RNA Biology: Structures to the people!
The structures of molecules often hold the key to understanding their roles in cells. Thus, when Watson and Crick proposed the double-helix structure for DNA, they immediately speculated on how DNA may replicate. Unfortunately, working out the structures of RNA molecules is challenging, and the techniques needed to do so—X-ray crystallography and NMR—are only available at a small number of locations worldwide. This has prevented many small laboratories from embracing structural work.
Instead, some researchers have started to predict the three-dimensional structures of RNA molecules based on the sequence of nucleotides in the molecule, and there is even an ‘RNA Puzzles’ competition to compare the performance of prediction algorithms (Cruz et al., 2012; Miao et al., 2015). Moreover, the discovery of large numbers of non-coding RNAs in recent years has substantially increased the need for methods that can determine the structure of RNA molecules quickly and reliably. Now, in eLife, Rhiju Das and co-workers—including Clarence Yu Cheng as first author—describe a new approach that promises to simplify the determination of RNA structures to nanometer resolution (Cheng et al., 2015).
This approach, which is called multidimensional chemical mapping, can be likened to the power of social networking. In social networks, centralized ‘hot spots’ of communication can be identified, based on how well connected they are, and in some cases these hot spots can influence, or constrain, the activity of large numbers of other individuals in the network. Similarly, the approach developed by Cheng et al., who are based at Stanford University, identifies the three-dimensional networks of interactions between nucleotides within a given RNA molecule to guide the predictions of its structure.
Interactions between nucleotides in most RNA molecules lead to secondary structures called helices, which include loops, bulged nucleotides and multi-helical junctions. In turn, these secondary structure elements influence how the molecule folds into its final three-dimensional (or tertiary) structure. Previously, Das and others have combined protocols that predict the secondary structure of RNA with three-dimensional modelling algorithms (Kladwang et al., 2011; Cruz et al., 2012; Miao et al., 2015) to generate models of the tertiary structures of RNAs. However, the relative orientations of the secondary structure elements could not be precisely defined due to a lack of experimental data on the three-dimensional structure.
Several experimental approaches can fill this gap, but many require specialized reagents or equipment, which limits their use. Just as the growth of social media depended on developments in technological infrastructure, the current progress in multidimensional chemical mapping has been made possible by the widespread availability of instruments for deep sequencing DNA. In a wry twist of fate, sequencing has become a key tool for defining structure.
The idea to use sequencing to determine RNA structures is not new (see review by Ge and Zhang, 2015), but to date the focus has been on predicting secondary structure. Recently, however, Kevin Weeks and co-workers went a step further and used correlated data between nucleotides to predict tertiary structure, although they only reported on the interactions between two nucleotides, cytosine and adenosine (Homan et al., 2014). Cheng et al. go even further by considering potentially all of the interactions between all four of the nucleotides.
Multidimensional chemical mapping combines a technique called MOHCA (short for multiplexed •OH cleavage analysis) with deep sequencing and algorithms that predict the RNA secondary and tertiary structure (Figure 1). MOHCA uses RNA molecules that contain chemically modified nucleotides, usually one per molecule, at random positions. When the RNA molecules are treated with certain chemicals, highly reactive molecules called hydroxyl radicals are produced, and these cleave the RNA molecule near the site of the modified nucleotide. This effectively marks the positions of other nucleotides that are found near the modified nucleotide in the three-dimensional structure of the RNA molecule.
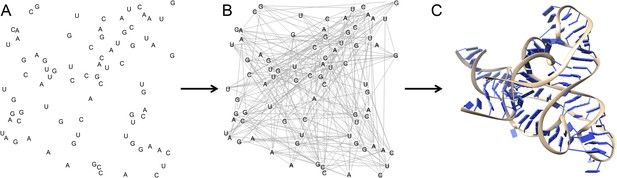
The three dimensional structure of an RNA molecule can be predicted by combining MOHCA, deep sequencing and algorithms that predict secondary and tertiary structures in the RNA.
(A) In MOHCA, copies of the RNA of interest that contain modified nucleotides—on average one per molecule—are made. These modified nucleotides produce hydroxyl groups that cleave the RNA and damage other nucleotides near to the modified nucleotide. A reverse transcriptase enzyme is used to generate DNA copies of the RNA molecules. This enzyme stops copying each RNA molecule at the point where it is cleaved or damaged. Therefore, sequencing these DNA fragments reveals the positions of nucleotides that are close to the modified nucleotide in the three-dimensional structure. This information is used to make a network of the interactions between all the nucleotides in the RNA (B). This network is then combined with algorithms that predict the secondary and tertiary structures to produce a single three-dimensional model of the tertiary structure (C). Image prepared by Nathan Baird using CodePen (codepen.io/blendmaster/full/uqibt) and structure 2YIE from the Protein Data Bank.
Previously, gel electrophoresis has been used to show where the RNA molecule is cleaved during MOHCA, but combining MOHCA with deep sequencing provides much more detailed information and significantly increases throughput. Cheng et al. constructed maps showing the interactions between the nucleotides in the molecule and combined these with algorithms that predict secondary and tertiary structures to generate a well-defined three-dimensional model of the RNA. To demonstrate the accuracy and utility of this new approach, Cheng et al. applied it to five RNAs of known structure, and to one RNA whose structure had not been released at the time. Multidimensional chemical mapping successfully predicted the three-dimensional structures of all six RNA molecules to within nanometer resolution.
However, RNA often interacts with cofactors, which remain largely undefined or otherwise make structure determination more challenging. Multidimensional chemical mapping works in solution and can help define structures in the absence of these cofactors. To test this, Cheng et al. used multidimensional chemical mapping to predict the structures of several RNAs without their cellular cofactors. Their prediction for the structure of the internal ribosomal entry site in human Hox messenger RNA will aid efforts to determine the structure of this mRNA in complex with the ribosome and other partner molecules using techniques such as cryo-electron microscopy. Cheng et al. also modeled ligand-free conformations of several riboswitches, which may guide the development of drugs that stabilize non-functional RNA conformations.
But what challenges are still ahead of us? Currently, the size of the RNA limits the analysis. Refining the modeling algorithm and the way the samples are prepared for sequencing may help to improve the predictions. A more general concern is the presence of naturally occurring modifications to RNA molecules inside cells. In highly modified structures—like transfer RNAs—these chemical groups influence the folding of the molecule. Thus, when solving the structure of an unmodified RNA molecule produced in an artificial system, an important piece in the puzzle is missing. Even when they are present, how these chemical modifications affect the cleavage of RNA by the hydroxyl radicals still needs to be assessed.
The field of structural biology is undergoing dramatic changes as improvements in technology—such as the development of free-electron lasers and improved detectors for electron microscopy—are making it possible to solve structures at atomic resolution, almost in a high-throughput manner. Will the combination of three-dimensional modeling and sequencing become a similar game changer in the field of RNA structures, being used and adapted by large numbers of researchers? Multidimensional chemical mapping clearly has the potential to transform structure determination by putting it into the hands of researchers who have experience in molecular biology and access to deep sequencing.
Today, this is almost everybody: structures to the people!
References
-
Single-molecule correlated chemical probing of RNAProceedings of the National Academy of Sciences of USA 111:13858–13863.https://doi.org/10.1073/pnas.1407306111
-
A two-dimensional mutate-and-map strategy for non-coding RNA structureNature Chemistry 3:954–962.https://doi.org/10.1038/nchem.1176
Article and author information
Author details
Publication history
Copyright
© 2015, Baird and Leidel
This article is distributed under the terms of the Creative Commons Attribution License, which permits unrestricted use and redistribution provided that the original author and source are credited.
Metrics
-
- 1,977
- views
-
- 172
- downloads
-
- 0
- citations
Views, downloads and citations are aggregated across all versions of this paper published by eLife.
Download links
Downloads (link to download the article as PDF)
Open citations (links to open the citations from this article in various online reference manager services)
Cite this article (links to download the citations from this article in formats compatible with various reference manager tools)
Further reading
-
- Biochemistry and Chemical Biology
The conformational ensemble and function of intrinsically disordered proteins (IDPs) are sensitive to their solution environment. The inherent malleability of disordered proteins, combined with the exposure of their residues, accounts for this sensitivity. One context in which IDPs play important roles that are concomitant with massive changes to the intracellular environment is during desiccation (extreme drying). The ability of organisms to survive desiccation has long been linked to the accumulation of high levels of cosolutes such as trehalose or sucrose as well as the enrichment of IDPs, such as late embryogenesis abundant (LEA) proteins or cytoplasmic abundant heat-soluble (CAHS) proteins. Despite knowing that IDPs play important roles and are co-enriched alongside endogenous, species-specific cosolutes during desiccation, little is known mechanistically about how IDP-cosolute interactions influence desiccation tolerance. Here, we test the notion that the protective function of desiccation-related IDPs is enhanced through conformational changes induced by endogenous cosolutes. We find that desiccation-related IDPs derived from four different organisms spanning two LEA protein families and the CAHS protein family synergize best with endogenous cosolutes during drying to promote desiccation protection. Yet the structural parameters of protective IDPs do not correlate with synergy for either CAHS or LEA proteins. We further demonstrate that for CAHS, but not LEA proteins, synergy is related to self-assembly and the formation of a gel. Our results suggest that functional synergy between IDPs and endogenous cosolutes is a convergent desiccation protection strategy seen among different IDP families and organisms, yet the mechanisms underlying this synergy differ between IDP families.
-
- Biochemistry and Chemical Biology
- Stem Cells and Regenerative Medicine
Human induced pluripotent stem cells (hiPSCs) have great potential to be used as alternatives to embryonic stem cells (hESCs) in regenerative medicine and disease modelling. In this study, we characterise the proteomes of multiple hiPSC and hESC lines derived from independent donors and find that while they express a near-identical set of proteins, they show consistent quantitative differences in the abundance of a subset of proteins. hiPSCs have increased total protein content, while maintaining a comparable cell cycle profile to hESCs, with increased abundance of cytoplasmic and mitochondrial proteins required to sustain high growth rates, including nutrient transporters and metabolic proteins. Prominent changes detected in proteins involved in mitochondrial metabolism correlated with enhanced mitochondrial potential, shown using high-resolution respirometry. hiPSCs also produced higher levels of secreted proteins, including growth factors and proteins involved in the inhibition of the immune system. The data indicate that reprogramming of fibroblasts to hiPSCs produces important differences in cytoplasmic and mitochondrial proteins compared to hESCs, with consequences affecting growth and metabolism. This study improves our understanding of the molecular differences between hiPSCs and hESCs, with implications for potential risks and benefits for their use in future disease modelling and therapeutic applications.