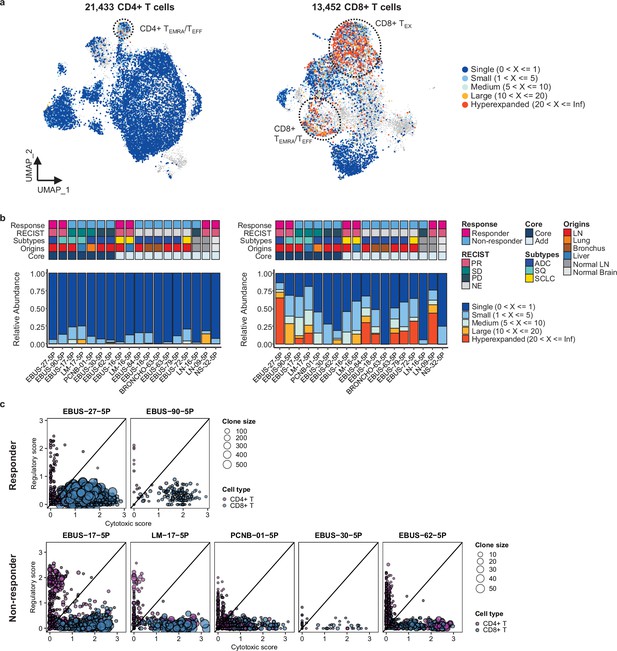
Features of T cell receptor (TCR) repertoires of CD4+ and CD8+ T cells.
(a) Uniform Manifold Approximation and Projection (UMAP) plot of CD4+ and CD8+ T cells, colored by clone types (single to hyperexpanded). (b) Proportions of clone types of CD4+ (left) and CD8+ (right) T cells in individual samples aligned with clinical data. (c) Association of T cell functional features with clonal expansion in individual samples. Dot size depicts the clone size of each cell and color indicates the cell lineage.
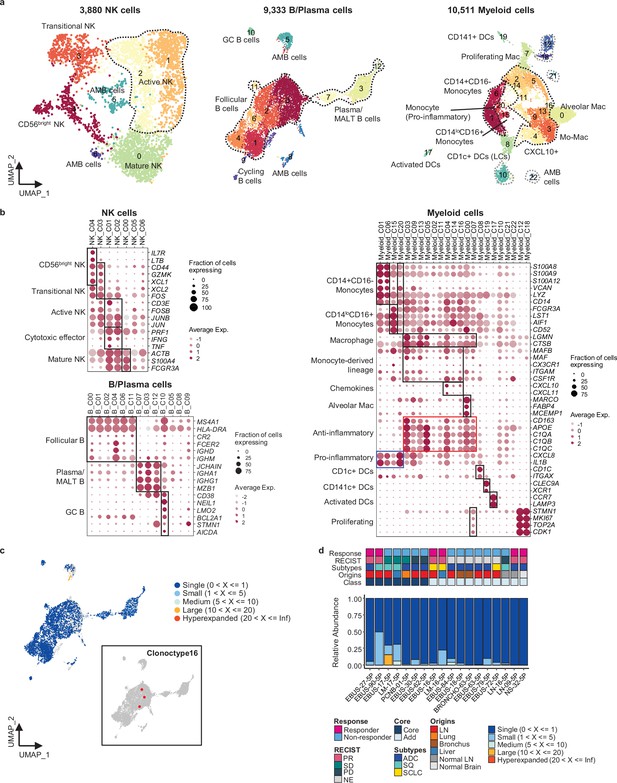
Heterogeneity in natural killer (NK), B, and myeloid cells and features of B cell receptor (BCR) repertoires.
(a) Uniform Manifold Approximation and Projection (UMAP) plot of NK, B, and myeloid cells, colored by clusters. (b) Dot plot of mean expression of selected marker genes in each cell cluster of NK, B, and myeloid cells. (c) UMAP plot of B cells, colored by clone types (single to hyperexpanded). Clone distribution of clonotype 16 in B cells (inset). (d) Proportions of clone types of B cells in individual samples aligned with clinical data.

Systemic evaluation of immune cell dynamics associated with response to immune checkpoint inhibitor (ICI).
(a) Heat map with unsupervised hierarchical clustering (left) and depicting significance (right) of proportional changes in cell subtypes within total immune cells. Proportional changes were compared for multiple ICI response groups. Color represents the –log (p-value) determined using two-tailed Student’s t-test. (b) Distribution map for each cell type across individual samples aligned with clinical data. Color represents Ro/e score calculated using the chi-square test.
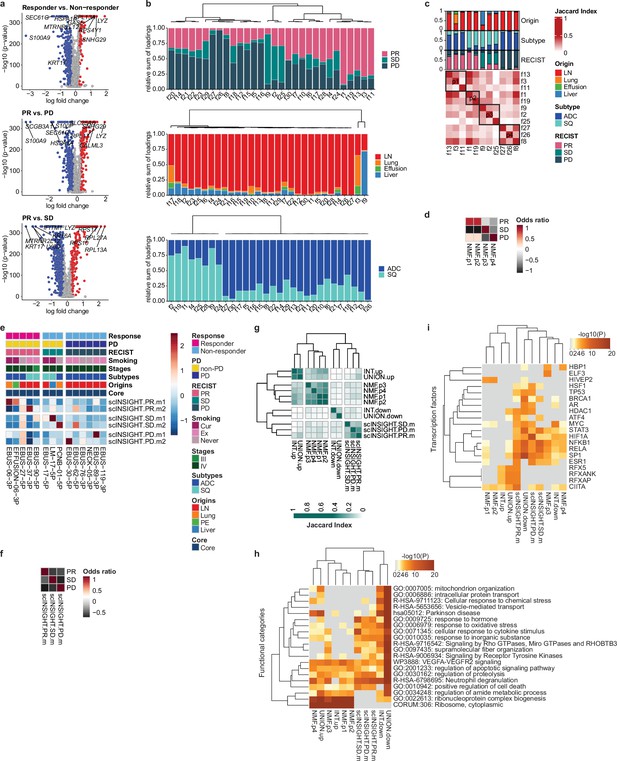
Single-cell tumor signatures associated with response to immune checkpoint inhibitor (ICI).
(a) Volcano plot of expression difference for responder vs. non-responder, partial response (PR) vs. progressive disease (PD), and PR vs. stable disease (SD) in 12,975 malignant cells from 11 core patients. The log fold change indicates the difference in the mean expression level for each gene. The significance level was determined using two-tailed Wilcoxon rank sum test. (b) Relative sum of loadings for all non-negative matrix factorization (NMF) factors contributed to malignant cells from 11 core patients across Response Evaluation Criteria in Solid Tumor (RECIST), tissue origins, and cancer subtypes, respectively. (c) Selection of RECIST-enriched NMF programs. (d) Enrichment of NMF programs for RECIST groups. Color represents the z-transformed odds ratio. (e) Expression map of RECIST-specific scINSIGHT modules across individual samples aligned with clinical data. Color represents the z-transformed mean expression of genes contributing to each module. (f) Enrichment of RECIST-specific gene modules for RECIST groups. Color represents the z-transformed odds ratio. (g) Hierarchical clustering of pairwise similarities between tumor signatures. INT and UNION, intersection and union of differentially expressed genes (DEGs) for responder vs. non-responder, PR vs. PD, and PR vs. SD in (a). (h) Functional categories and (i) transcription factors of the selected tumor signatures, analyzed by Metascape.
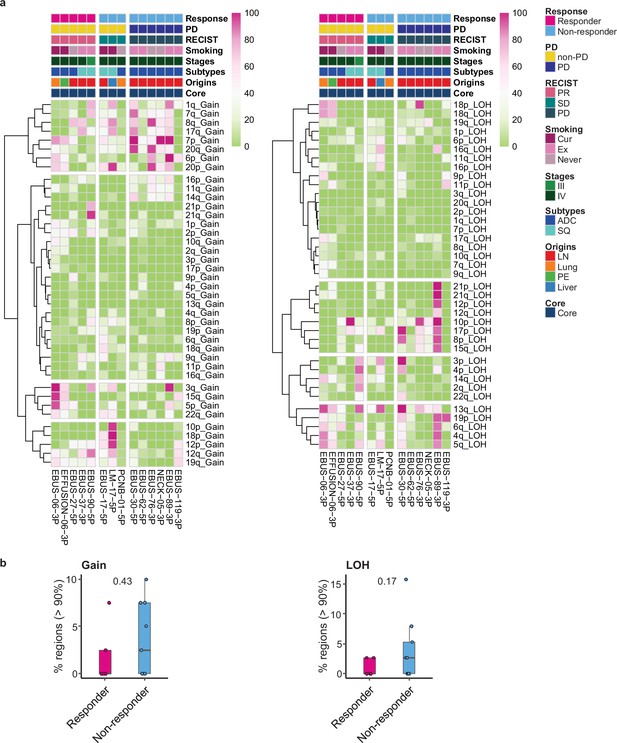
Copy number alteration (CNA) profiles in non-small cell lung cancer (NSCLC) tissue aligned with immune checkpoint inhibitor (ICI) response.
(a) Percent map of single-cell CNA events in individual samples aligned with clinical data. The copy number variation (CNV) signals of epithelial cells inferred from single-cell RNA sequencing (scRNA-seq) were calculated using inferCNV R package. Color represents the percentage of the gain (left) and loss of heterozygosity (LOH) (right) events in individual cells from each sample. (b) Comparison of % regions showing high perturbation (>90%) of CNA events between responder and non-responder. Label represents p-value calculated via two-tailed Student’s t-test. Each box represents the median and the interquartile range (IQR), whiskers indicate the 1.5 times of IQR.
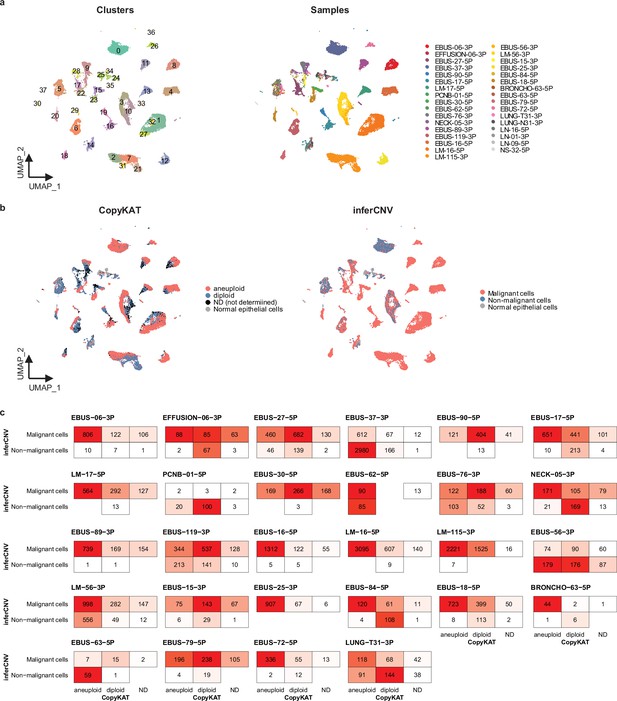
Classification of malignant cells based on genomic perturbations.
(a) Uniform Manifold Approximation and Projection (UMAP) plot of 31,519 epithelial cells from 26 patients, projected without batch correction, colored by clusters (left) and samples (right). (b) UMAP projection as shown in a, colored by malignant cell classification predicted using CopyKAT (left) and inferCNV (right) R packages. (c) Comparisons of malignant cell classification between CopyKAT and inferCNV in each individual sample.

Functional categories of single-cell differentially expressed gene (DEG) signatures associated with response to immune checkpoint inhibitor (ICI).
Enrichment map of significant Gene Ontology (GO) terms for each single-cell DEG signature associated with response to ICI. Color and size indicate the adjusted p-value (padj) and the normalized enrichment score (NES) calculated using fgsea R package, respectively.
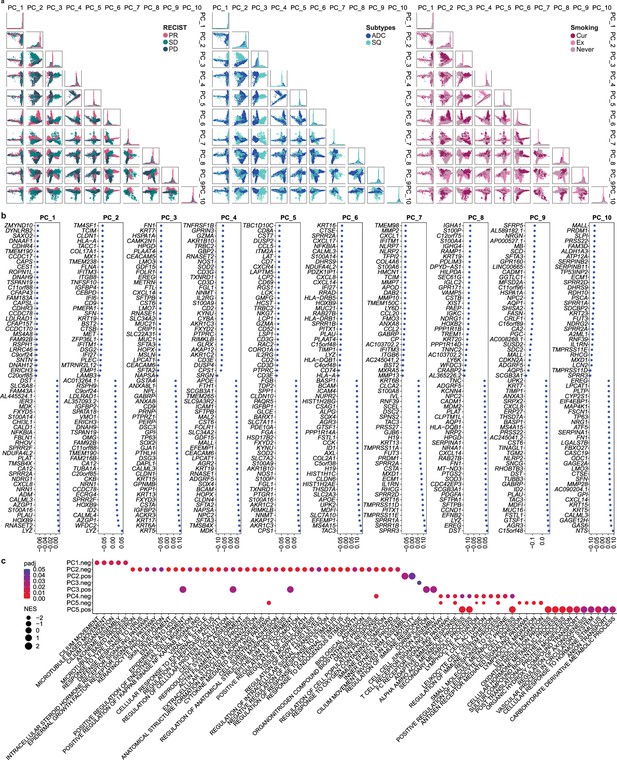
Selection and characteristics of principal component (PC) signatures in malignant cells.
(a) Unsupervised principal component analysis (PCA) projections of 12,975 malignant cells from PC1 to PC10, colored by Response Evaluation Criteria in Solid Tumor (RECIST), cancer subtypes, and smoking status. (b) Dot plot of top 60 (30 genes with + and – scores) genes that contributed to each PC. (c) Enrichment map of significant Gene Ontology (GO) terms for each PC signature. Color and size indicate the adjusted p-value (padj) and the normalized enrichment score (NES) calculated using fgsea R package, respectively.
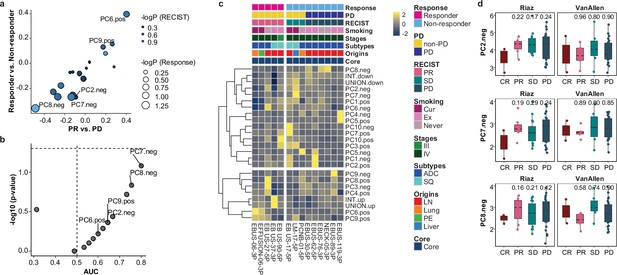
Immune checkpoint inhibitor (ICI) response association of principal component (PC) signatures in malignant cells.
(a) Comparison of mean expression difference for each PC signature along ICI responses. Dot size and color represent –log (p-value) for responder vs. non-responder and partial response (PR) vs. progressive disease (PD), respectively. p-Value, two-tailed Student’s t-test. (b) Receiver operating characteristic (ROC) analysis of PC signatures to classify responder and non-responder. p-Value, two-tailed Wilcoxon rank sum test. (c) Heat map of relative expression of PC signatures across individual samples aligned with clinical data. Mean expression of each PC signature in each sample is scaled by z-transform and visualized in the range from –2.5 to 2.5. INT and UNION, intersection and union of differentially expressed genes (DEGs) for responder vs. non-responder, PR vs. PD, and PR vs. stable disease (SD) in Figure 4a. (d) Box plot of mean expression of PC2.neg, PC7.neg, and PC9.neg genes in Riaz et al.’s and Van Allen al.’s melanoma ICI cohorts. Label represents p-value for comparisons of each group to complete response (CR), calculated using two-tailed Student’s t-test. Each box represents the median and the IQR, and whiskers indicate the 1.5 times of IQR.

Combination of tumor signatures and immune index classifying the response to immune checkpoint inhibitor (ICI).
(a) Heat map of relative contribution of tumor signatures and immune index across individual samples aligned with clinical data. Mean expression of each tumor signature and the percentage of each immune index are divided by the maximum value across samples. INT and UNION, intersection and union of differentially expressed genes (DEGs) for responder vs. non-responder, partial response (PR) vs. progressive disease (PD), and PR vs. stable disease (SD) in Figure 4a. (b) Univariate regression analysis of immune and tumor signatures for ICI response, together with clinical variables. (c) Receiver operating characteristic (ROC) analysis of combinatorial index to classify responder and non-responder. p-Value, two-tailed Wilcoxon rank sum test. p-Values adjusted with the Benjamini-Hochberg correction; UNION.down+B/plasma, 0.22; INT.down+B/plasma, 0.22; UNION.down+CD4+ regulatory T cell (Treg), 0.26; INT.down+UNION.down, 0.26; INT.down, 0.26; UNION.down, 0.26.
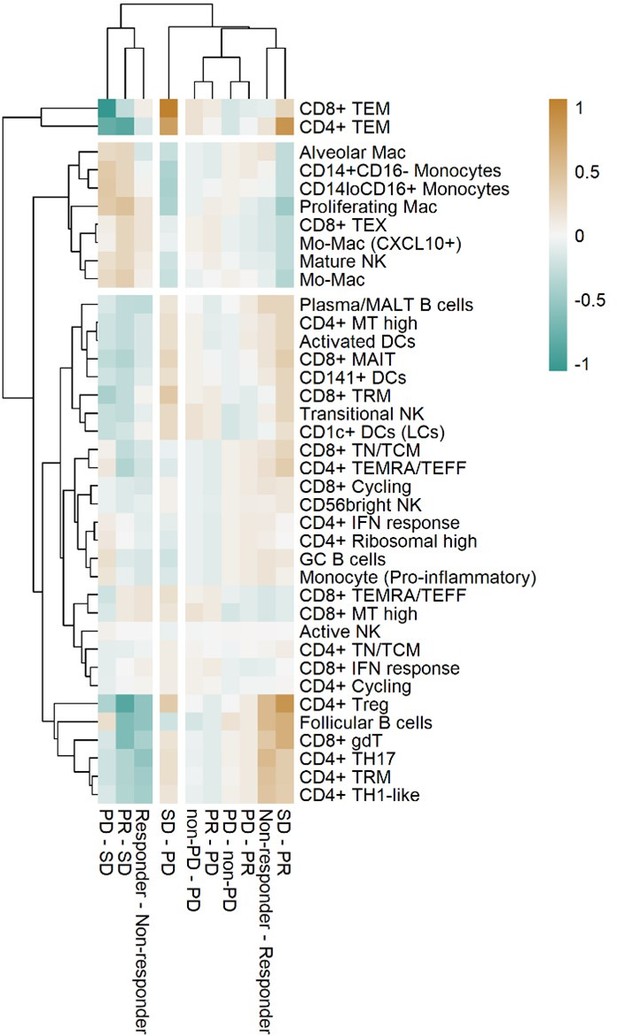
Heat map with unsupervised hierarchical clustering of proportional changes in cell subtypes within total immune cells.
Proportional changes were compared across multiple ICI response groups. The color represents the adjusted -log (p-value) calculated using the False Discovery Rate.
Tables
Clinical overview of non-small cell lung cancer (NSCLC) patients treated with immune checkpoint inhibitor (ICI).
Immunotherapy | Targets | No. of samples | No. of patients |
---|---|---|---|
Pembrolizumab | PD-1 | 9 | 8 |
Atezolizumab | PD-L1 | 1 | 1 |
Nivolumab | PD-1 | 2 | 1 |
Vibostolimab+Pembrolizumab | TIGIT+PD-1 | 2 | 1 |
RECIST | Description | No. of samples | No. of patients |
PR | Partial response | 5 | 4 |
SD | Stable disease | 3 | 2 |
PD | Progressive disease | 6 | 5 |
Multivariate overall survival analysis of tumor signature genes.
TCGA LUAD | TCGA LUSC | |||||||
---|---|---|---|---|---|---|---|---|
HR | Lower CI | Upper CI | p-Value | HR | Lower CI | Upper CI | p-Value | |
NMF.p1 | 0.89 | 0.53 | 1.48 | 0.64 | 0.92 | 0.66 | 1.27 | 0.60 |
NMF.p2 | 0.87 | 0.53 | 1.43 | 0.59 | 0.93 | 0.67 | 1.28 | 0.66 |
NMF.p3 | 0.76 | 0.49 | 1.21 | 0.25 | 1.06 | 0.76 | 1.49 | 0.72 |
NMF.p4 | 1.00 | 0.64 | 1.55 | 0.98 | 1.00 | 0.70 | 1.44 | 0.98 |
scINSIGHT.PR.m | 0.97 | 0.63 | 1.51 | 0.90 | 0.87 | 0.62 | 1.23 | 0.44 |
scINSIGHT.SD.m | 0.81 | 0.53 | 1.23 | 0.32 | 0.94 | 0.67 | 1.33 | 0.74 |
scINSIGHT.PD.m | 0.89 | 0.57 | 1.40 | 0.62 | 0.87 | 0.62 | 1.24 | 0.45 |
PC1.pos | 0.48 | 0.27 | 0.84 | 0.01 | 0.78 | 0.55 | 1.12 | 0.17 |
PC1.neg | 0.98 | 0.65 | 1.48 | 0.92 | 0.72 | 0.49 | 1.06 | 0.10 |
PC2.pos | 0.87 | 0.55 | 1.36 | 0.53 | 0.98 | 0.69 | 1.40 | 0.91 |
PC2.neg | 0.82 | 0.53 | 1.25 | 0.35 | 0.90 | 0.63 | 1.27 | 0.54 |
PC3.pos | 1.14 | 0.68 | 1.90 | 0.63 | 0.87 | 0.63 | 1.19 | 0.38 |
PC3.neg | 0.86 | 0.55 | 1.35 | 0.52 | 0.79 | 0.54 | 1.16 | 0.23 |
PC4.pos | 0.88 | 0.54 | 1.42 | 0.59 | 0.66 | 0.46 | 0.95 | 0.02 |
PC4.neg | 1.10 | 0.75 | 1.62 | 0.62 | 0.97 | 0.69 | 1.35 | 0.84 |
PC5.pos | 1.09 | 0.71 | 1.68 | 0.68 | 0.76 | 0.53 | 1.10 | 0.15 |
PC5.neg | 1.01 | 0.69 | 1.50 | 0.95 | 1.03 | 0.71 | 1.49 | 0.88 |
PC6.pos | 0.77 | 0.45 | 1.29 | 0.32 | 1.09 | 0.79 | 1.51 | 0.60 |
PC6.neg | 0.68 | 0.41 | 1.13 | 0.13 | 0.81 | 0.58 | 1.13 | 0.21 |
PC7.pos | 1.10 | 0.71 | 1.72 | 0.67 | 0.75 | 0.51 | 1.10 | 0.14 |
PC7.neg | 0.88 | 0.58 | 1.33 | 0.53 | 0.77 | 0.55 | 1.09 | 0.14 |
PC8.pos | 0.77 | 0.50 | 1.18 | 0.23 | 1.01 | 0.72 | 1.43 | 0.94 |
PC8.neg | 1.00 | 0.65 | 1.52 | 0.99 | 0.95 | 0.66 | 1.37 | 0.80 |
PC9.pos | 0.91 | 0.60 | 1.38 | 0.66 | 1.02 | 0.73 | 1.43 | 0.91 |
PC9.neg | 1.11 | 0.73 | 1.68 | 0.63 | 1.02 | 0.72 | 1.45 | 0.89 |
PC10.pos | 1.13 | 0.76 | 1.68 | 0.55 | 1.28 | 0.88 | 1.86 | 0.20 |
PC10.neg | 0.93 | 0.59 | 1.46 | 0.75 | 0.93 | 0.65 | 1.35 | 0.71 |
INT.up | 0.82 | 0.53 | 1.26 | 0.37 | 1.00 | 0.71 | 1.42 | 0.99 |
UNION.up | 0.80 | 0.52 | 1.21 | 0.28 | 0.77 | 0.53 | 1.13 | 0.18 |
INT.down | 0.84 | 0.56 | 1.24 | 0.37 | 1.07 | 0.73 | 1.57 | 0.74 |
UNION.down | 0.67 | 0.42 | 1.06 | 0.08 | 0.75 | 0.52 | 1.07 | 0.11 |
P-values from univariate and multivariate regression analysis of immune and tumor signatures for ICI response.
Univariate | Multivariate | ||
---|---|---|---|
p-value < | INT.down | 0.009 | 0.633 |
0.05 in | UNION.down | 0.009 | 0.346 |
univariate | CD4+ TH17 | 0.034 | 0.429 |
analysis | PC7.neg | 0.047 | 0.360 |
CD4+ Treg | 0.048 | 0.710 | |
p-value < 0.05 in multivariate analysis | Active NK | 0.973 | 0.004 |
Additional files
-
Supplementary file 1
Patient information of lung cancer immune checkpoint inhibitor (ICI) cohorts.
- https://cdn.elifesciences.org/articles/98366/elife-98366-supp1-v1.xlsx
-
Supplementary file 2
List of genes specific to the cell clusters in total cells and each cell lineage.
- https://cdn.elifesciences.org/articles/98366/elife-98366-supp2-v1.xlsx
-
Supplementary file 3
List of differentially expressed genes (DEGs) in comparison between immune checkpoint inhibitor (ICI) response groups.
- https://cdn.elifesciences.org/articles/98366/elife-98366-supp3-v1.xlsx
-
Supplementary file 4
Details of Gene Ontology (GO) terms significantly enriched in comparisons for immune checkpoint inhibitor (ICI) response groups and principal components (PCs).
- https://cdn.elifesciences.org/articles/98366/elife-98366-supp4-v1.xlsx
-
Supplementary file 5
Gene list of tumor signatures.
- https://cdn.elifesciences.org/articles/98366/elife-98366-supp5-v1.xlsx
-
MDAR checklist
- https://cdn.elifesciences.org/articles/98366/elife-98366-mdarchecklist1-v1.docx